DPAUC: Differentially Private AUC Computation in Federated Learning
arxiv(2022)
摘要
Federated learning (FL) has gained significant attention recently as a privacy-enhancing tool to jointly train a machine learning model by multiple participants. The prior work on FL has mostly studied how to protect label privacy during model training. However, model evaluation in FL might also lead to potential leakage of private label information. In this work, we propose an evaluation algorithm that can accurately compute the widely used AUC (area under the curve) metric when using the label differential privacy (DP) in FL. Through extensive experiments, we show our algorithms can compute accurate AUCs compared to the ground truth. The code is available at {\url{https://github.com/bytedance/fedlearner/tree/master/example/privacy/DPAUC}}.
更多查看译文
关键词
private dpauc computation,learning
AI 理解论文
溯源树
样例
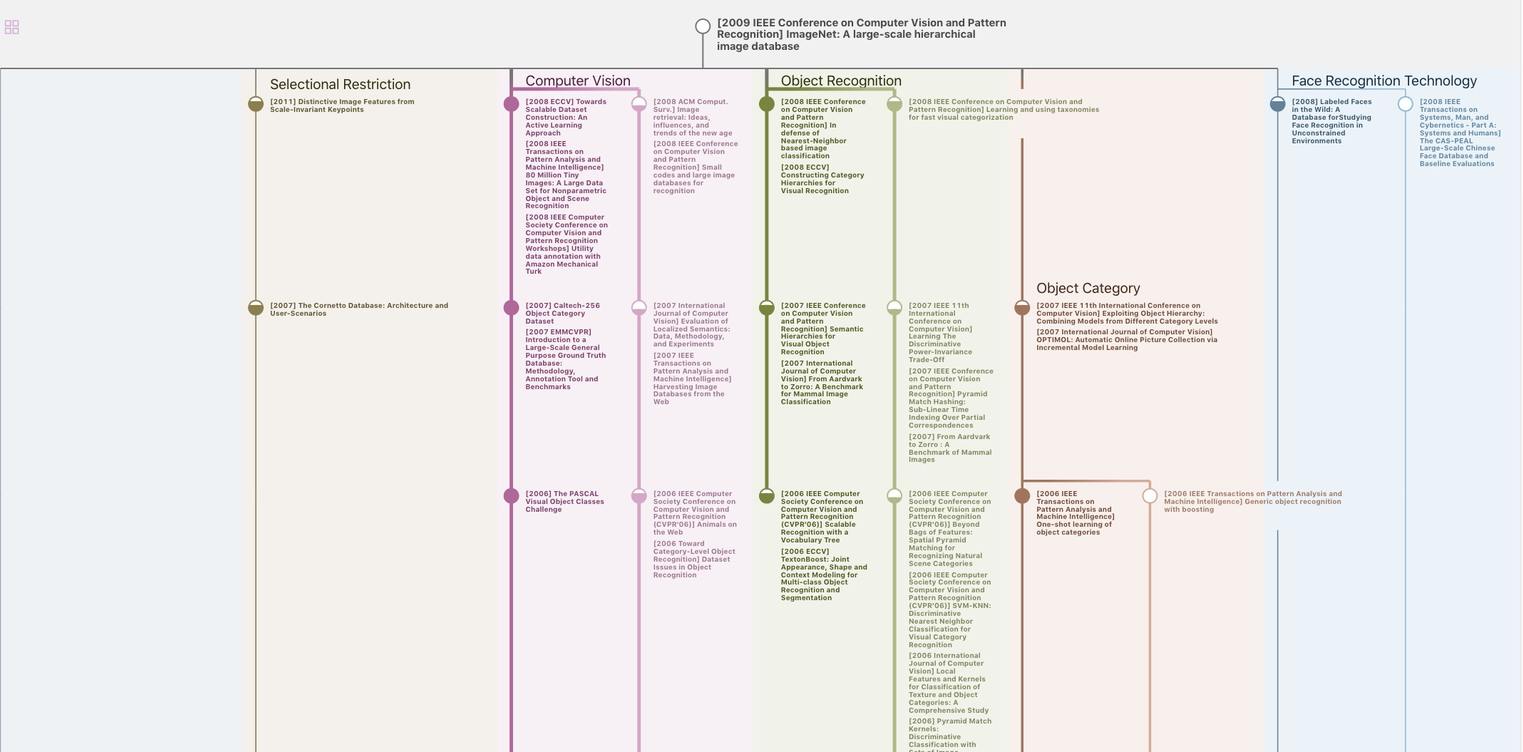
生成溯源树,研究论文发展脉络
Chat Paper
正在生成论文摘要