F*** workflows: when parts of FAIR are missing
2022 IEEE 18th International Conference on e-Science (e-Science)(2022)
摘要
The FAIR principles for scientific data (Findable, Accessible, Interoperable, Reusable) are also relevant to other digital objects such as research software and scientific workflows that operate on scientific data. The FAIR principles can be applied to the data being handled by a scientific workflow as well as the processes, software, and other infrastructure which are necessary to specify and execute a workflow. The FAIR principles were designed as guidelines, rather than rules, that would allow for differences in standards for different communities and for different degrees of compliance. There are many practical considerations which impact the level of FAIR-ness that can actually be achieved, including policies, traditions, and technologies. Because of these considerations, obstacles are often encountered during the workflow lifecycle that trace directly to shortcomings in the implementation of the FAIR principles. Here, we detail some cases, without naming names, in which data and workflows were Findable but otherwise lacking in areas commonly needed and expected by modern FAIR methods, tools, and users. We describe how some of these problems, all of which were overcome successfully, have motivated us to push on systems and approaches for fully FAIR workflows.
更多查看译文
关键词
data science,FAIR principles,high performance computing,workflows
AI 理解论文
溯源树
样例
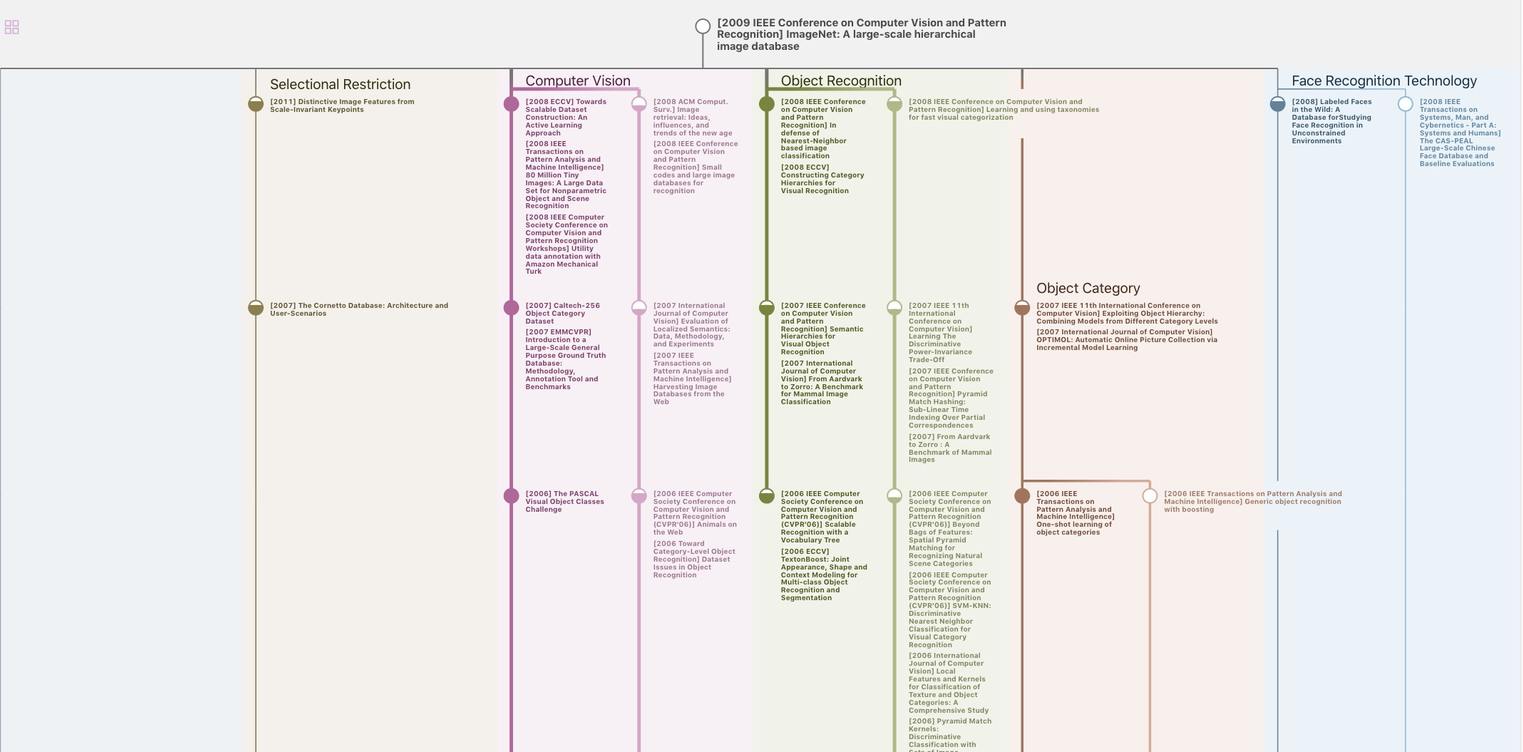
生成溯源树,研究论文发展脉络
Chat Paper
正在生成论文摘要