Mitigating Representation Bias in Action Recognition: Algorithms and Benchmarks
arxiv(2022)
摘要
Deep learning models have achieved excellent recognition results on large-scale video benchmarks. However, they perform poorly when applied to videos with rare scenes or objects, primarily due to the bias of existing video datasets. We tackle this problem from two different angles: algorithm and dataset. From the perspective of algorithms, we propose Spatial-aware Multi-Aspect Debiasing (SMAD), which incorporates both explicit debiasing with multi-aspect adversarial training and implicit debiasing with the spatial actionness reweighting module, to learn a more generic representation invariant to non-action aspects. To neutralize the intrinsic dataset bias, we propose OmniDebias to leverage web data for joint training selectively, which can achieve higher performance with far fewer web data. To verify the effectiveness, we establish evaluation protocols and perform extensive experiments on both re-distributed splits of existing datasets and a new evaluation dataset focusing on the action with rare scenes. We also show that the debiased representation can generalize better when transferred to other datasets and tasks.
更多查看译文
关键词
action recognition,representation,bias
AI 理解论文
溯源树
样例
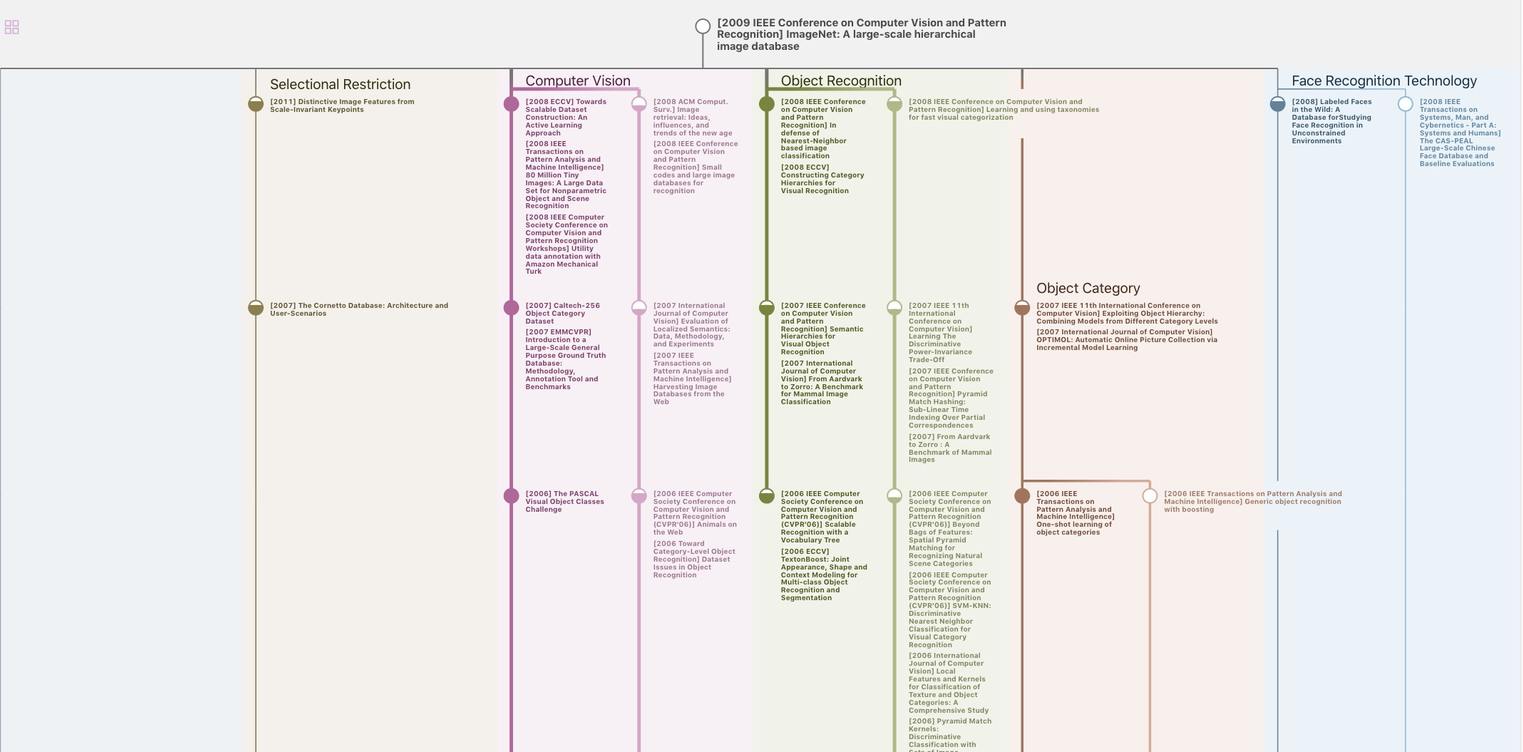
生成溯源树,研究论文发展脉络
Chat Paper
正在生成论文摘要