Vexation-Aware Active Learning for On-Menu Restaurant Dish Availability
KDD '22: Proceedings of the 28th ACM SIGKDD Conference on Knowledge Discovery and Data Mining(2022)
摘要
Here we leverage the power of the crowd: online users who are willing to answer questions about dish availability at restaurants visited. While motivated users are happy to contribute knowledge, they are much less likely to respond to "silly'' or embarrassing questions (e.g., "DoesPizza Hut serve pizza?'' or "DoesMike's Vegan Restaurant serve steak?'') In this paper, we study the problem of Vexation-Aware Active Learning (VAAL), where judiciously selected questions are targeted towards improving restaurant-dish model prediction, subject to a limit on the percentage of "unsure'' answers or "dismissals'' (e.g., swiping the app closed) measuring user vexation. We formalize the selection problem as an integer program and solve it efficiently using a distributed solution that scales linearly with the number of candidate questions. Since our algorithm relies on an accurate estimation of the unsure-dismiss rate (UDR), we present a regression model that provides high-quality results compared to baselines including collaborative filtering. Finally, we demonstrate in a live system that our proposed VAAL strategy performs competitively against classical (margin-based) active learning approaches while reducing the UDR for the questions being asked.
更多查看译文
关键词
learning,vexation-aware,on-menu
AI 理解论文
溯源树
样例
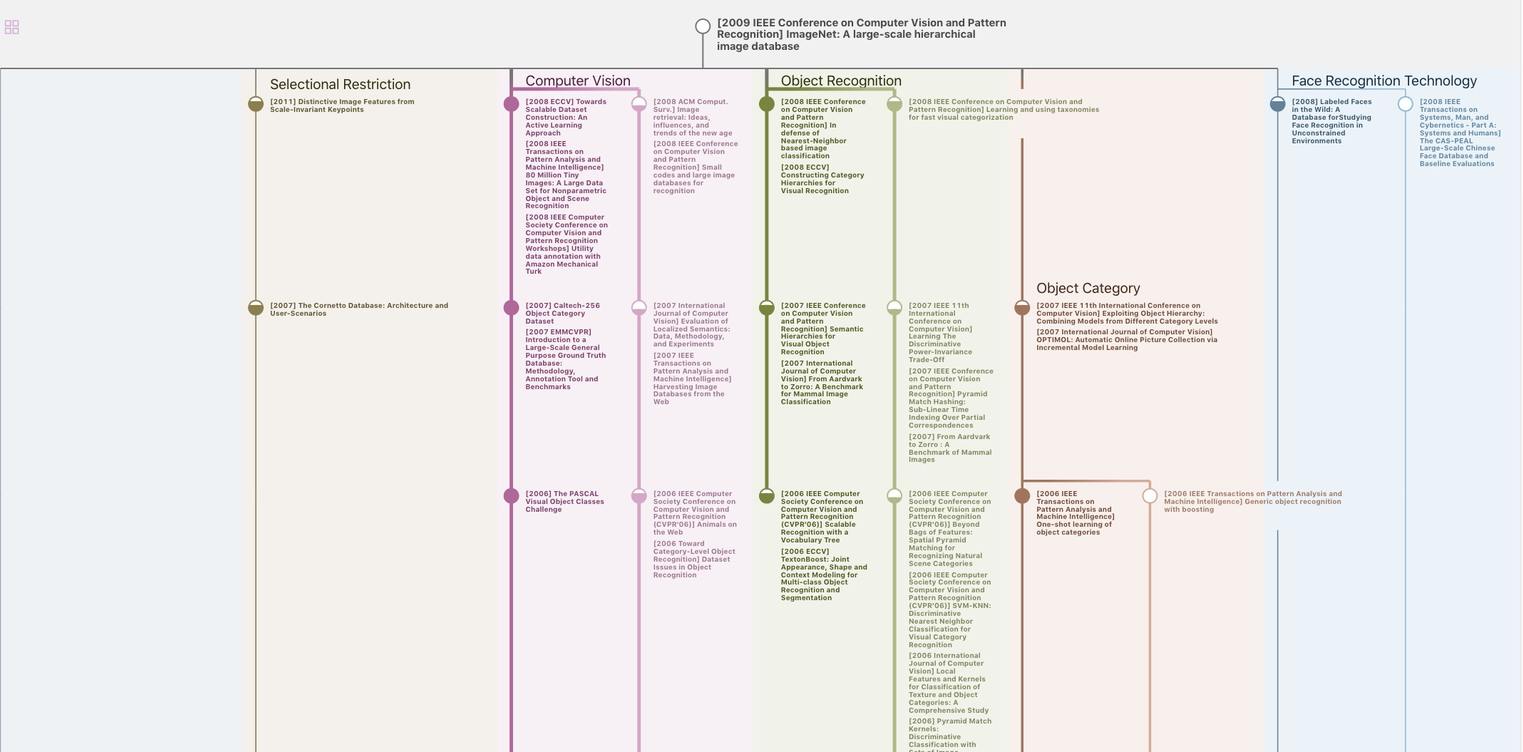
生成溯源树,研究论文发展脉络
Chat Paper
正在生成论文摘要