Learning Task-Oriented Flows to Mutually Guide Feature Alignment in Synthesized and Real Video Denoising
arxiv(2023)
摘要
Video denoising aims at removing noise from videos to recover clean ones. Some existing works show that optical flow can help the denoising by exploiting the additional spatial-temporal clues from nearby frames. However, the flow estimation itself is also sensitive to noise, and can be unusable under large noise levels. To this end, we propose a new multi-scale refined optical flow-guided video denoising method, which is more robust to different noise levels. Our method mainly consists of a denoising-oriented flow refinement (DFR) module and a flow-guided mutual denoising propagation (FMDP) module. Unlike previous works that directly use off-the-shelf flow solutions, DFR first learns robust multi-scale optical flows, and FMDP makes use of the flow guidance by progressively introducing and refining more flow information from low resolution to high resolution. Together with real noise degradation synthesis, the proposed multi-scale flow-guided denoising network achieves state-of-the-art performance on both synthetic Gaussian denoising and real video denoising. The codes will be made publicly available.
更多查看译文
关键词
mutually guide feature alignment,learning,video,task-oriented
AI 理解论文
溯源树
样例
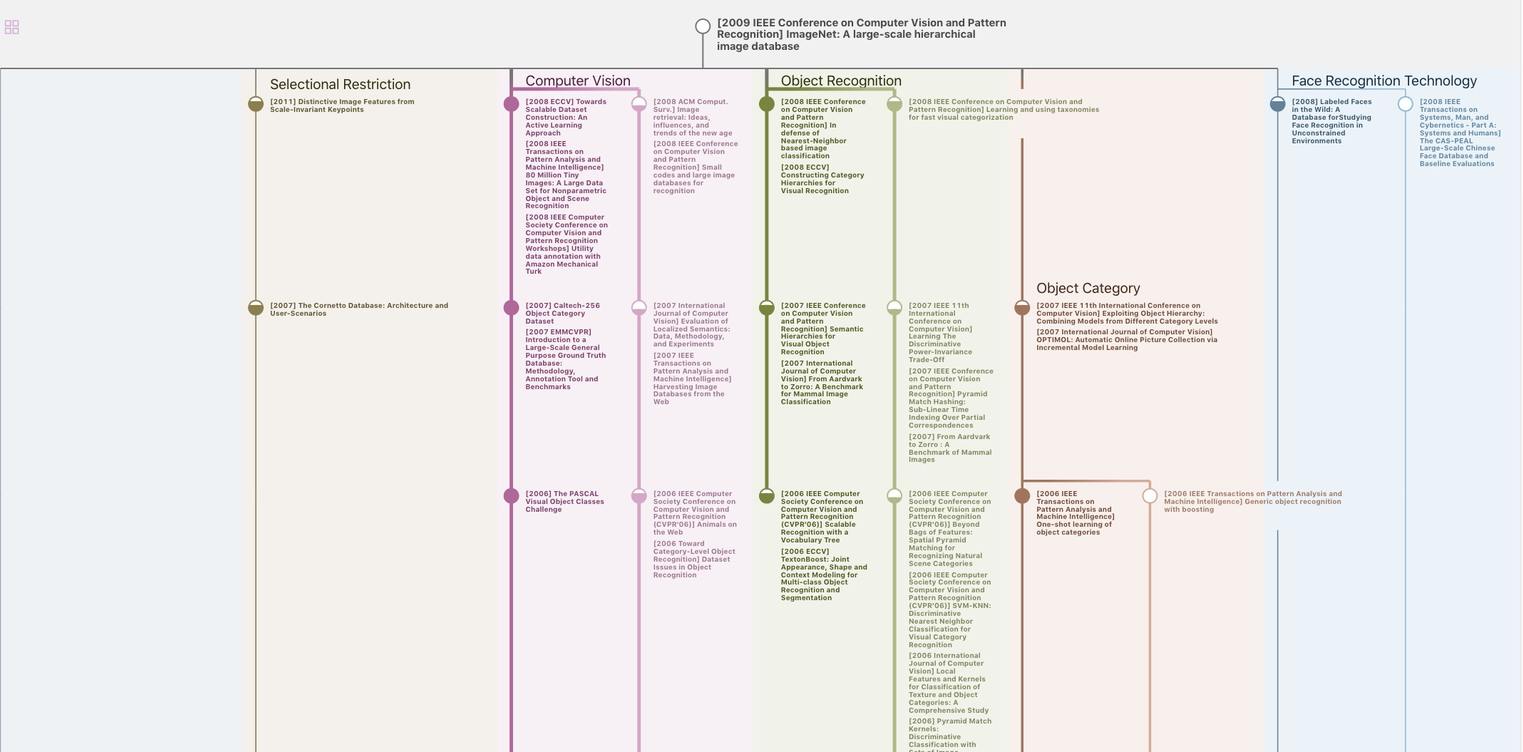
生成溯源树,研究论文发展脉络
Chat Paper
正在生成论文摘要