Architecture and Application Co-Design for Beyond-FPGA Reconfigurable Acceleration Devices
IEEE ACCESS(2022)
摘要
In recent years, field-programmable gate arrays (FPGAs) have been increasingly deployed in datacenters as programmable accelerators that can offer software-like flexibility and custom-hardware-like efficiency for key datacenter workloads. To improve the efficiency of FPGAs for these new datacenter use cases and data-intensive applications, a new class of reconfigurable acceleration devices (RADs) is emerging. In these devices, the FPGA fine-grained reconfigurable fabric is a component of a bigger monolithic or multi-die system-in-package that can incorporate general-purpose software-programmable cores, domain-specialized accelerator blocks, and high-performance networks-on-chip (NoCs) for efficient communication between these system components. The integration of all these components in a RAD results in a huge design space and requires re-thinking the implementation of applications that need to be migrated from conventional FPGAs to these novel devices. In this work, we introduce RAD-Sim, an architecture simulator that allows rapid design space exploration for RADs and facilitates the study of complex interactions between their various components. We also present a case study that highlights the utility of RAD-Sim in re-designing applications for these novel RADs by mapping a state-of-the-art deep learning (DL) inference FPGA overlay to different RAD instances. Our case study illustrates how RAD-Sim can capture a wide variety of reconfigurable architectures, from conventional FPGAs to devices augmented with hard NoCs, specialized matrix-vector blocks, and 3D-stacked multi-die devices. In addition, we show that our tool can help architects evaluate the effect of specific RAD architecture parameters on end-to-end workload performance. Through RAD-Sim, we also show that novel RADs can potentially achieve 2.6x better performance on average compared to conventional FPGAs in the key DL application domain.
更多查看译文
关键词
Deep learning, field-programmable gate arrays, hardware acceleration, network-on-chip, reconfigurable computing
AI 理解论文
溯源树
样例
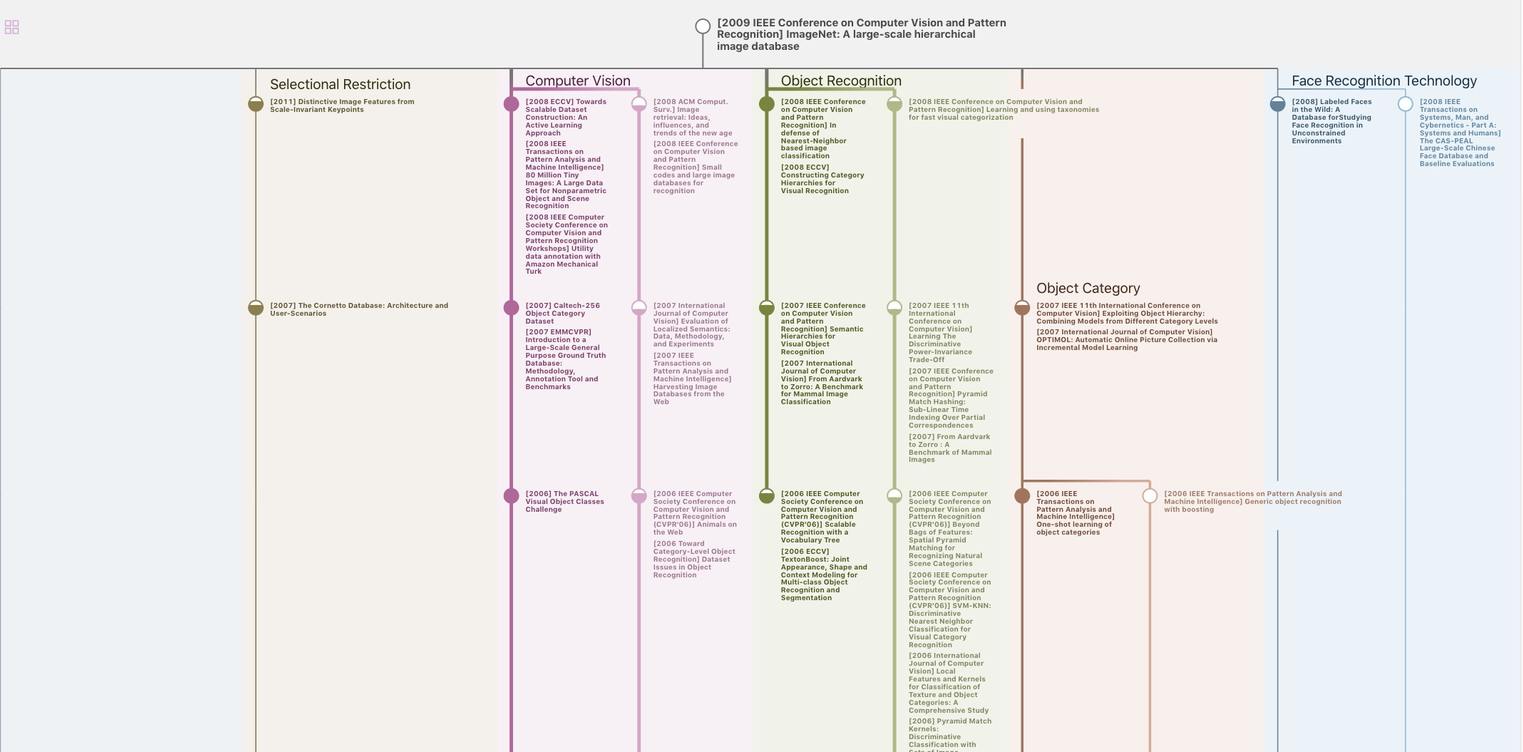
生成溯源树,研究论文发展脉络
Chat Paper
正在生成论文摘要