An Online Multi-task Learning Framework for Google Feed Ads Auction Models
KDD '22: Proceedings of the 28th ACM SIGKDD Conference on Knowledge Discovery and Data Mining(2022)
摘要
In this paper, we introduce a large scale online multi-task deep learning framework for modeling multiple feed ads auction prediction tasks on an industry-scale feed ads recommendation platform. Multiple prediction tasks are combined into one single model which is continuously trained on real time new ads data. Multi-tasking ads auction models in real-time faces many real-world challenges. For example, each task may be trained on different set of training data; the labels of different tasks may have different arrival time due to label delay; different tasks will interact with each other; combining the losses of each task is non-trivial. We tackle these challenges using practical and novel techniques such as multi-stage training for handling label delay, Multi-gate Mixture-of-Experts (MMoE) to optimize model interaction and an auto-parameter learning algorithm to optimize the loss weights of different tasks. We demonstrate that our proposed techniques can lead to quality improvements and substantial resource saving compared to modeling each single task independently.
更多查看译文
关键词
learning,feed,multi-task
AI 理解论文
溯源树
样例
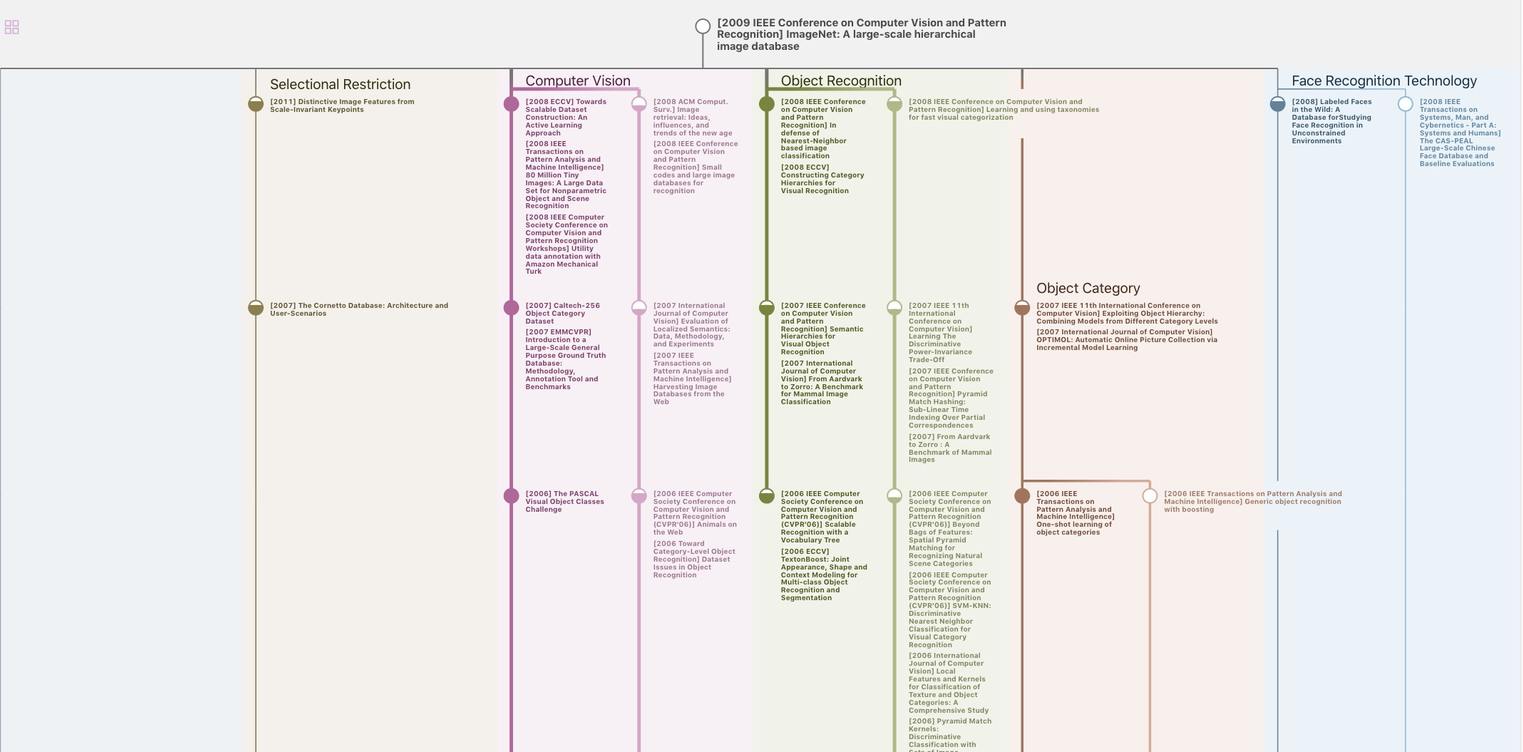
生成溯源树,研究论文发展脉络
Chat Paper
正在生成论文摘要