Accelerating SLIDE: Exploiting Sparsity on Accelerator Architectures
2022 IEEE 36TH INTERNATIONAL PARALLEL AND DISTRIBUTED PROCESSING SYMPOSIUM WORKSHOPS (IPDPSW 2022)(2022)
摘要
A significant trend in machine learning is sparsifying the training of neural networks to reduce the amount of computation required. Algorithms like Sub-LInear Deep learning Engine (SLIDE) [2] use locality-sensitive hashing (LSH) to create sparsity. These sparse training algorithms were originally developed on multi-threaded multicore CPUs. However, they are not well-studied and optimized for accelerator platforms such as GPUs and reconfigurable dataflow architectures (RDAs). In this paper, we study the different variants of the SLIDE algorithm and investigate accuracy-performance tradeoffs on CPU, GPU, and RDAs. The implementation targeting RDA outperforms the GPU by 7.5x. The performance on a limited-memory RDA is improved further by proposing a smart caching algorithm, which is 2 x faster than the baseline RDA. Furthermore, we are able to achieve another 2 x performance by putting all of the weights on-chip using an RDA with enough memory. We believe our work will pave the road for the future development of both algorithm and hardware architecture for sparse training.
更多查看译文
关键词
hardware architecture,accelerating SLIDE,accelerator architectures,machine learning,neural networks,Sub-LInear Deep learning Engine,locality-sensitive hashing,LSH,sparse training algorithms,multithreaded multicore CPUs,accelerator platforms,GPU,reconfigurable dataflow architectures,SLIDE algorithm,accuracy-performance tradeoffs,limited-memory RDA,smart caching algorithm,baseline RDA
AI 理解论文
溯源树
样例
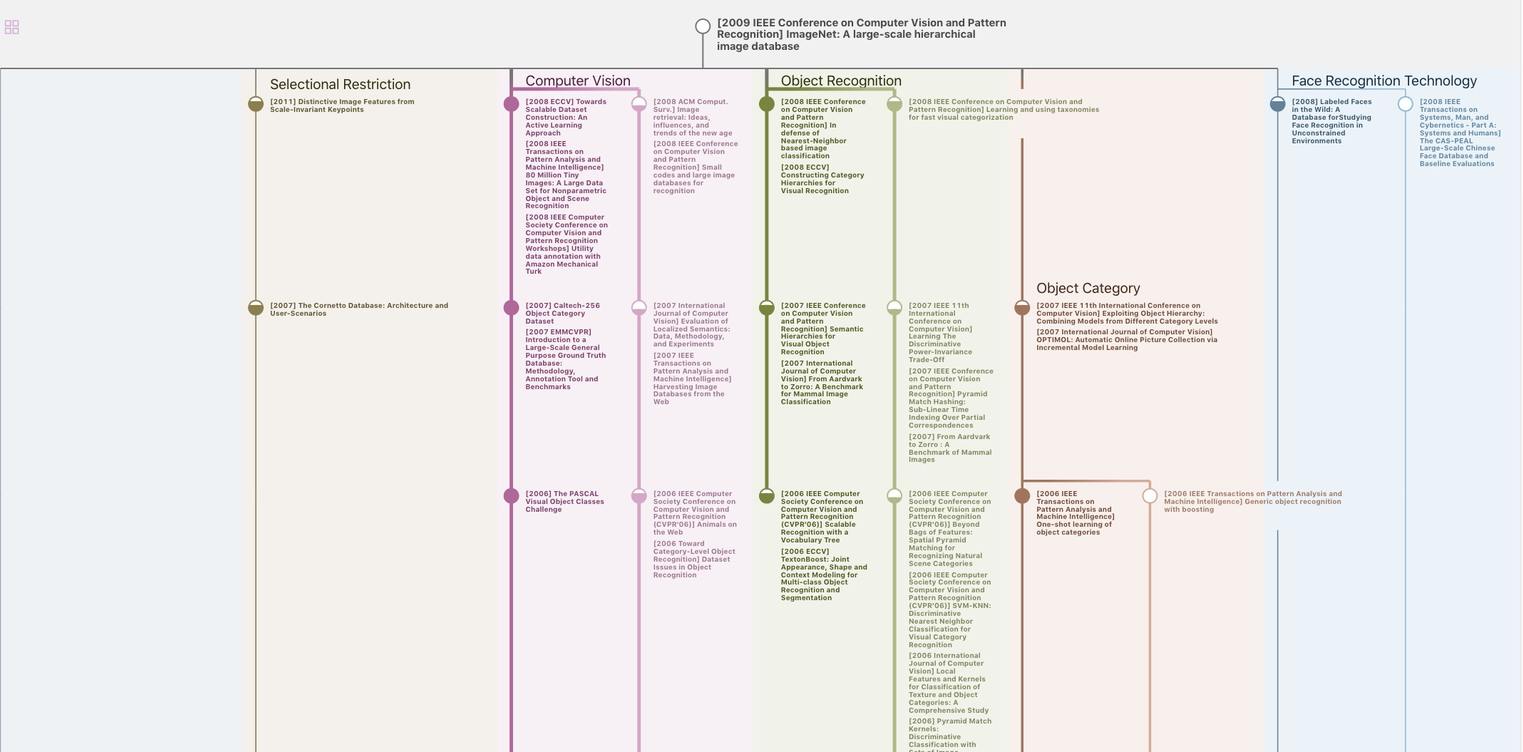
生成溯源树,研究论文发展脉络
Chat Paper
正在生成论文摘要