Polite Teacher: Semi-Supervised Instance Segmentation With Mutual Learning and Pseudo-Label Thresholding
IEEE ACCESS(2024)
摘要
We present Polite Teacher, a simple yet effective method for the task of semi-supervised instance segmentation. The proposed architecture relies on the Teacher-Student mutual learning framework. To filter out noisy pseudo-labels, we use confidence thresholding for bounding boxes and mask scoring for masks. The approach has been tested with CenterMask, a single-stage anchor-free detector. Tested on the COCO 2017 val dataset, our architecture significantly (approx. +8 pp. in mask AP) outperforms the baseline at different supervision regimes. To the best of our knowledge, this is one of the first works tackling the problem of semi-supervised instance segmentation and the first one devoted to an anchor-free detector. The code is available: github.com/AI-Clearing/PoliteTeacher.
更多查看译文
关键词
Instance segmentation,Detectors,Task analysis,Semantic segmentation,Computer architecture,Training,Feature extraction,Semisupervised learning,Education,Electronic learning,Semi-supervised instance segmentation,anchor-free detection,instance segmentation,semi-supervised learning
AI 理解论文
溯源树
样例
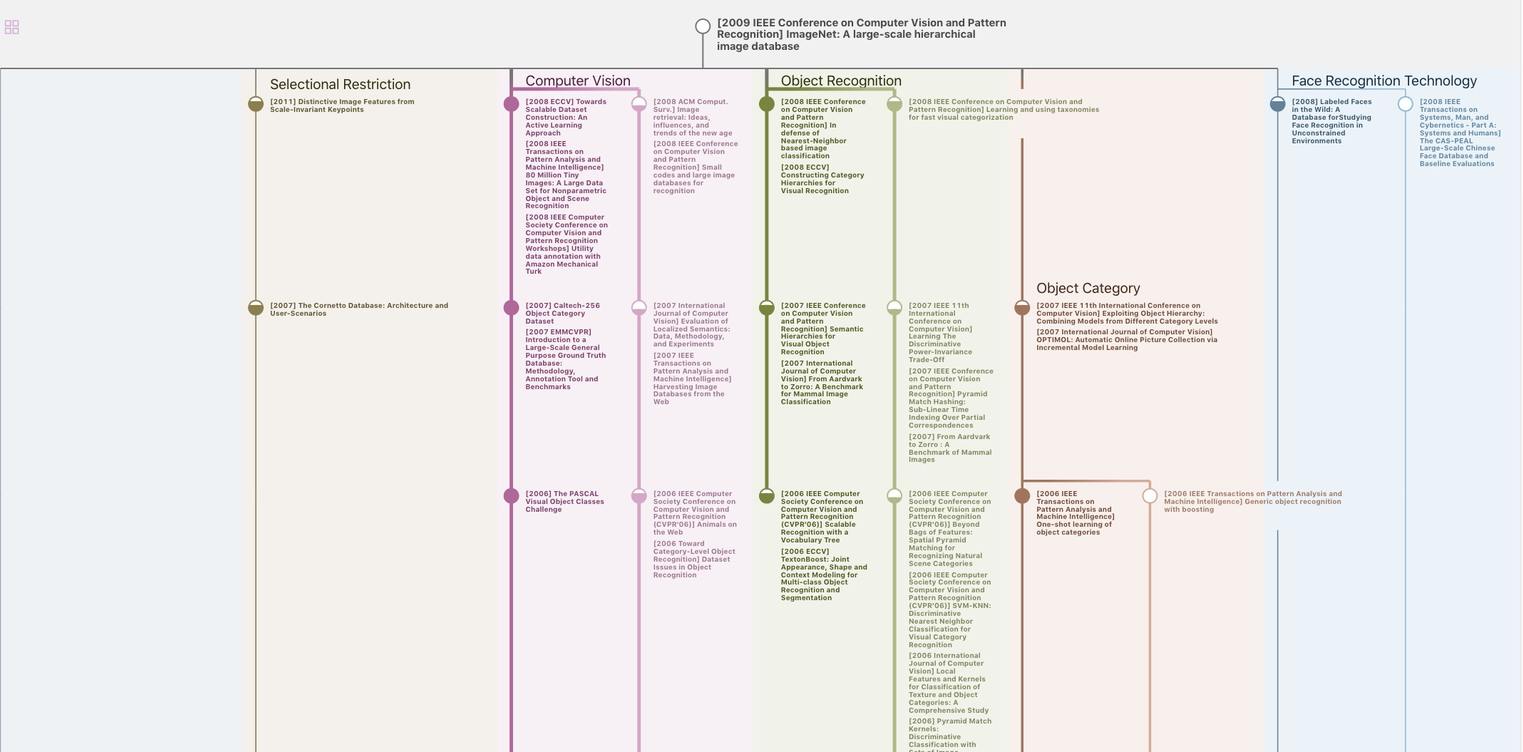
生成溯源树,研究论文发展脉络
Chat Paper
正在生成论文摘要