PathFlow: A normalizing flow generator that finds transition paths.
International Conference on Uncertainty in Artificial Intelligence(2022)
摘要
Sampling from a Boltzmann distribution to calculate important macro statistics is one of the central tasks in the study of large atomic and molecular systems. Recently, a one-shot configuration sampler, the Boltzmann generator [Noé et al., 2019], is introduced. Though a Boltzmann generator can directly generate independent metastable states, it lacks the ability to find transition pathways and describe the whole transition process. In this paper, we propose PathFlow that can function as a one-shot generator as well as a transition pathfinder. More specifically, a normalizing flow model is constructed to map the base distribution and linear interpolated path in the latent space to the Boltzmann distribution and a minimum (free) energy path in the configuration space simultaneously. PathFlow can be trained by standard gradient-based optimizers using the proposed gradient estimator with a theoretical guarantee. PathFlow, validated with the extensively studied examples including a synthetic Müller potential and Alanine dipeptide, shows a remarkable performance.
更多查看译文
AI 理解论文
溯源树
样例
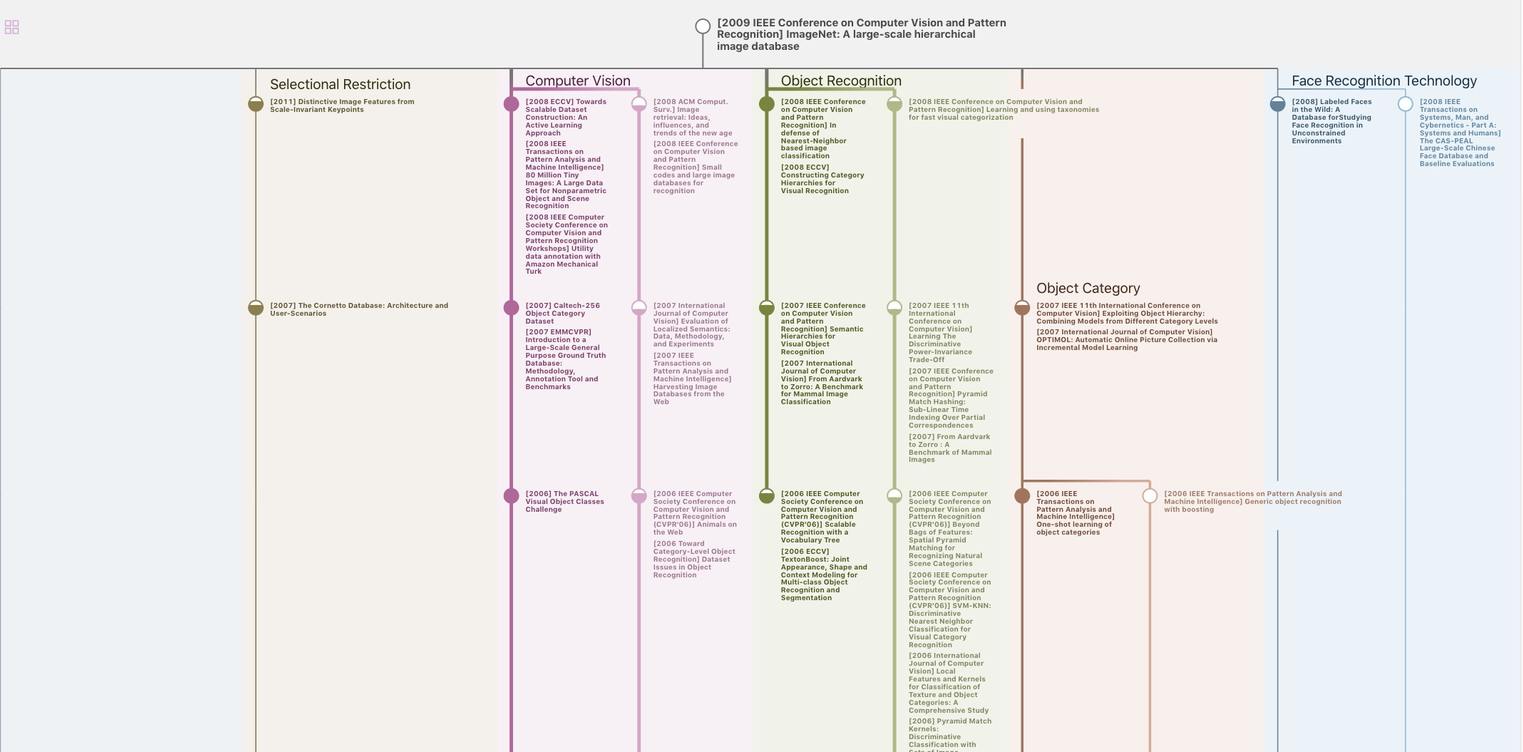
生成溯源树,研究论文发展脉络
Chat Paper
正在生成论文摘要