Reducing Cross-Topic Political Homogenization in Content-Based News Recommendation
ACM Conference On Recommender Systems(2022)
摘要
BSTRACT Content-based news recommenders learn words that correlate with user engagement and recommend articles accordingly. This can be problematic for users with diverse political preferences by topic — e.g., users that prefer conservative articles on one topic but liberal articles on another. In such instances, recommenders can have a homogenizing effect by recommending articles with the same political lean on both topics, particularly if both topics share salient, politically polarized terms like “far right” or “radical left.” In this paper, we propose attention-based neural network models to reduce this homogenization effect by increasing attention on words that are topic specific while decreasing attention on polarized, topic-general terms. We find that the proposed approach results in more accurate recommendations for simulated users with such diverse preferences.
更多查看译文
AI 理解论文
溯源树
样例
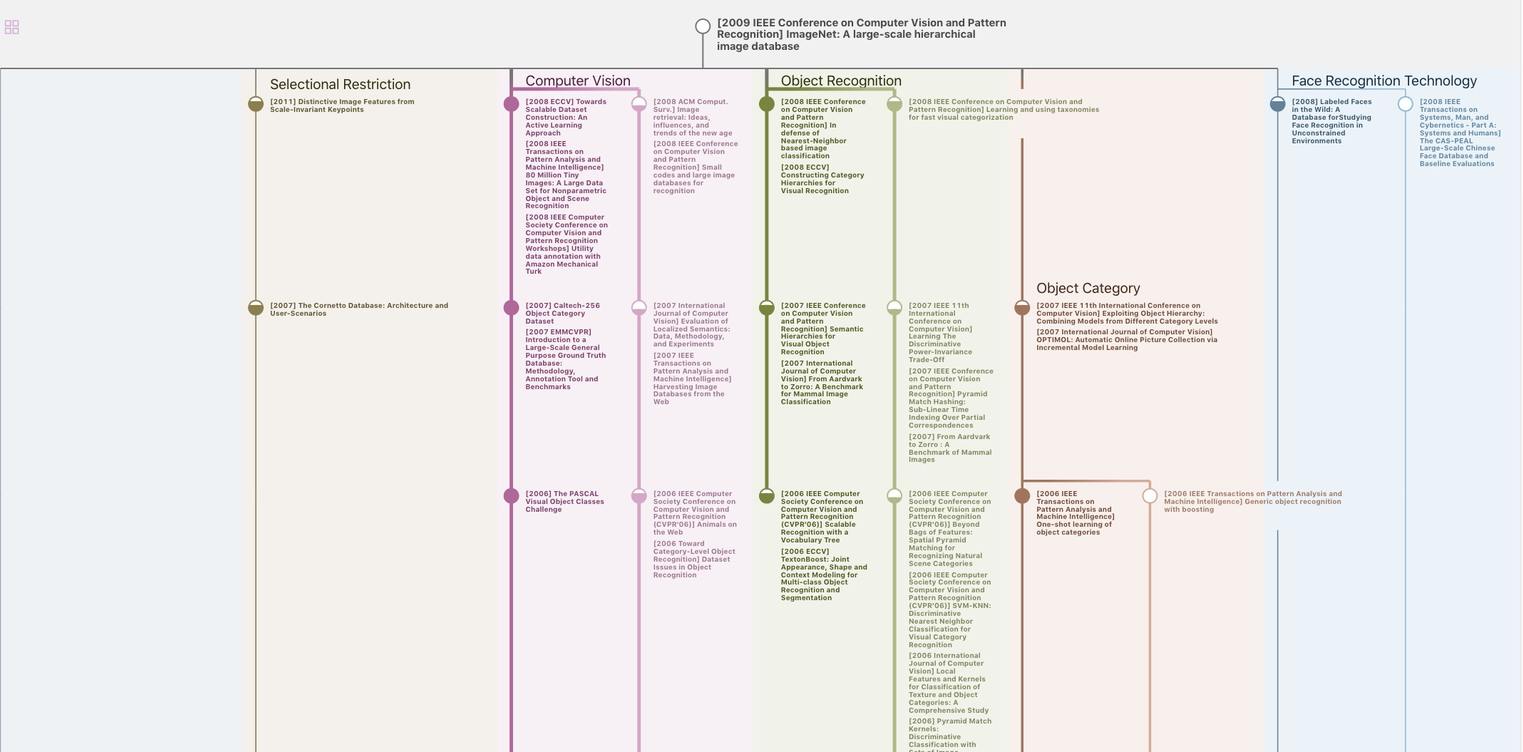
生成溯源树,研究论文发展脉络
Chat Paper
正在生成论文摘要