Anytime-Lidar: Deadline-aware 3D Object Detection
2022 IEEE 28th International Conference on Embedded and Real-Time Computing Systems and Applications (RTCSA)(2022)
摘要
In this work, we present a novel scheduling frame-work enabling anytime perception for deep neural network (DNN) based 3D object detection pipelines. We focus on computationally expensive region proposal network (RPN) and per-category multi-head detector components, which are common in 3D object detection pipelines, and make them deadline-aware. We propose a scheduling algorithm, which intelligently selects the subset of the components to make effective time and accuracy trade-off on the fly. We minimize accuracy loss of skipping some of the neural network sub-components by projecting previously detected objects onto the current scene through estimations. We apply our approach to a state-of-art 3D object detection network, PointPillars, and evaluate its performance on Jetson Xavier AGX using nuScenes dataset. Compared to the baselines, our approach significantly improve the network’s accuracy under various deadline constraints.
更多查看译文
关键词
Lidar,3d object detection,PointPillars,Anytime computing
AI 理解论文
溯源树
样例
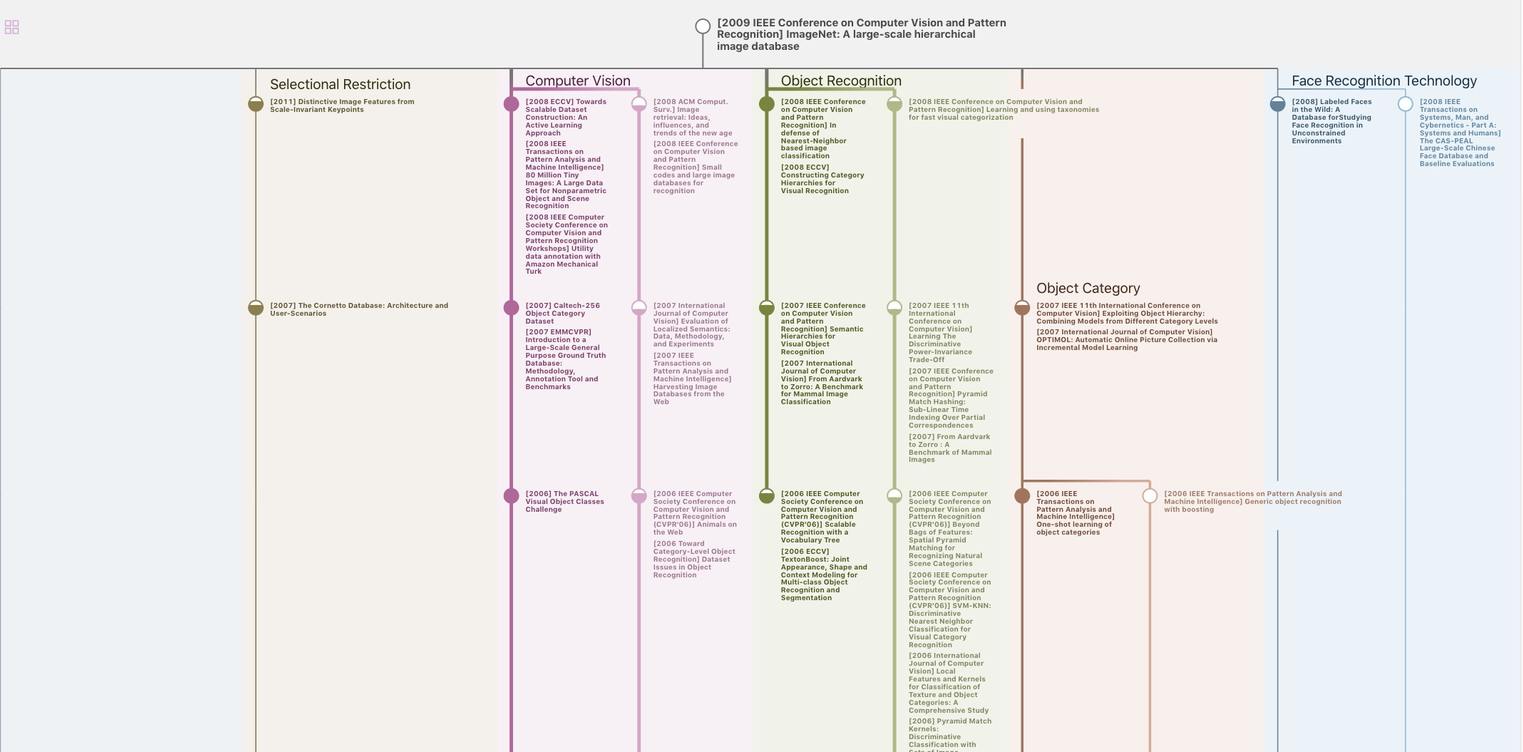
生成溯源树,研究论文发展脉络
Chat Paper
正在生成论文摘要