Unsupervised Audio-Visual Lecture Segmentation
WACV(2023)
摘要
Over the last decade, online lecture videos have become increasingly popular and have experienced a meteoric rise during the pandemic. However, video-language research has primarily focused on instructional videos or movies, and tools to help students navigate the growing online lectures are lacking. Our first contribution is to facilitate research in the educational domain by introducing AVLectures, a large-scale dataset consisting of 86 courses with over 2,350 lectures covering various STEM subjects. Each course contains video lectures, transcripts, OCR outputs for lecture frames, and optionally lecture notes, slides, assignments, and related educational content that can inspire a variety of tasks. Our second contribution is introducing video lecture segmentation that splits lectures into bite-sized topics. Lecture clip representations leverage visual, textual, and OCR cues and are trained on a pretext self-supervised task of matching the narration with the temporally aligned visual content. We formulate lecture segmentation as an unsupervised task and use these representations to generate segments using a temporally consistent 1-nearest neighbor algorithm, TW-FINCH [44]. We evaluate our method on 15 courses and compare it against various visual and textual baselines, outperforming all of them. Our comprehensive ablation studies also identify the key factors driving the success of our approach.
更多查看译文
关键词
segmentation,audio-visual
AI 理解论文
溯源树
样例
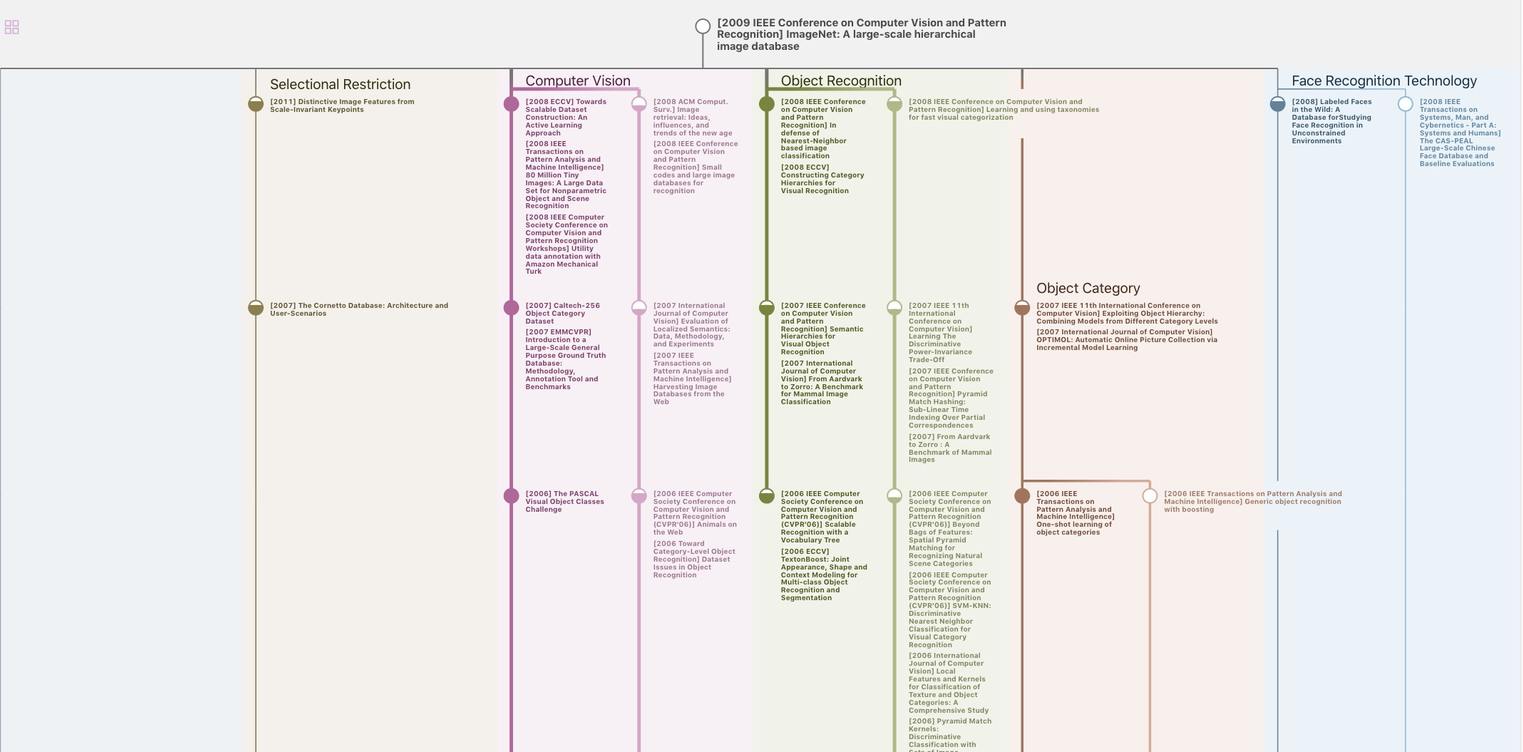
生成溯源树,研究论文发展脉络
Chat Paper
正在生成论文摘要