Towards Human-Machine Recognition Alignment: An Adversarilly Robust Multimodal Retrieval Hashing Framework
IEEE TRANSACTIONS ON COMPUTATIONAL SOCIAL SYSTEMS(2023)
摘要
The multimodality nature of web data has necessitated complex multimodal information retrieval for a wide range of web applications. Deep neural networks (DNNs) have been widely employed to extract semantic features from raw samples to improve retrieval accuracy. In addition, hashing is widely used to improve computational and storage efficiency. As such, deep hashing frameworks have been applied for multimodal retrieval tasks. However, there is still a great recognitive gap between primate brain structure-inspired DNNs and humans. On computer vision tasks, well-crafted DNN models can be easily defeated by invisible small attacks, and this phenomenon indicates a large recognition gap between DNN models and humans. Recently, adversarial defense methods have been shown to improve the human-machine recognition alignment in several classification tasks. However, the robustness problem on the retrieval tasks, especially on the deep hashing-based multimodal retrieval models, is still not well studied. Therefore, in this article, we present an adversarially robust training mechanism to improve model robustness for the purpose of human-machine recognition alignment on retrieval tasks. Through extensive experimental results on several social multimodal retrieval benchmarks, we show that the robust training hashing framework proposed can mitigate the recognition gap on retrieval tasks. Our study highlights the necessity of robustness enhancement on deep hashing models.
更多查看译文
关键词
Training, Task analysis, Semantics, Perturbation methods, Feature extraction, Computational modeling, Robustness, Adversarial perturbation, adversarially robust training, deep hashing, multimodal retrieval
AI 理解论文
溯源树
样例
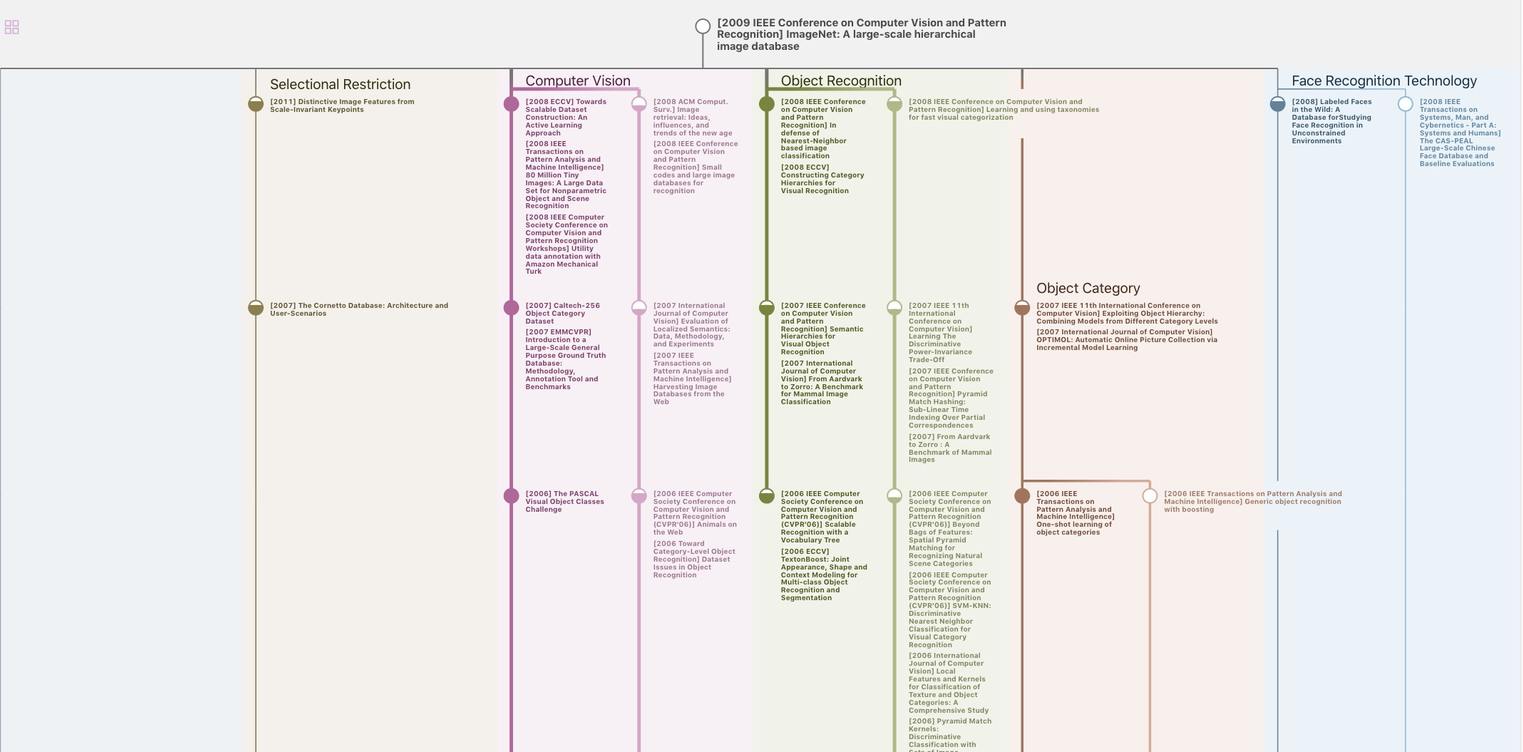
生成溯源树,研究论文发展脉络
Chat Paper
正在生成论文摘要