Novel split quality measures for stratified multilabel cross validation with application to large and sparse gene ontology datasets
Applied Computing and Intelligence(2022)
摘要
Multilabel learning is an important topic in machine learning research. Evaluating models in multilabel settings requires specific cross validation methods designed for multilabel data. In this article, we show that the most widely used cross validation split quality measure does not behave adequately with multilabel data that has strong class imbalance. We present improved measures and an algorithm, optisplit, for optimizing cross validations splits. Extensive comparison of various types of cross validation methods shows that optisplit produces more even cross validation splits than the existing methods and it is among the fastest methods with good splitting performance.
查看译文
关键词
stratified multilabel,quality measures,gene,validation,datasets
AI 理解论文
溯源树
样例
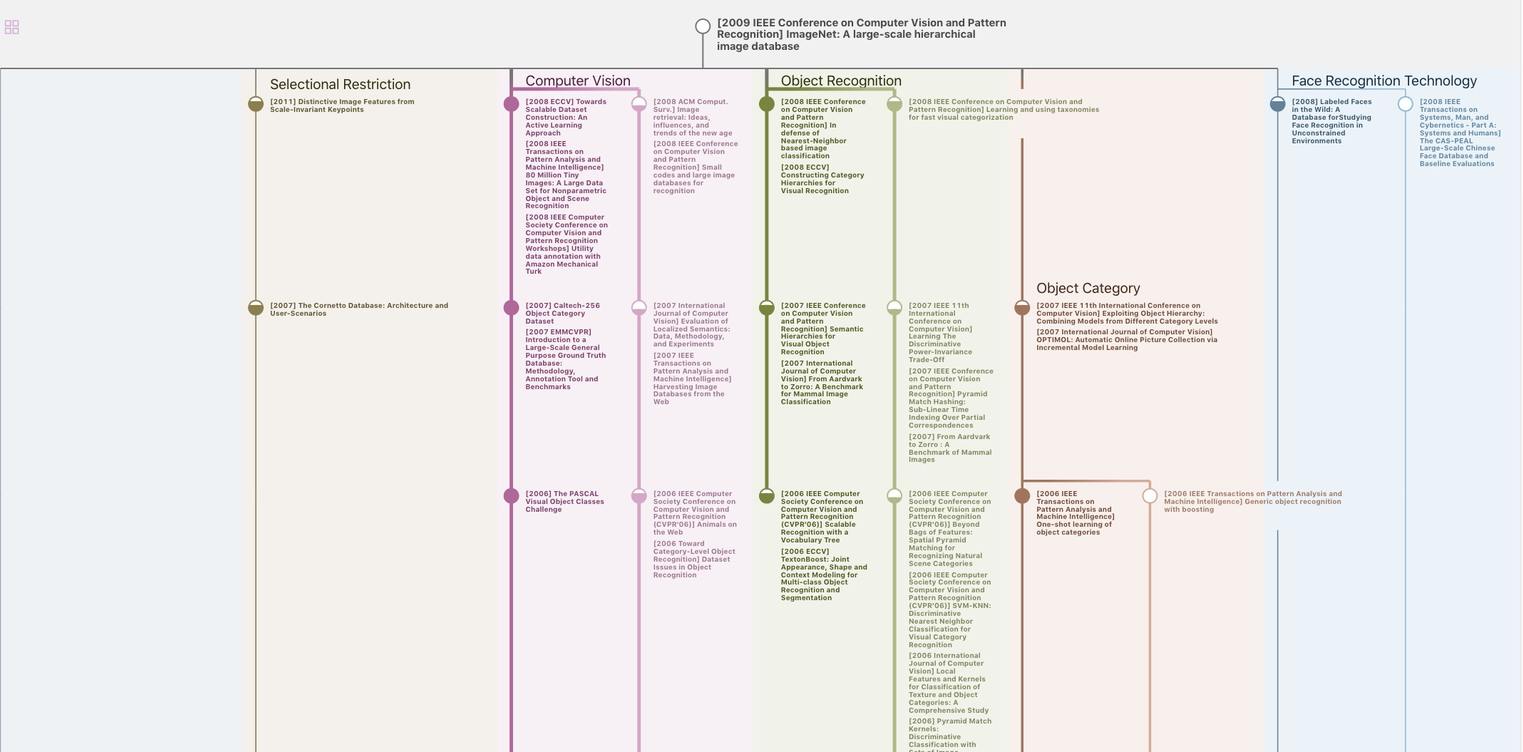
生成溯源树,研究论文发展脉络
Chat Paper
正在生成论文摘要