Differential Refinement Network for Zero-Shot Learning
IEEE TRANSACTIONS ON NEURAL NETWORKS AND LEARNING SYSTEMS(2024)
摘要
Zero-shot learning (ZSL) aims to recognize novel categories by merely utilizing disjoint seen samples. It is a challenging task as the knowledge of unseen objects is forbidden in the training stage, which easily leads to unseen samples degrading to mismatched categories. In order to alleviate the biased recognition problem, in this article, we propose a differential refinement network (DRNet) for ZSL, which aims to explore robust semantic-to-visual embedding. Our DRNet model consists of two subnetworks: basic network and differential network. The basic network targets to generate initial class-specific visual centers conditioned on corresponding semantic prototypes. The differential network is designed to predict class-unrelated differences between visual centers of arbitrary semantic prototype pairs, which are applied to further polish the initial visual centers. The motivation is that, by comparing different prototypes, interactions between various categories will be characterized, benefiting the generation of authentic and discriminative visual centers. Moreover, a modified episode-based training paradigm is explored to optimize the two subnetworks actively. In the training stage, we form a collection of episodes, each of which is an imitated ZSL task. Our DRNet is optimized by those sampled tasks rather than individual samples, which progressively learns skills to adapt and generalize to novel classes. Experiments on four challenging datasets demonstrate the effectiveness of our method.
更多查看译文
关键词
Visualization,Semantics,Prototypes,Training,Task analysis,Generative adversarial networks,Adaptation models,Basic network,differential network,episode-based learning,zero-shot learning (ZSL)
AI 理解论文
溯源树
样例
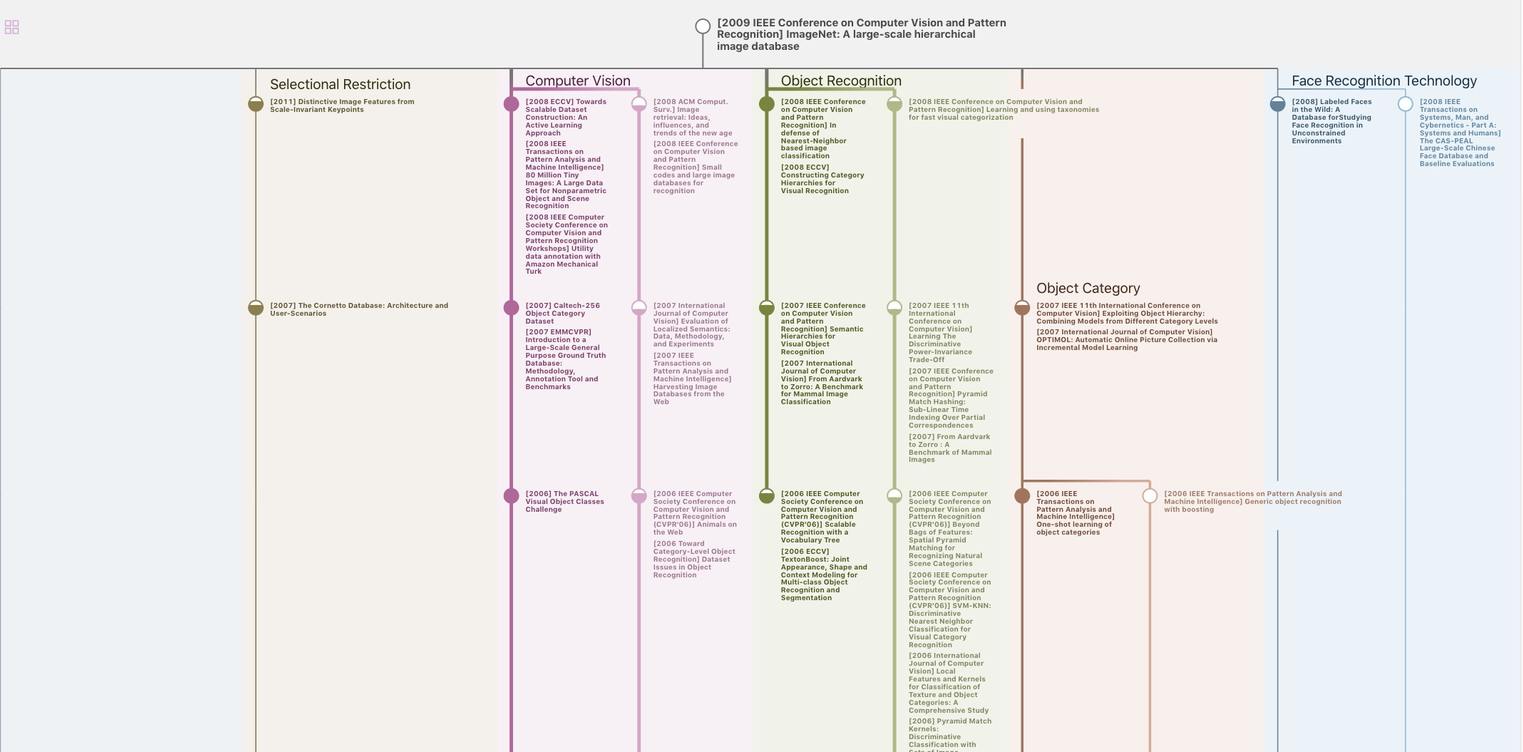
生成溯源树,研究论文发展脉络
Chat Paper
正在生成论文摘要