DiffEdit: Diffusion-based semantic image editing with mask guidance
ICLR 2023(2022)
摘要
Image generation has recently seen tremendous advances, with diffusion models allowing to synthesize convincing images for a large variety of text prompts. In this article, we propose DiffEdit, a method to take advantage of text-conditioned diffusion models for the task of semantic image editing, where the goal is to edit an image based on a text query. Semantic image editing is an extension of image generation, with the additional constraint that the generated image should be as similar as possible to a given input image. Current editing methods based on diffusion models usually require to provide a mask, making the task much easier by treating it as a conditional inpainting task. In contrast, our main contribution is able to automatically generate a mask highlighting regions of the input image that need to be edited, by contrasting predictions of a diffusion model conditioned on different text prompts. Moreover, we rely on latent inference to preserve content in those regions of interest and show excellent synergies with mask-based diffusion. DiffEdit achieves state-of-the-art editing performance on ImageNet. In addition, we evaluate semantic image editing in more challenging settings, using images from the COCO dataset as well as text-based generated images.
更多查看译文
关键词
computer vision,image editing,diffusion models
AI 理解论文
溯源树
样例
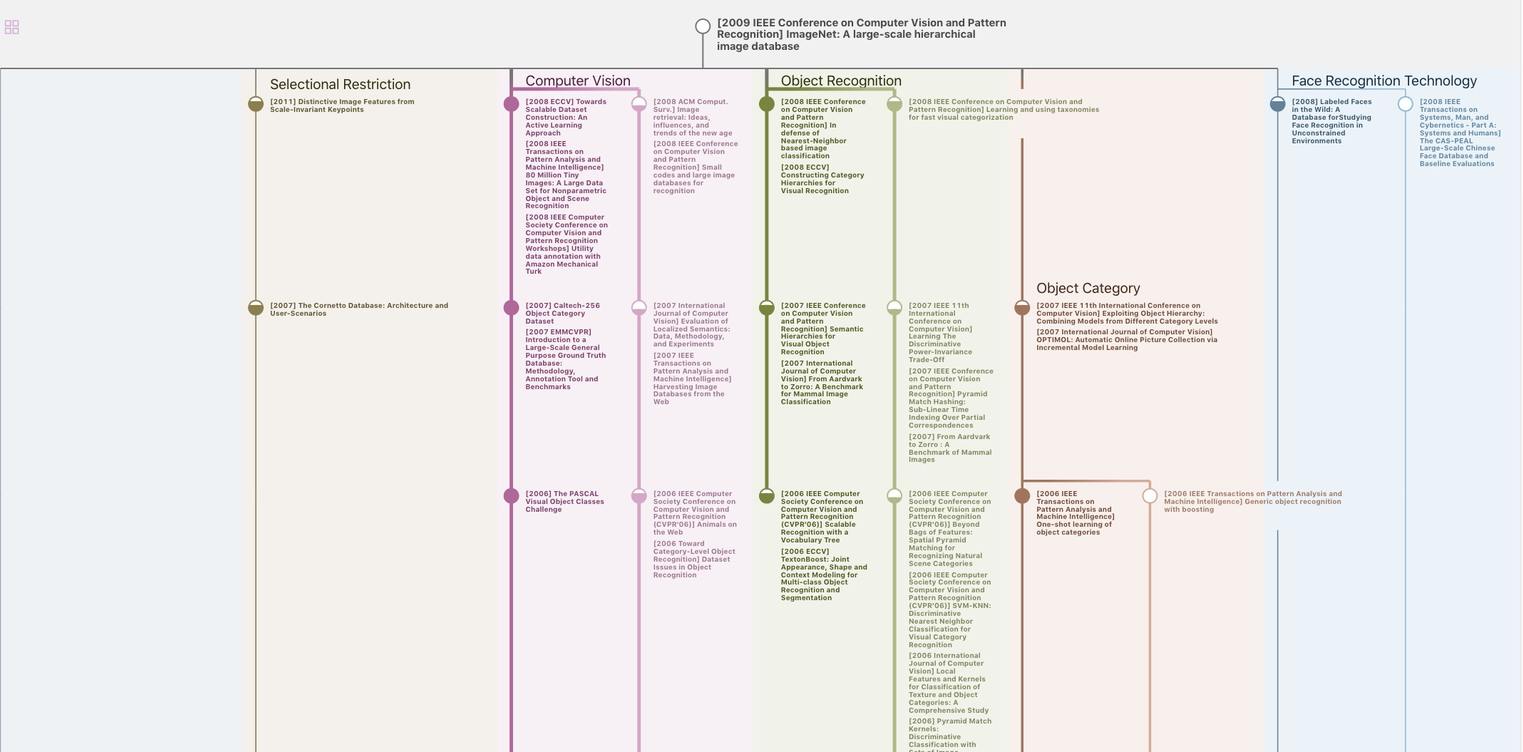
生成溯源树,研究论文发展脉络
Chat Paper
正在生成论文摘要