Online Performance and Power Prediction for Edge TPU via Comprehensive Characterization
PROCEEDINGS OF THE 2022 DESIGN, AUTOMATION & TEST IN EUROPE CONFERENCE & EXHIBITION (DATE 2022)(2022)
摘要
In this paper, we characterize and model the performance and power consumption of Edge TPU, which efficiently accelerates deep learning (DL) inference in a low-power environment. Systolic array, as a high throughput computation architecture, its usage in the edge excites our interest in its performance and power pattern. We perform an extensive study for various neural network settings and sizes using more than 10,000 DL models. Through comprehensive exploration, we profile which factors highly influence the inference time and power to run DL Models. We show our key remarks for the relation between the performance/power and DL model complexity to enable hardware-aware optimization and design decisions. For example, our measurement shows that energy/performance is not linearly-proportional to the number of MAC operations. In fact, as the computation and DL model size increase, the performance follows a stepped pattern. Hence, the accurate estimate should consider other features of DL models such as on-chip/off-chip memory usages. Based on the characterization, we propose a modeling framework, called PETET, which perform online predictions for the performance and power of Edge TPU. The proposed method automatically identifies the relationship of the performance, power, and memory usages to the DL model settings based on machine learning techniques.
更多查看译文
关键词
power prediction,Edge TPU,comprehensive characterization,deep learning inference,low-power environment,systolic array,high throughput computation architecture,power pattern,neural network,DL model complexity,hardware-aware optimization,PETET
AI 理解论文
溯源树
样例
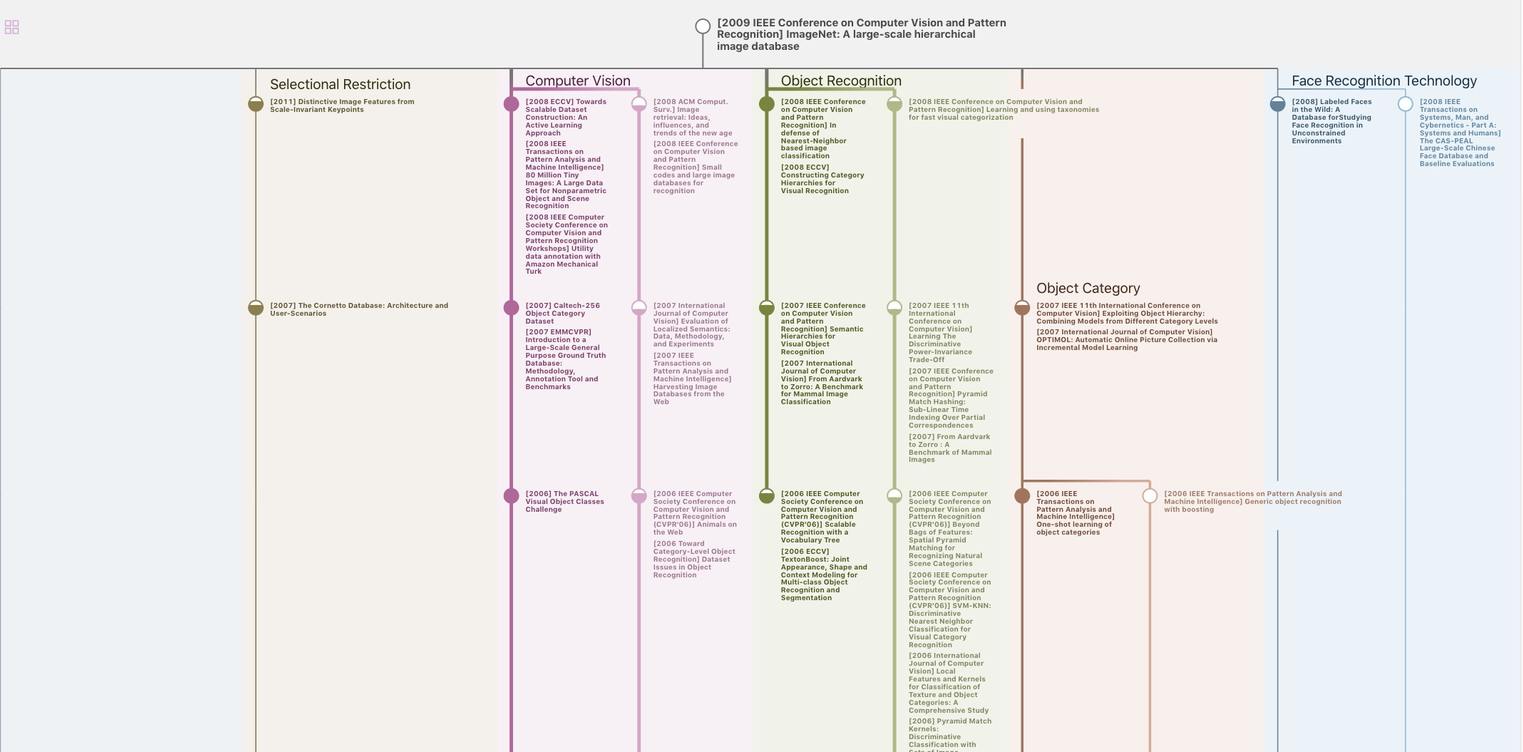
生成溯源树,研究论文发展脉络
Chat Paper
正在生成论文摘要