Malware Detection with Limited Supervised Information via Contrastive Learning on API Call Sequences
International Conference on Information and Communication Security (ICICS)(2022)
摘要
Malware is a software capable of causing damage to computer systems. Conventional malware detection methods either require feature engineering to extract specific features or require a large amount of labeled data to train an end-to-end deep learning model. Both feature engineering and labelling are laborious. In this paper, we propose a semi-supervised contrastive learning malware detection method based on API call sequences with limited label information, called SCLMD. Specifically, a heterogeneous graph is constructed from API behavior to express the rich relationships among labeled and unlabeled software. After extracting the structural and sequential features of software by two encoders, we adopt the cross-view contrastive learning to obtain the shared and consistent feature of software. A hybrid positive selection strategy is designed to select positive pairs for contrastive learning by the guidance of the limited label information. Experimental results on two real world datasets show that the SCLMD outperforms the baseline methods, especially when the supervised information is limited.
更多查看译文
关键词
Malware detection,Contrastive learning,Heterogeneous graph neural network
AI 理解论文
溯源树
样例
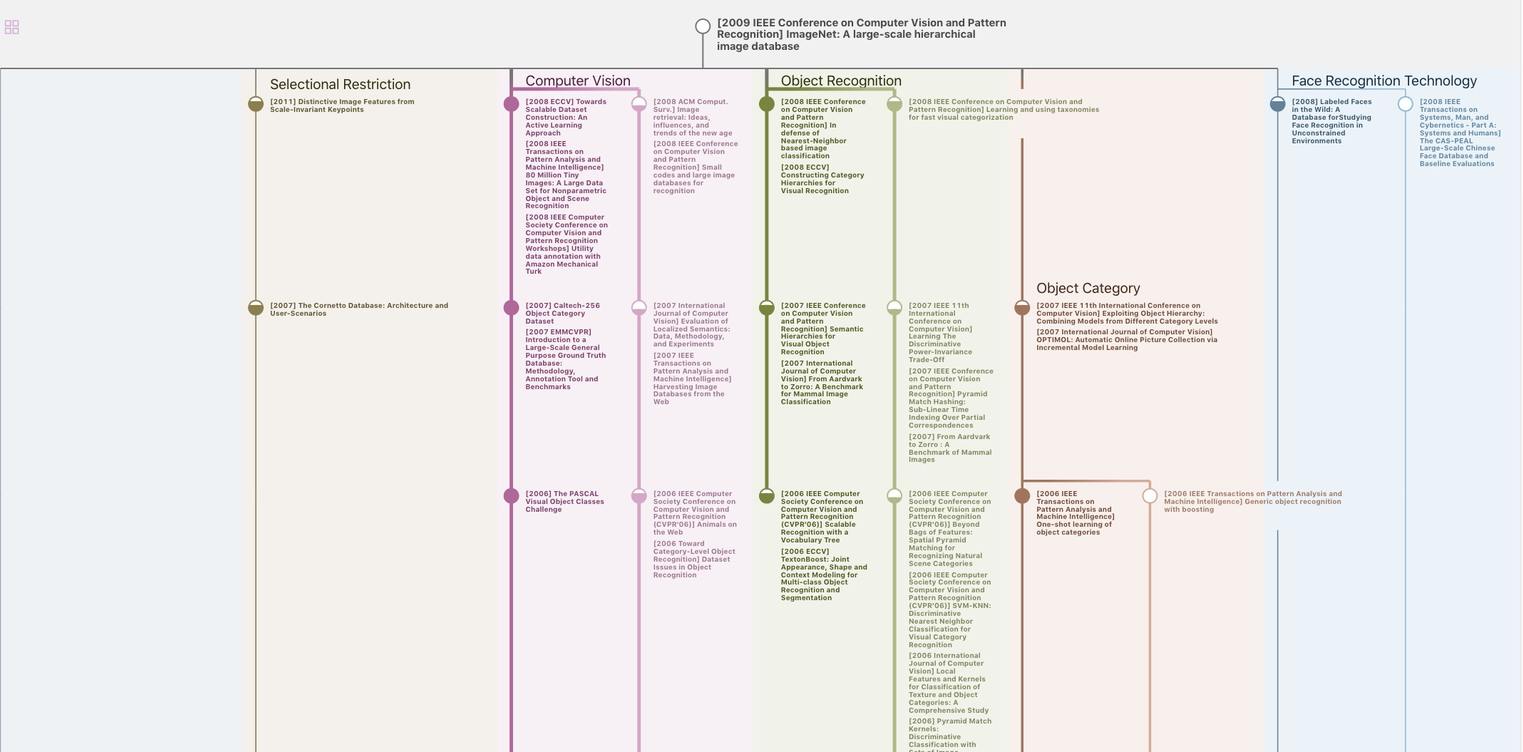
生成溯源树,研究论文发展脉络
Chat Paper
正在生成论文摘要