Robot Navigation With Reinforcement Learned Path Generation and Fine-Tuned Motion Control
arxiv(2023)
摘要
In this letter, we propose a novel reinforcement learning (RL) based path generation (RL-PG) approach for mobile robot navigation without a prior exploration of an unknown environment. Multiple predictive path points are dynamically generated by a deep Markov model optimized using an RL approach for the robot to track. To ensure safety when tracking the predictive points, the robot's motion is fine-tuned by a motion fine-tuning module. Such an approach, using a deep Markov model with RL algorithm for planning, focuses on the relationship between adjacent path points. We analyze the benefits of our proposed approach and show it is more effective and has higher success rates than the RL-based approach DWA-RL (Patel et al. 2021) and a traditional navigation approach APF (Chen et al. 2021). We deploy our model on both simulation and physical platforms and demonstrate our model performs robot navigation effectively and safely.
更多查看译文
关键词
Index Terms-Motion and path planning,RL-based path generation,collision avoidance
AI 理解论文
溯源树
样例
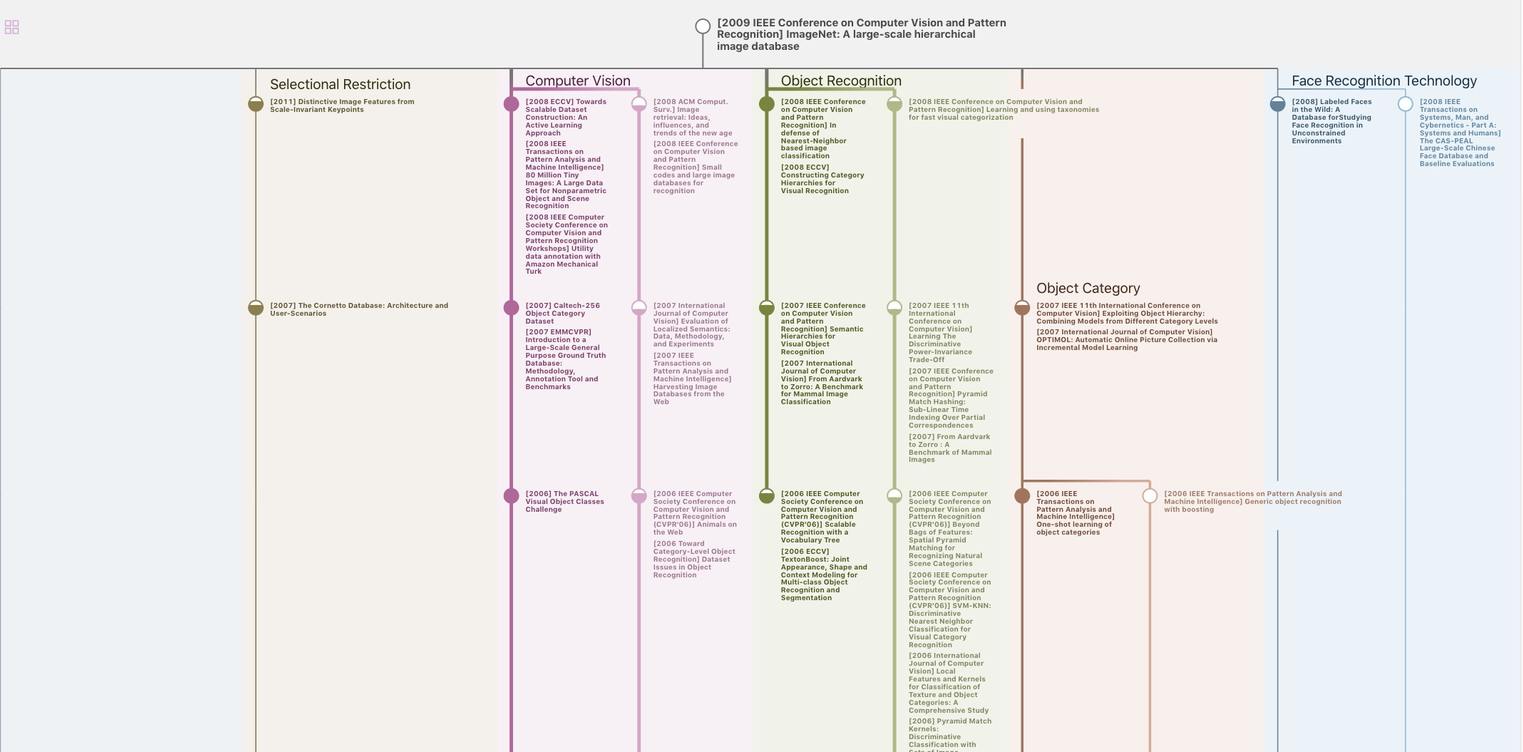
生成溯源树,研究论文发展脉络
Chat Paper
正在生成论文摘要