A deep learning approach to cardiovascular disease classification using empirical mode decomposition for ECG feature extraction
Biomedical Signal Processing and Control(2023)
摘要
Deep learning has achieved promising results on a broad spectrum of tasks using an end-to-end approach, and domain-specific knowledge can be used to supplement it by either constraining the solution-space, or to transform data such that relevant signals are more influential during the training process. This is especially important in the context of continuous recordings or wearable-based monitoring, where signal quality may be constrained by hardware, such as a limited number of available electrodes, or by external perturbations. In this study, a global feature extraction method for multi-periodic signals is proposed, which effectively classifies multi-cycle normal and abnormal heartbeats. The abnormal heartbeats are classed as the Left Bundle Branch Block beat (LBBB), Right Bundle Branch Block beat (RBBB), and Paced beat (P). The signal analysis process of the electrocardiogram (ECG) recordings consist of three main stages: (I) segmentation of the ECG data and partitioning of the data set; (II) generation of an overall feature map representing the “heartbeat condition” based on Empirical Mode Decomposition (EMD); and (III) a classification stage for determining the patient’s heartbeat condition. The extracted feature image is used to classify the heartbeat condition using a two-dimensional Convolutional Neural Network (CNN). This method is applied to the publicly available MIT-BIH arrhythmia database. Experimental results show that the reconstructed ECG features outperform use of the raw ECG signals. Under self-test conditions of 3 min of ECG signal, the Total Classification Accuracy (TCA) was approximately 99.01%, while the TCA without the proposed method was approximately 90.69%.
更多查看译文
关键词
EMD,2-D CNN,Heart disease classification,Deep learning,Denoising ECG,IMF,Cardiovascular
AI 理解论文
溯源树
样例
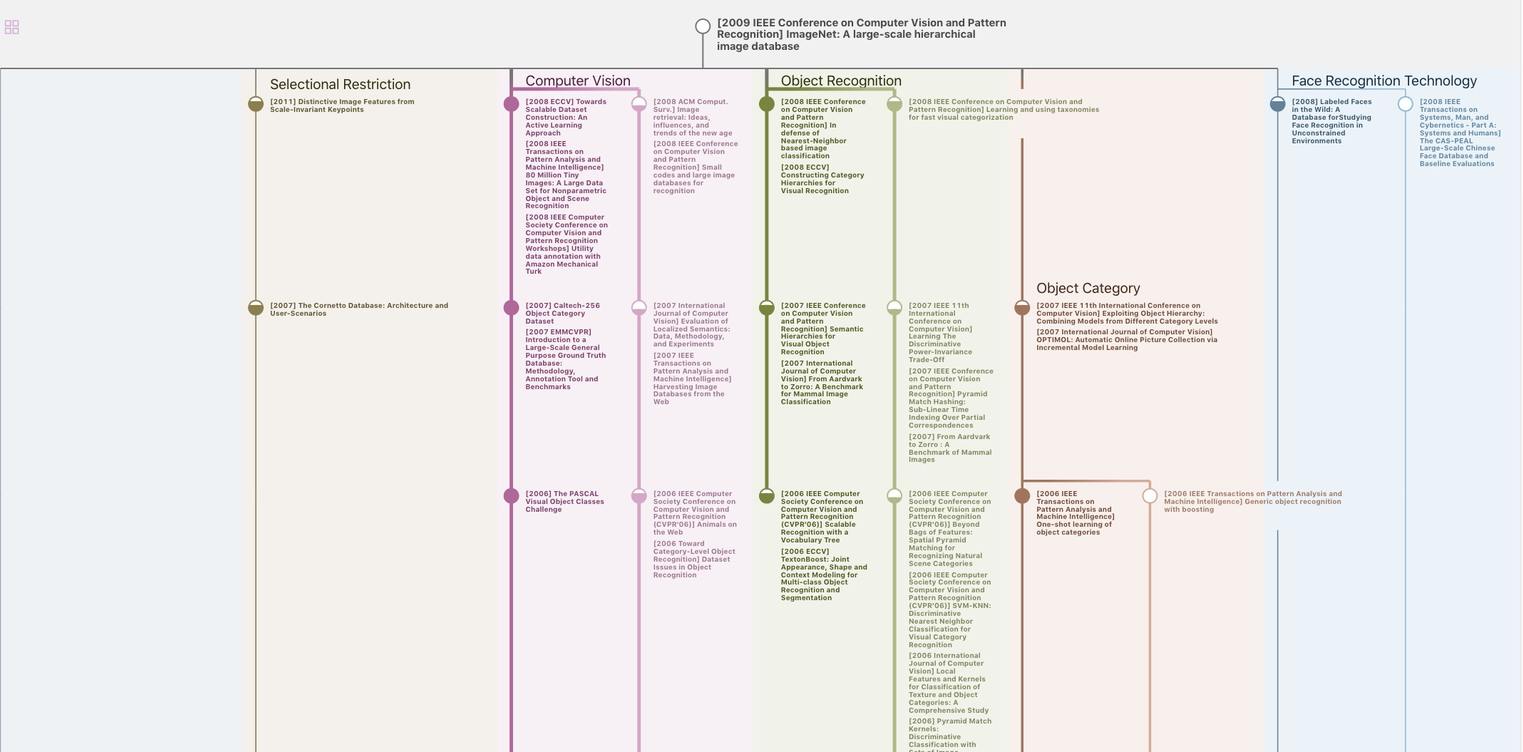
生成溯源树,研究论文发展脉络
Chat Paper
正在生成论文摘要