Feature Decoupling in Self-supervised Representation Learning for Open Set Recognition
arxiv(2022)
摘要
Assuming unknown classes could be present during classification, the open set recognition (OSR) task aims to classify an instance into a known class or reject it as unknown. In this paper, we use a two-stage training strategy for the OSR problems. In the first stage, we introduce a self-supervised feature decoupling method that finds the content features of the input samples from the known classes. Specifically, our feature decoupling approach learns a representation that can be split into content features and transformation features. In the second stage, we fine-tune the content features with the class labels. The fine-tuned content features are then used for the OSR problems. Moreover, we consider an unsupervised OSR scenario, where we cluster the content features learned from the first stage. To measure representation quality, we introduce intra-inter ratio (IIR). Our experimental results indicate that our proposed self-supervised approach outperforms others in image and malware OSR problems. Also, our analyses indicate that IIR is correlated with OSR performance.
更多查看译文
关键词
Open set recognition,self-supervised learning,unsupervised learning,representation learning
AI 理解论文
溯源树
样例
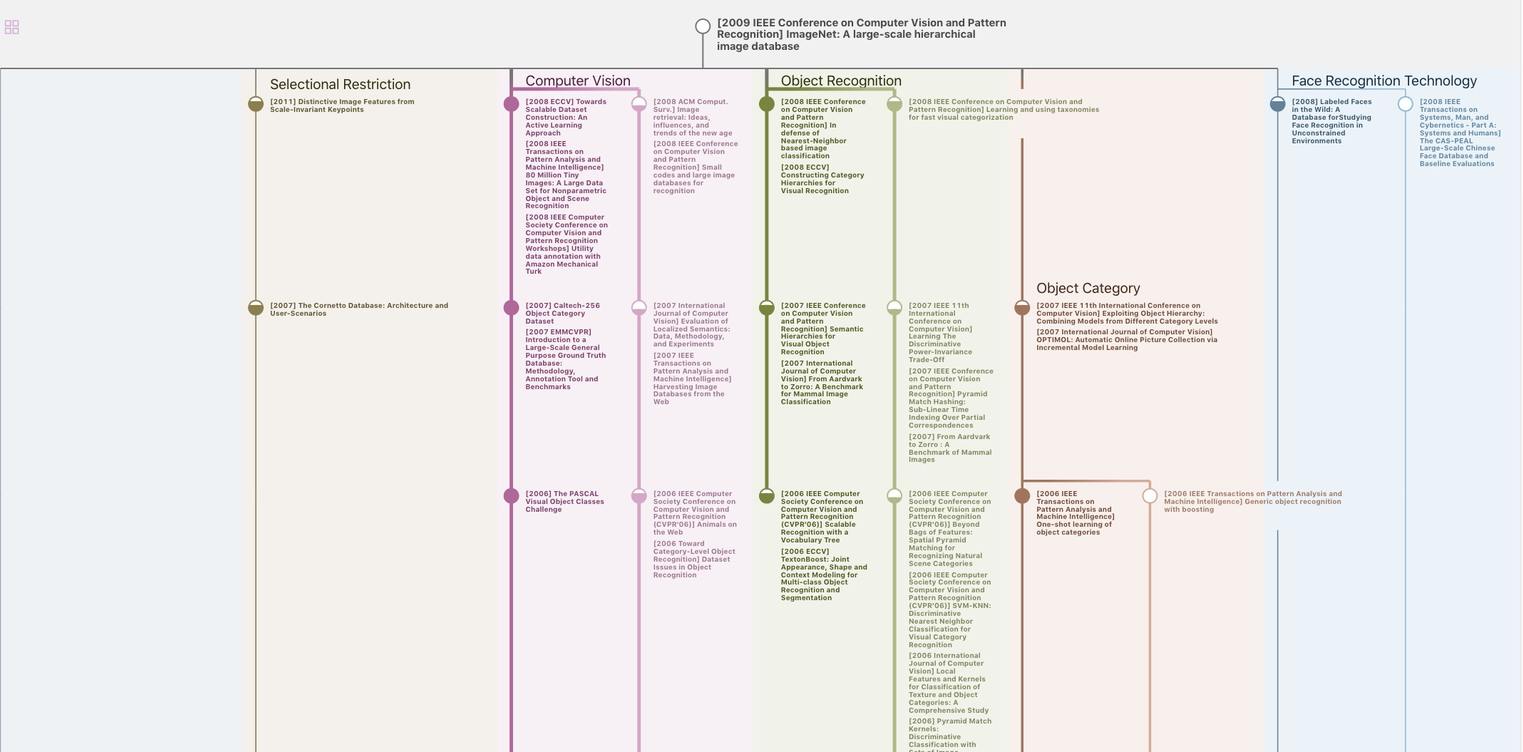
生成溯源树,研究论文发展脉络
Chat Paper
正在生成论文摘要