Information Bottleneck Measurement for Compressed Sensing Image Reconstruction
IEEE SIGNAL PROCESSING LETTERS(2022)
摘要
Image Compressed Sensing (CS) has achieved a lot of performance improvement thanks to advances in deep networks. The CS method is generally composed of a sensing and a decoder. The sensing and decoder networks have a significant impact on the reconstruction performance, and it is obvious that both two networks must be in harmony. However, previous studies have focused on designing the loss function considering only the decoder network. In this paper, we propose a novel training process that can learn sensing and decoder networks simultaneously using Information Bottleneck (IB) theory. By maximizing importance through proposed importance generator, the sensing network is trained to compress important information for image reconstruction of the decoder network. The representative experimental results demonstrate that the proposed method is applied in recently proposed CS algorithms and increases the reconstruction performance with large margin in all CS ratios.
更多查看译文
关键词
Sensors, Generators, Decoding, Training, Image reconstruction, Image coding, Loss measurement, Information bottleneck, image compressed sens- ing, deep learning
AI 理解论文
溯源树
样例
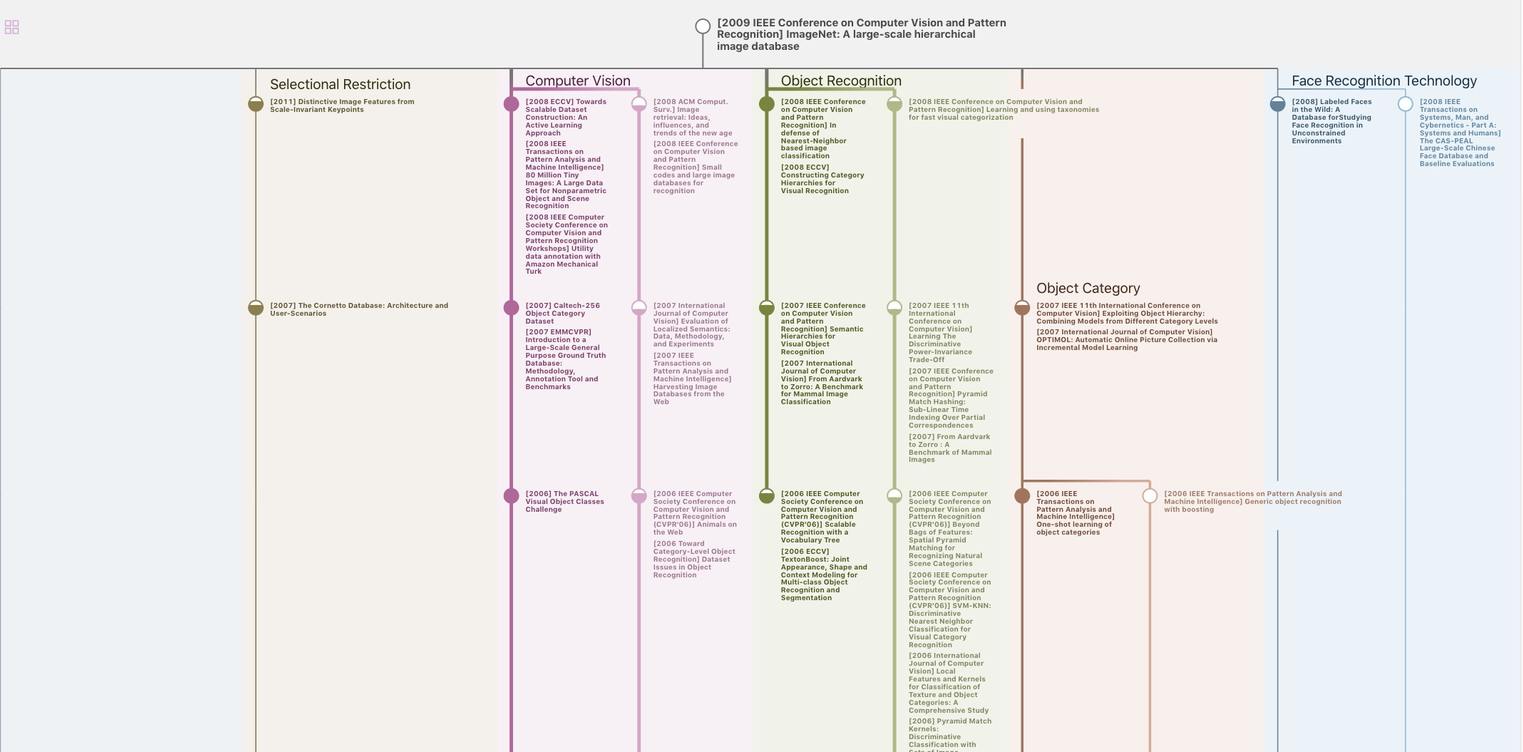
生成溯源树,研究论文发展脉络
Chat Paper
正在生成论文摘要