DETERRENT: Detecting Trojans Using Reinforcement Learning
IEEE TRANSACTIONS ON COMPUTER-AIDED DESIGN OF INTEGRATED CIRCUITS AND SYSTEMS(2024)
摘要
The globalized nature of the integrated circuits supply chain has given rise to several security problems. The insertion of malicious components, called hardware Trojans, is one such serious problem. Since Trojans are activated only under extremely rare trigger conditions and the search space is exponentially large, detecting them is arduous. Researchers have attempted to detect Trojans by querying the design-under-test using appropriate test patterns and monitoring its logical or side-channel response. However, techniques in both these categories lack either in terms of detection accuracy or scalability for larger designs. In this work, we investigate why existing techniques fall short and use our findings to propose a new reinforcement learning (RL) framework for detecting Trojans. We carefully design two RL agents (one for each category) that navigate the exponential search space of the test patterns and return minimal sets of patterns that are most likely to detect Trojans. We overcome challenges related to scalability and efficacy through appropriate solutions. Experimental results on a variety of benchmarks demonstrate the scalability and efficacy of our RL agents, which reduce the number of test patterns significantly (169.68x and 34.73x on average overall and 27.59x and 3.72x on average over large benchmarks) while maintaining or improving the Trojan-detection success rate compared to the state-of-the-art techniques.
更多查看译文
关键词
Trojan horses,Benchmark testing,Test pattern generators,Hardware,Logic testing,Switches,Scalability,Hardware Trojans,logic testing,reinforcement learning (RL),side-channel analysis,test generation
AI 理解论文
溯源树
样例
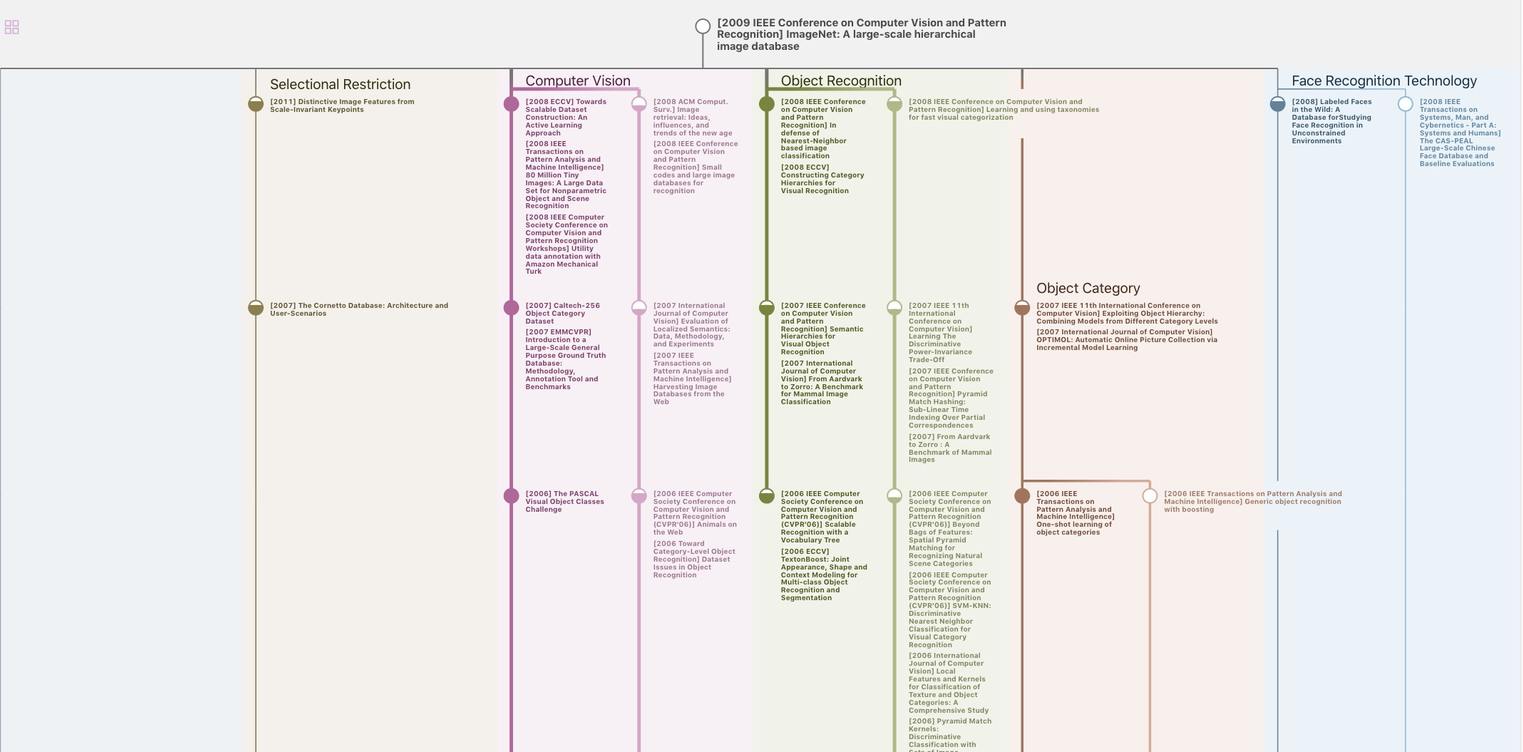
生成溯源树,研究论文发展脉络
Chat Paper
正在生成论文摘要