A Low-Complexity Transceiver Design for Terahertz Communication based on Deep Learning
2022 IEEE INTERNATIONAL CONFERENCE ON COMMUNICATIONS WORKSHOPS (ICC WORKSHOPS)(2022)
摘要
Terahertz communication is one of the important candidate technologies for 6G in the future. The deep learning (DL)-based transmission method can use the actual transmission data to learn and fit the channel and non-ideal characteristics of devices in real time, and thus is an effective manner to solve the modeling problems of the channel and device responses in the terahertz band. However, the DL-based method has a high complexity which prevents its usage in the high-rate terahertz transmission. This paper proposes a binary neural network based model to reduce complexity, combined with the training method based on generative adversarial network (GAN) to overcome the unknown channel problem. Simulation results show that the proposed method can achieve a similar performance with QAM under the AWGN channel, but performs much better when nonideal characteristics exist. Besides, the complexity of the proposed method is much less than the existing DL-based method, and the data size to be transmitted back in GAN is also largely reduced. All these results reflect the feasibility of the proposed method in scenarios with significant non-ideal characteristics such as the terahertz communication.
更多查看译文
关键词
Terahertz communication, deep learning, binary neural network, generative adversarial network
AI 理解论文
溯源树
样例
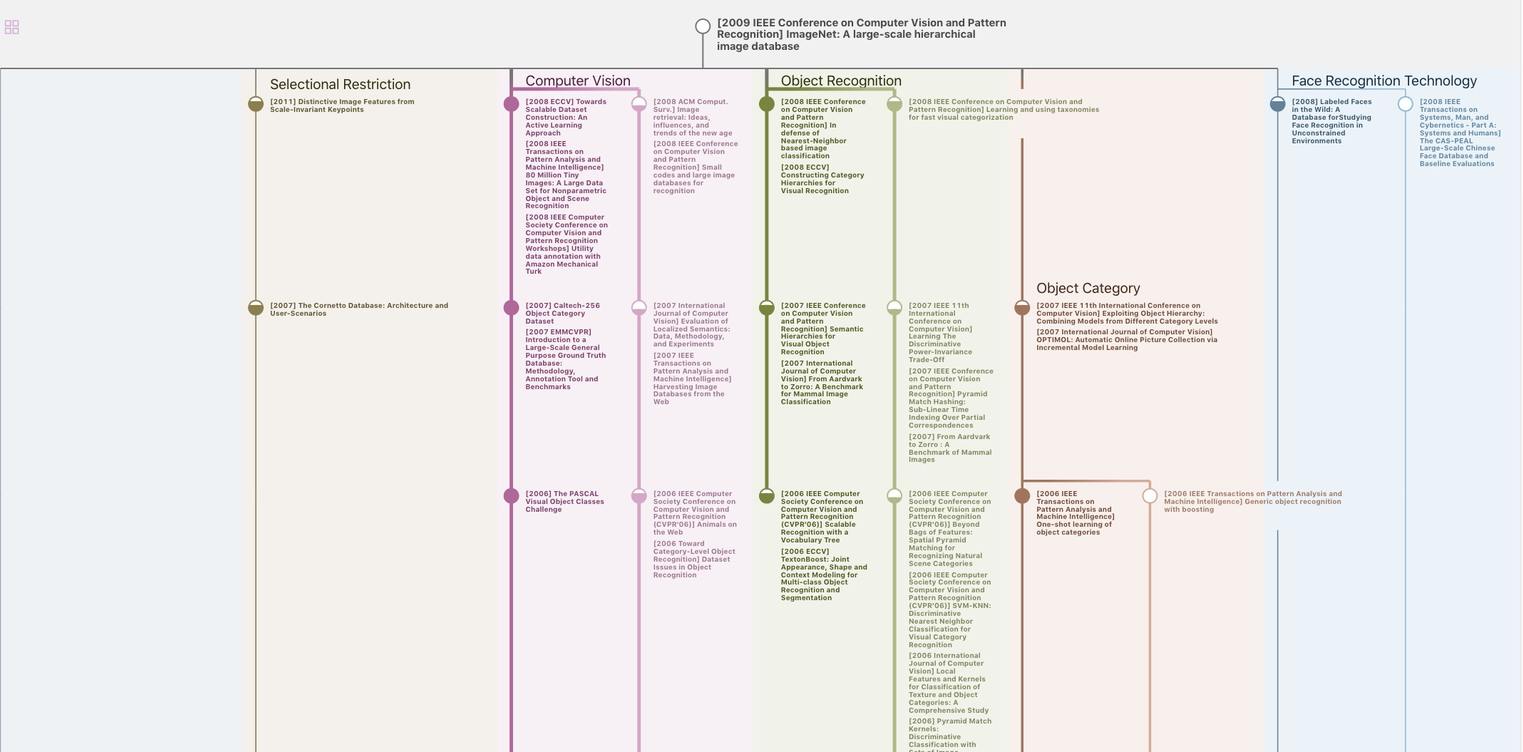
生成溯源树,研究论文发展脉络
Chat Paper
正在生成论文摘要