Consistency-Based Semi-supervised Evidential Active Learning for Diagnostic Radiograph Classification
MEDICAL IMAGE COMPUTING AND COMPUTER ASSISTED INTERVENTION, MICCAI 2022, PT I(2022)
摘要
Deep learning approaches achieve state-of-the-art performance for classifying radiology images, but rely on large labelled datasets that require resource-intensive annotation by specialists. Both semi-supervised learning and active learning can be utilised to mitigate this annotation burden. However, there is limited work on combining the advantages of semi-supervised and active learning approaches for multi-label medical image classification. Here, we introduce a novel Consistency-based Semi-supervised Evidential Active Learning framework (CSEAL). Specifically, we leverage predictive uncertainty based on theories of evidence and subjective logic to develop an end-to-end integrated approach that combines consistency-based semi-supervised learning with uncertainty-based active learning. We apply our approach to enhance four leading consistency-based semi-supervised learning methods: Pseudo-labelling, Virtual Adversarial Training, Mean Teacher and No Teacher. Extensive evaluations on multi-label Chest X-Ray classification tasks demonstrate that CSEAL achieves substantive performance improvements over two leading semi-supervised active learning baselines. Further, a class-wise breakdown of results shows that our approach can substantially improve accuracy on rarer abnormalities with fewer labelled samples.
更多查看译文
关键词
Semi-supervised learning, Active learning, Theory of evidence, Subjective logic, Multi-label classification
AI 理解论文
溯源树
样例
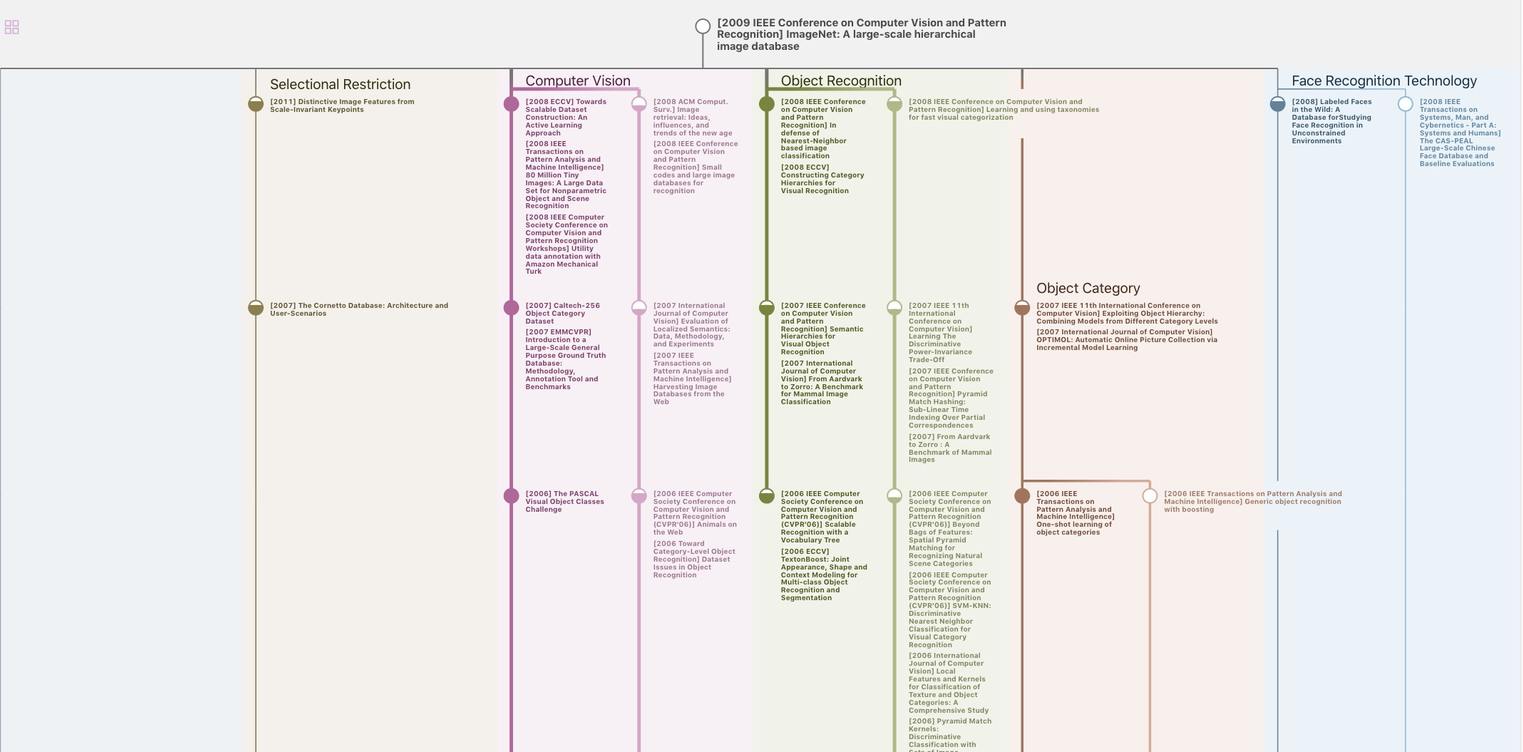
生成溯源树,研究论文发展脉络
Chat Paper
正在生成论文摘要