Game Theoretic Rating in N-player general-sum games with Equilibria
arxiv(2022)
摘要
Rating strategies in a game is an important area of research in game theory and artificial intelligence, and can be applied to any real-world competitive or cooperative setting. Traditionally, only transitive dependencies between strategies have been used to rate strategies (e.g. Elo), however recent work has expanded ratings to utilize game theoretic solutions to better rate strategies in non-transitive games. This work generalizes these ideas and proposes novel algorithms suitable for N-player, general-sum rating of strategies in normal-form games according to the payoff rating system. This enables well-established solution concepts, such as equilibria, to be leveraged to efficiently rate strategies in games with complex strategic interactions, which arise in multiagent training and real-world interactions between many agents. We empirically validate our methods on real world normal-form data (Premier League) and multiagent reinforcement learning agent evaluation.
更多查看译文
关键词
game,n-player,general-sum
AI 理解论文
溯源树
样例
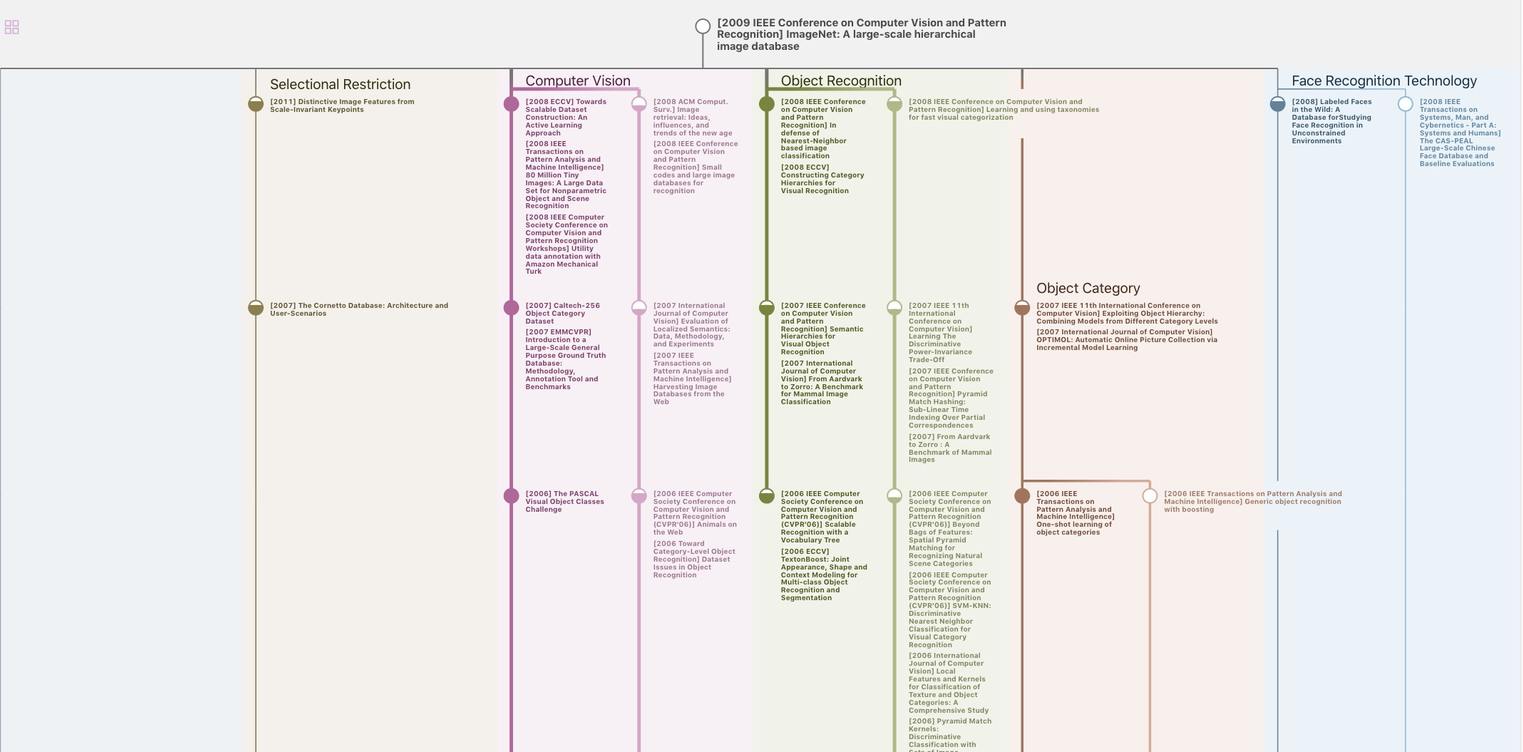
生成溯源树,研究论文发展脉络
Chat Paper
正在生成论文摘要