Debiasing the Cloze Task in Sequential Recommendation with Bidirectional Transformers
KDD '22: Proceedings of the 28th ACM SIGKDD Conference on Knowledge Discovery and Data Mining(2022)
摘要
Bidirectional Transformer architectures are state-of-the-art sequential recommendation models that use a bi-directional representation capacity based on the Cloze task, a.k.a. Masked Language Modeling. The latter aims to predict randomly masked items within the sequence. Because they assume that the true interacted item is the most relevant one, an exposure bias results, where non-interacted items with low exposure propensities are assumed to be irrelevant. The most common approach to mitigating exposure bias in recommendation has been Inverse Propensity Scoring (IPS), which consists of down-weighting the interacted predictions in the loss function in proportion to their propensities of exposure, yielding a theoretically unbiased learning. In this work, we argue and prove that IPS does not extend to sequential recommendation because it fails to account for the temporal nature of the problem. We then propose a novel propensity scoring mechanism, which can theoretically debias the Cloze task in sequential recommendation. Finally we empirically demonstrate the debiasing capabilities of our proposed approach and its robustness to the severity of exposure bias.
更多查看译文
关键词
sequential recommendation,cloze task
AI 理解论文
溯源树
样例
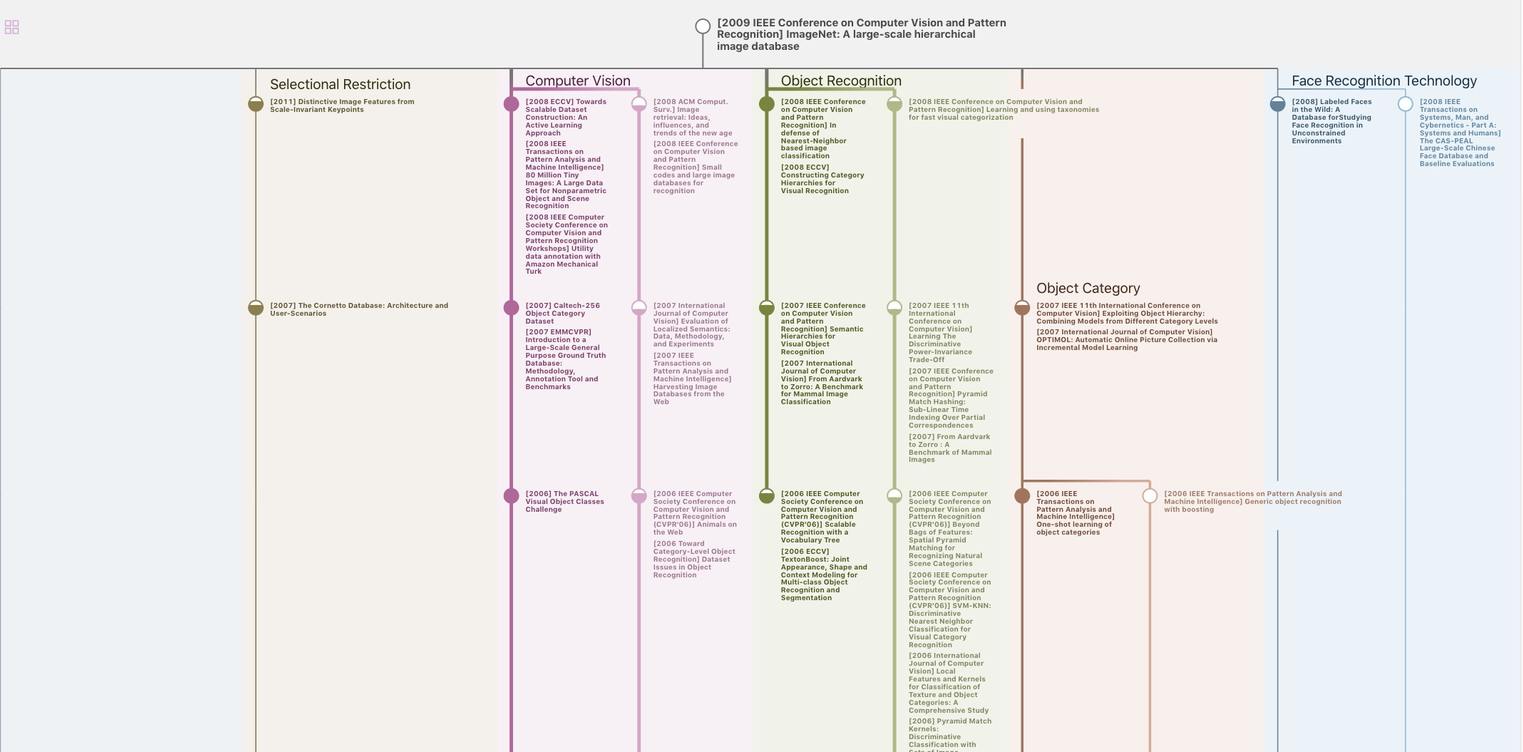
生成溯源树,研究论文发展脉络
Chat Paper
正在生成论文摘要