Lost in Translation: Reimagining the Machine Learning Life Cycle in Education
arxiv(2022)
摘要
Machine learning (ML) techniques are increasingly prevalent in education, from their use in predicting student dropout, to assisting in university admissions, and facilitating the rise of MOOCs. Given the rapid growth of these novel uses, there is a pressing need to investigate how ML techniques support long-standing education principles and goals. In this work, we shed light on this complex landscape drawing on qualitative insights from interviews with education experts. These interviews comprise in-depth evaluations of ML for education (ML4Ed) papers published in preeminent applied ML conferences over the past decade. Our central research goal is to critically examine how the stated or implied education and societal objectives of these papers are aligned with the ML problems they tackle. That is, to what extent does the technical problem formulation, objectives, approach, and interpretation of results align with the education problem at hand. We find that a cross-disciplinary gap exists and is particularly salient in two parts of the ML life cycle: the formulation of an ML problem from education goals and the translation of predictions to interventions. We use these insights to propose an extended ML life cycle, which may also apply to the use of ML in other domains. Our work joins a growing number of meta-analytical studies across education and ML research, as well as critical analyses of the societal impact of ML. Specifically, it fills a gap between the prevailing technical understanding of machine learning and the perspective of education researchers working with students and in policy.
更多查看译文
关键词
machine learning life cycle,education,life cycle,translation
AI 理解论文
溯源树
样例
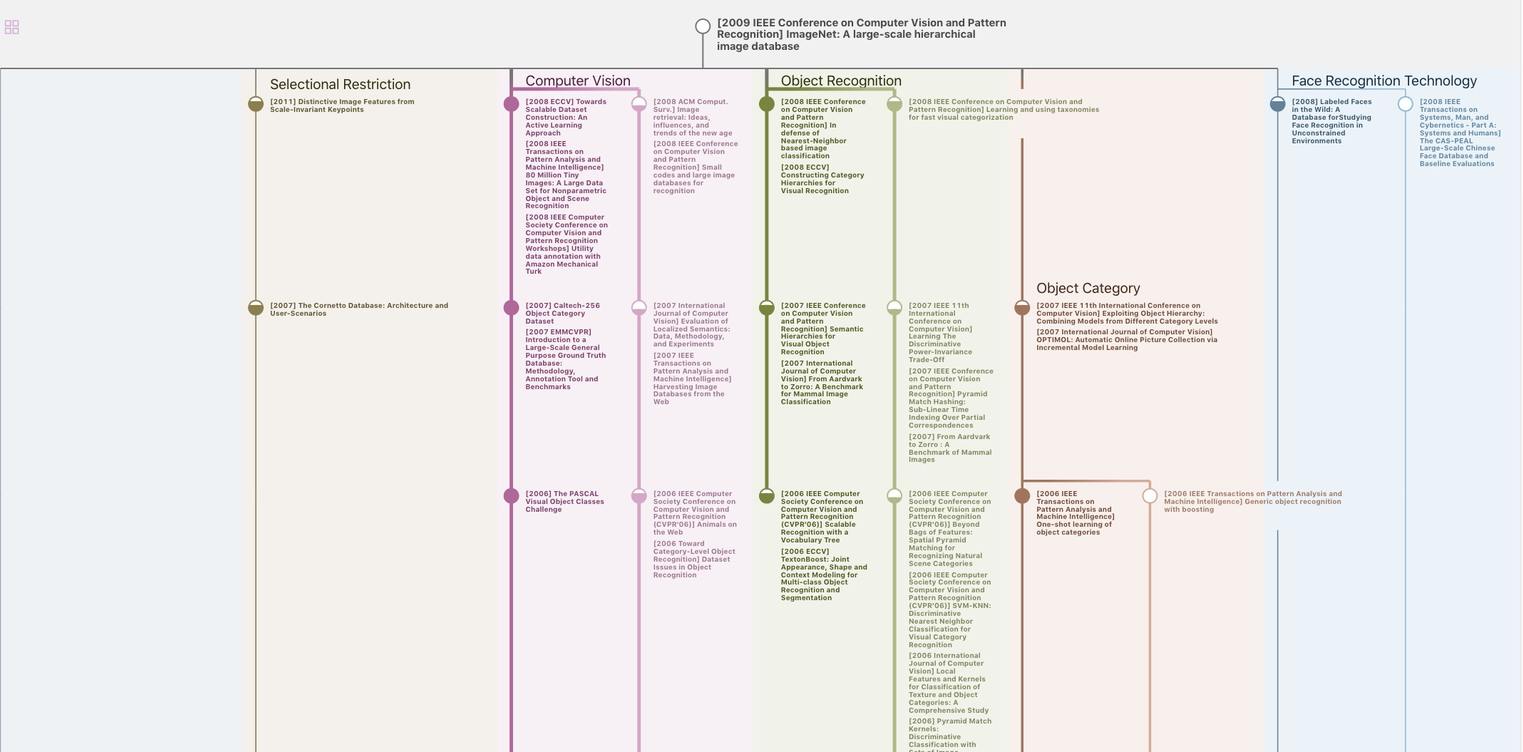
生成溯源树,研究论文发展脉络
Chat Paper
正在生成论文摘要