Fair Robust Active Learning by Joint Inconsistency
2023 IEEE/CVF INTERNATIONAL CONFERENCE ON COMPUTER VISION WORKSHOPS, ICCVW(2023)
摘要
We introduce a new learning framework, Fair Robust Active Learning (FRAL), generalizing conventional active learning to fair and adversarial robust scenarios. This framework enables us to achieve fair-performance and fair-robustness with limited labeled data, which is essential for various annotation-expensive visual applications with safety-critical needs. However, existing fairness-aware data selection strategies face two challenges when applied to the FRAL framework: they are either ineffective under severe data imbalance or inefficient due to huge computations of adversarial training. To address these issues, we develop a novel Joint INconsistency (JIN) method that exploits prediction inconsistencies between benign and adversarial inputs and between standard and robust models. By leveraging these two types of easy-to-compute inconsistencies simultaneously, JIN can identify valuable samples that contribute more to fairness gains and class imbalance mitigation in both standard and adversarial robust settings. Extensive experiments on diverse datasets and sensitive groups demonstrate that our approach outperforms existing active data selection baselines, achieving fair-performance and fair-robustness under white-box PGD attacks.
更多查看译文
AI 理解论文
溯源树
样例
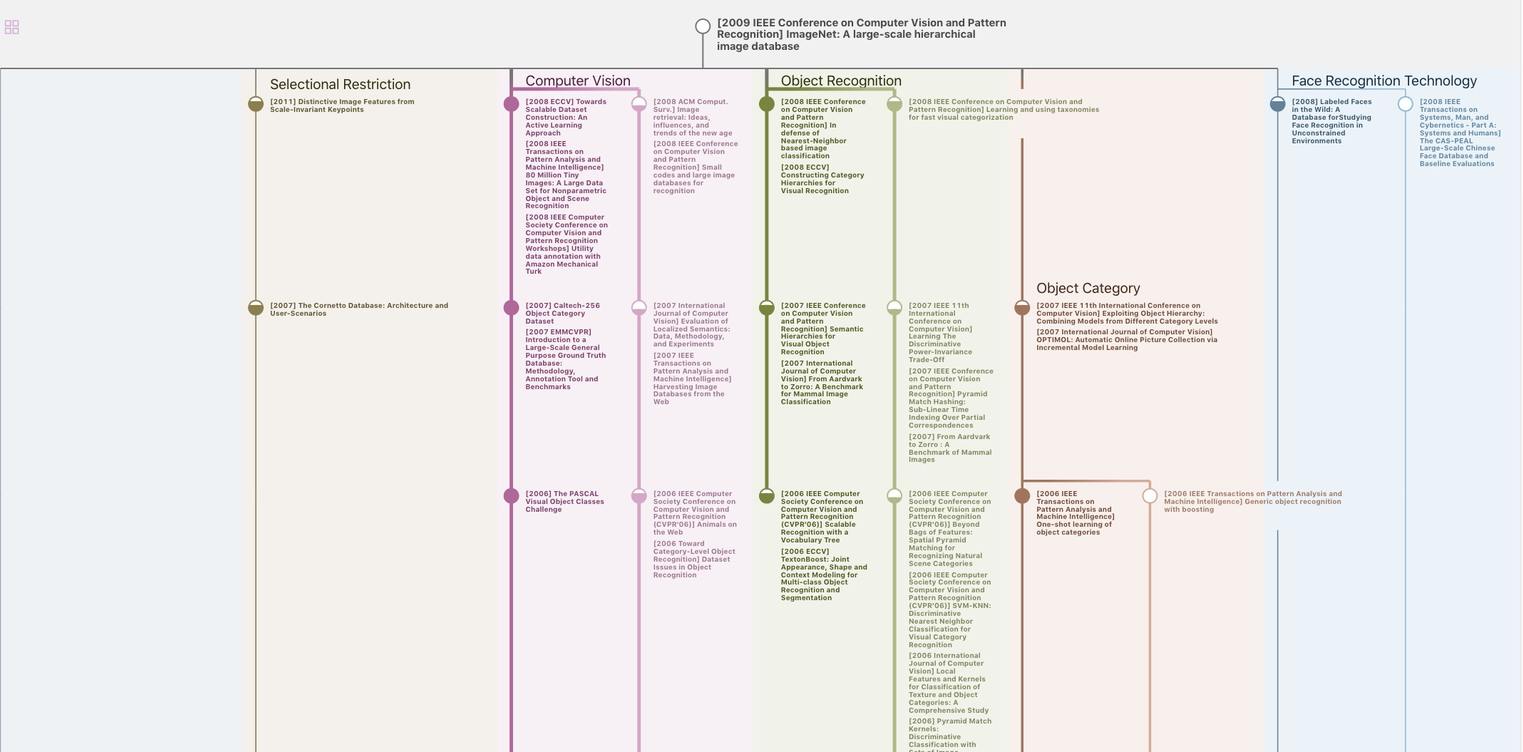
生成溯源树,研究论文发展脉络
Chat Paper
正在生成论文摘要