Unsupervised learning of visual representations using delay-weight spike-timing-dependent plasticity
IEEE International Joint Conference on Neural Network (IJCNN)(2022)
摘要
Unsupervised learning in Spiking Neural Networks (SNN) is performed by adjusting the synaptic parameters with spike-timing-dependent plasticity rules (STDP), that leverage the firing times of neurons. Commonly the targetted parameters are limited to synaptic weights. However, recent empirical evidences suggest that synaptic delays are not fixed, but plastic parameters affecting the firing time of neurons. The firing times are crucials as biological evidence strongly supports that information is carried in the temporal domain, through precise spike timing. Unlike weights, delays intrinsically operate in the temporal domain. Thus, delays can serve as a meaningful way to compute and learn for living and artificial systems. In this regard, this work proposes novel STDP rules to learn delays and weights in a SNN in order to extract visual representations. These representations are learned from patterns of spikes that encode images in the relative spike timing. One STDP rule adjusts the delays, another one the weights. The rules operate locally both in space and time, making them biologically plausible and neuromorphic-friendly. The model is evaluated on natural images. Numerical experimental results demonstrate state-of-the-art performances in terms of reconstruction error.
更多查看译文
关键词
unsupervised learning,representations learning,spike-timing-dependent plasticity,spiking neural networks
AI 理解论文
溯源树
样例
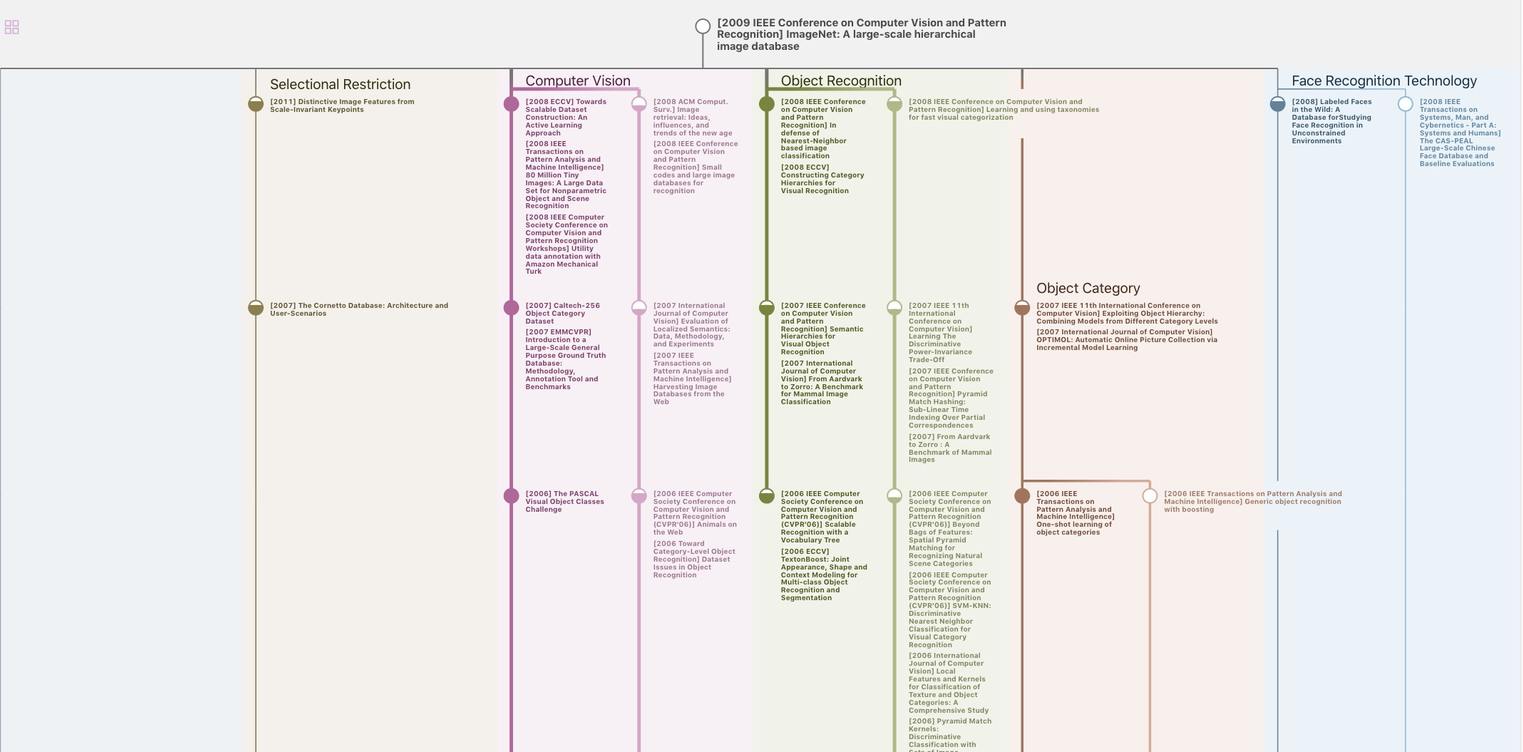
生成溯源树,研究论文发展脉络
Chat Paper
正在生成论文摘要