A New Neural Beamformer for Multi-channel Speech Separation
Journal of Signal Processing Systems(2022)
摘要
Speech separation is the key to many speech backend tasks, like multi-speaker speech recognition. In recent years, with the development and aid of deep learning technology, many single-channel speech separation models have shown good performance in weak reverberant environment. However, with the presence of reverberation, the multi-channel speech separation model still has greater advantages. Among them, the deep neural network (DNN) based beamformers (also known as neural beamformers) have achieved significant improvements in separation quality. The current neural beamformers can’t jointly optimize beamforming layers and DNN layers when using the prior knowledge of the existing beamforming algorithms, which may make the model unable to obtain the optimal separation performance. In order to solve this problem, this paper employs a set of beamformers that uniformly sample the space as a learning module in the neural network, and the initial values of their coefficients are determined by the existing maximum directivity factor (DF) beamformer. Furthermore, to obtain beam representations of source signals when their directions are unknown, a cross-attention mechanism is introduced. The experimental results show that in the separation task with reverberation, the proposed method has better performance than the current state-of-the-art temporal neural beamformer filter-and-sum network (FasNet) and several mainstream multi-channel speech separation approaches in terms of scale-invariant signal-to-noise ratio (SI-SNR), perceptual evaluation of speech quality (PESQ) and short-time objective intelligibility measure (STOI).
更多查看译文
关键词
Multi-channel speech separation,Neural beamformer,Microphone array,Attention mechanism
AI 理解论文
溯源树
样例
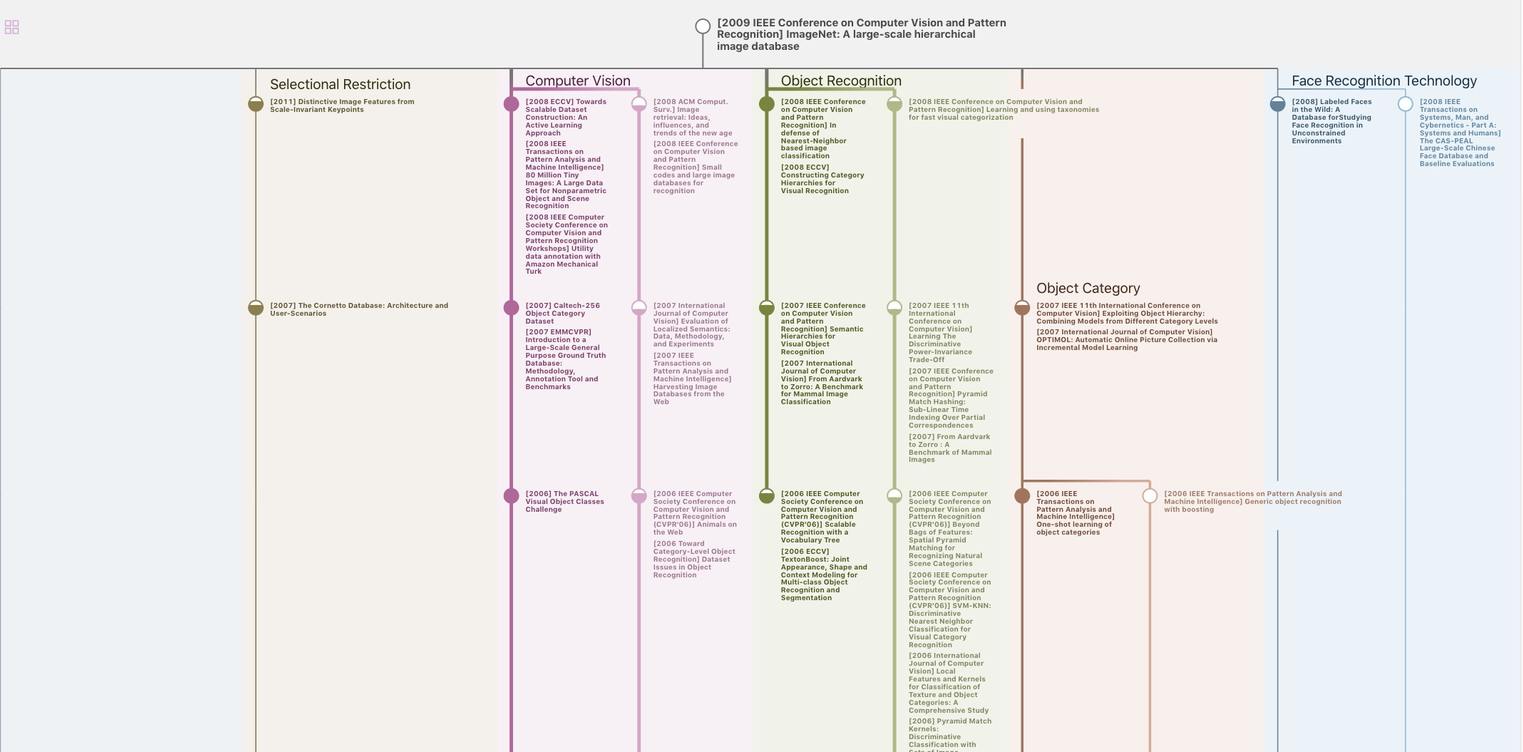
生成溯源树,研究论文发展脉络
Chat Paper
正在生成论文摘要