Power of Explanations: Towards automatic debiasing in hate speech detection
2022 IEEE 9th International Conference on Data Science and Advanced Analytics (DSAA)(2022)
摘要
Hate speech detection is a common downstream application of natural language processing (NLP) in the real world. In spite of the increasing accuracy, current data-driven approaches could easily learn biases from the imbalanced data distributions originating from humans. The deployment of biased models could further enhance the existing social biases. But unlike handling tabular data, defining and mitigating biases in text classifiers, which deal with unstructured data, are more challenging. A popular solution for improving machine learning fairness in NLP is to conduct the debiasing process with a list of potentially discriminated words given by human annotators. In addition to suffering from the risks of overlooking the biased terms, exhaustively identifying bias with human annotators are unsustainable since discrimination is variable among different datasets and may evolve over time. To this end, we propose an automatic misuse detector (MiD) relying on an explanation method for detecting potential bias. And built upon that, an end-to-end debiasing framework with the proposed staged correction is designed for text classifiers without any external resources required.
更多查看译文
关键词
AI fairness,bias detection,bias mitigation,explainable AI,text classification
AI 理解论文
溯源树
样例
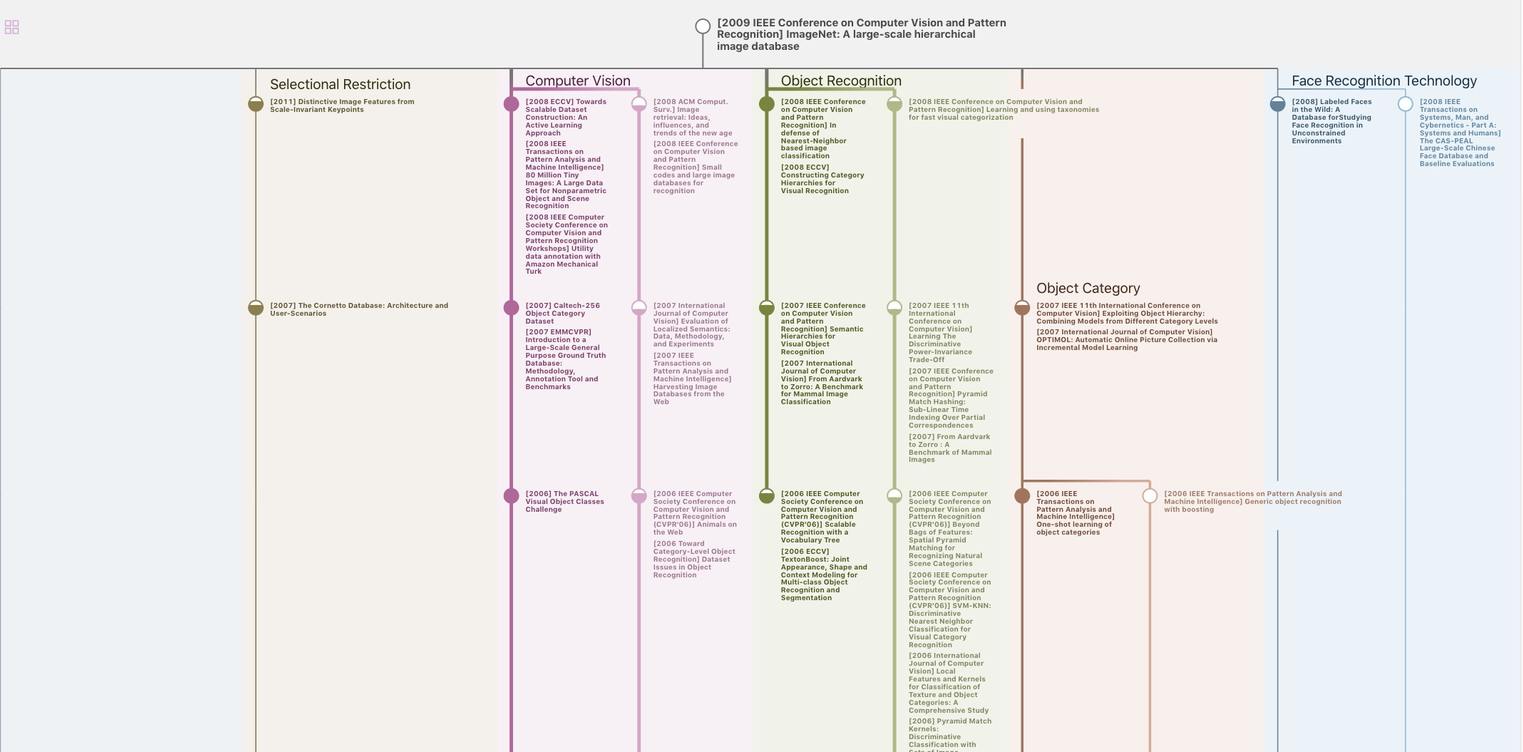
生成溯源树,研究论文发展脉络
Chat Paper
正在生成论文摘要