ShapGraph: An Holistic View of Explanations through Provenance Graphs and Shapley Values
PROCEEDINGS OF THE 2022 INTERNATIONAL CONFERENCE ON MANAGEMENT OF DATA (SIGMOD '22)(2022)
摘要
Explaining query results is an essential tool for enhancing the transparency and quality of data processing, and has been extensively studied in recent years. In particular, Data Provenance - the tracking of transformations that data undergoes in query evaluation has been shown to be a key component of explanations. A hurdle that remains is that data provenance itself is often too large and complex to be presented in its entirety. To that end, we propose to leverage novel advancements on quantifying and computing the contributions of individual input tuples to query answers, based on the game-theoretic notion of the Shapley value. Our proposed prototype solution, called ShapGraph, combines the global view of explanations through provenance graphs with a local quantification of contributions through Shapley values. The graphical interface allows users to switch between and combine these two views to obtain a deeper understanding of the most influential parts of the database and how they interact to yield query answers.
更多查看译文
关键词
Shapley value, Data provenance
AI 理解论文
溯源树
样例
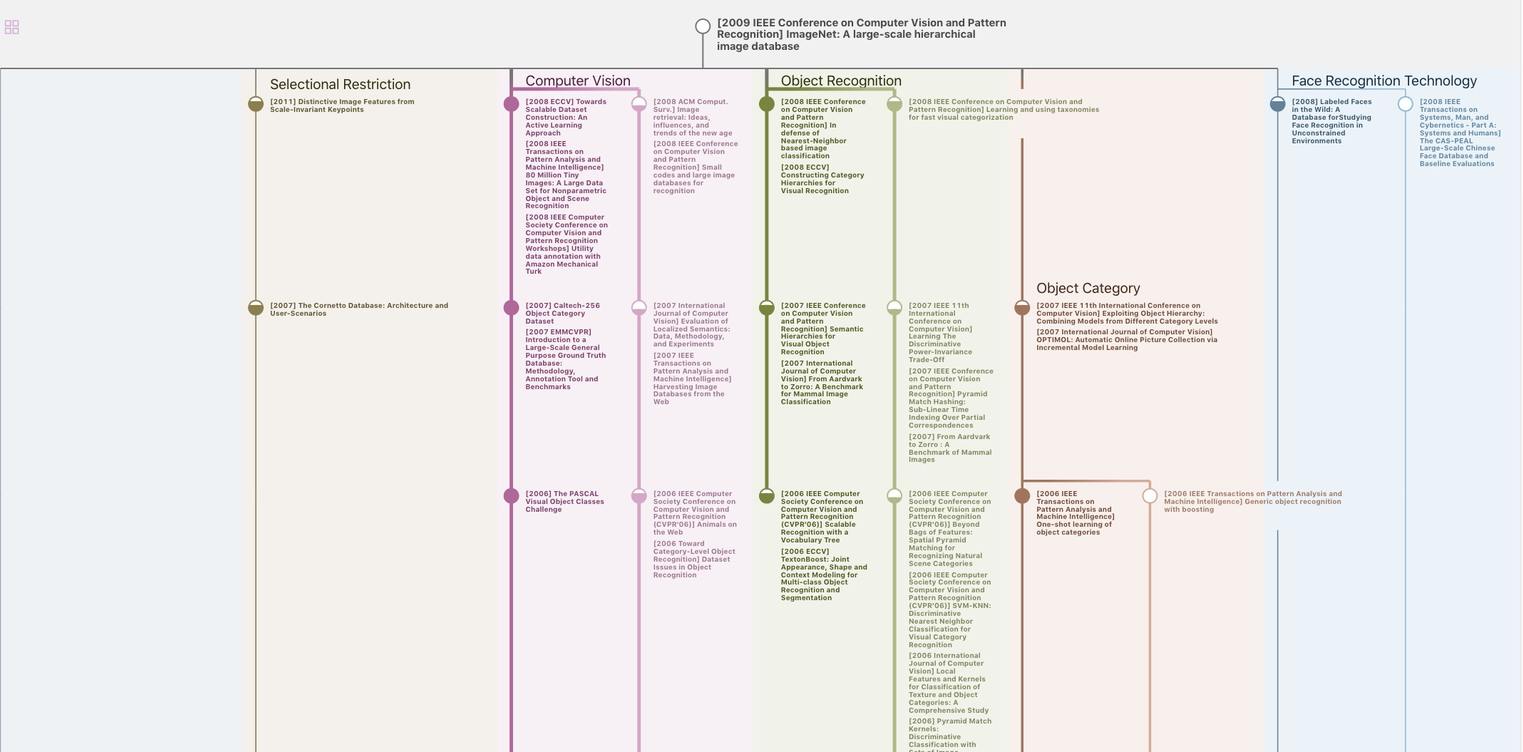
生成溯源树,研究论文发展脉络
Chat Paper
正在生成论文摘要