Learning to Pick by Digging: Data-Driven Dig-Grasping for Bin Picking from Clutter
2022 International Conference on Robotics and Automation (ICRA)(2022)
摘要
We present a data-driven approach for effective bin picking from clutter. Recent bin picking solutions usually lead to a direct pinch grasp on a target object without addressing any other potential contact interaction in clutter. However, appropriate physical interaction can be essential to successful singulation and subsequent secure picking, the goal of bin picking. In this work, we contribute a framework that learns physically interactive actions for object picking end-to-end from a visual input in a self-supervised manner. The learned actions enable the robot to purposefully interact with a target object by performing a digging operation through the clutter. By leveraging a fully convolutional network (FCN), we predict picking success probabilities for a set of interactive action primitives that will in turn specify an optimal action to perform. The FCN is trained in a simulated environment through trial and error. Moreover, new datasets are collected using the latest network through iterative self-supervision. Extensive real-world bin picking experiments show the effectiveness and generalizability of the approach.
更多查看译文
关键词
bin picking,data-driven,dig-grasping
AI 理解论文
溯源树
样例
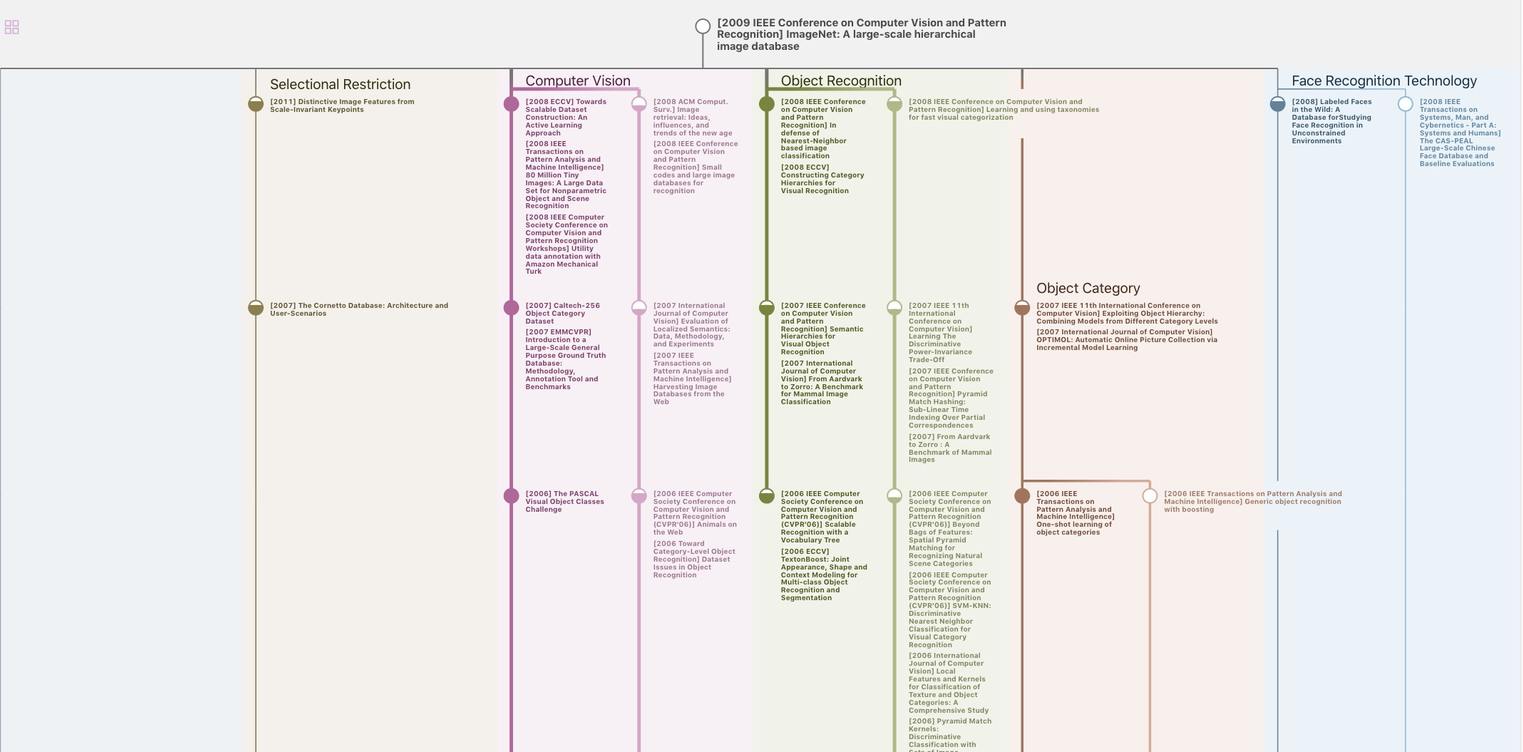
生成溯源树,研究论文发展脉络
Chat Paper
正在生成论文摘要