Learning A Continuous and Reconstructible Latent Space for Hardware Accelerator Design
2022 IEEE International Symposium on Performance Analysis of Systems and Software (ISPASS)(2022)
摘要
The hardware design space is high-dimensional and discrete. Systematic and efficient exploration of this space has been a significant challenge. Central to this problem is the intractable search complexity that grows exponentially with the design choices and the discrete nature of the search space. This work investigates the feasibility of learning a meaningful low-dimensional continuous representation for hardware designs to reduce such complexity and facilitate the search process. We devise a variational autoencoder (VAE)-based design space exploration framework called VAESA, to encode the hardware design space in a compact and continuous representation. We show that black-box and gradient-based design space exploration algorithms can be applied to the latent space, and design points optimized in the latent space can be reconstructed to high-performance realistic hardware designs. Our experiments show that performing the design space search on the latent space consistently leads to the optimal design point under a fixed number of samples. In addition, the latent space can improve the sample efficiency of the original algorithm by 6.8$\times$ and can discover hardware designs that are up to 5% more efficient than the optimal design searched directly in the high-dimensional input space.
更多查看译文
关键词
accelerator,design space exploration,latent space,variational autoencoder,vae,representation learning
AI 理解论文
溯源树
样例
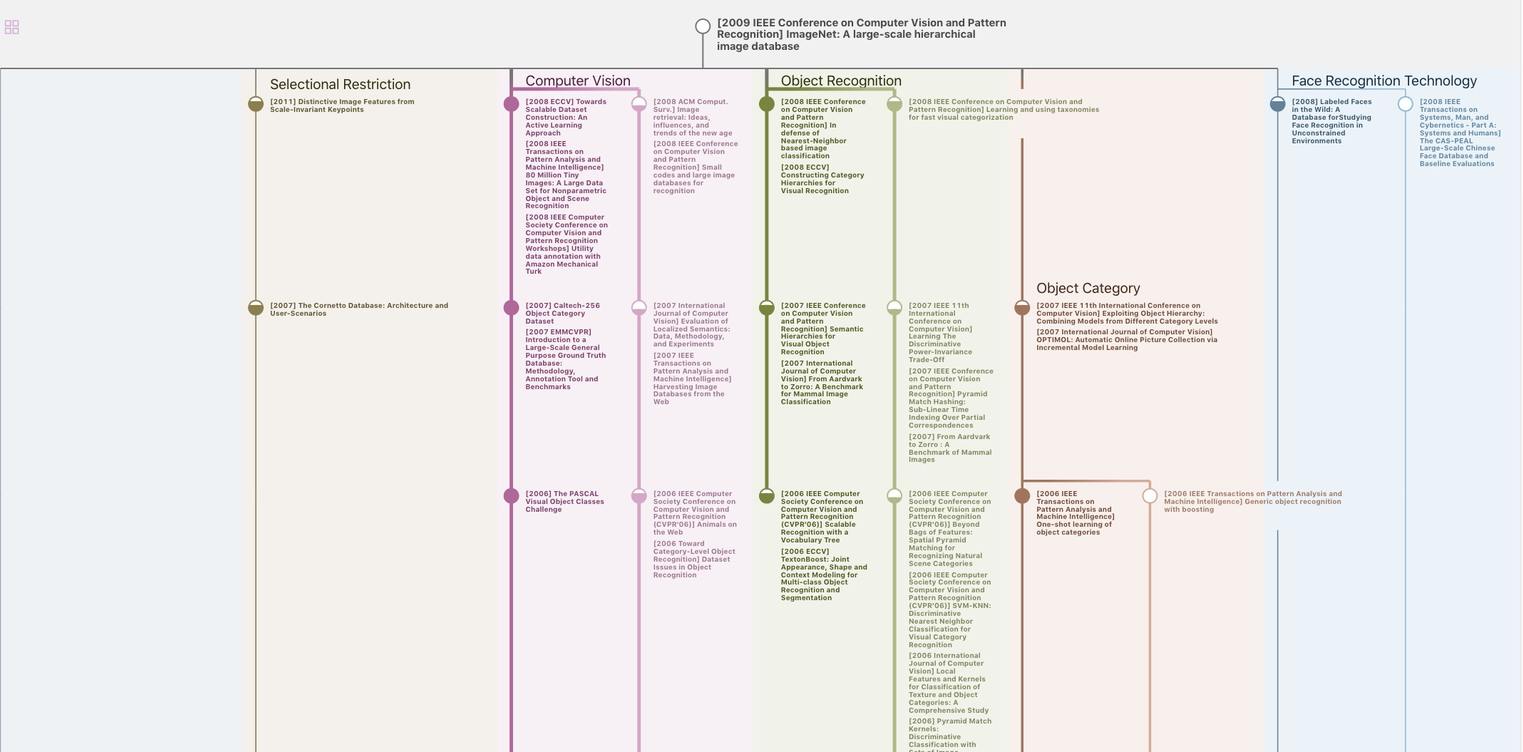
生成溯源树,研究论文发展脉络
Chat Paper
正在生成论文摘要