Learning Relationship-Based Access Control Policies from Black-Box Systems
ACM TRANSACTIONS ON PRIVACY AND SECURITY(2022)
摘要
Access control policies are crucial in securing data in information systems. Unfortunately, often times, such policies are poorly documented, and gaps between their specification and implementation prevent the system users, and even its developers, from understanding the overall enforced policy of a system. To tackle this problem, we propose the first of its kind systematic approach for learning the enforced authorizations from a target system by interacting with and observing it as a black box. The black-box view of the target system provides the advantage of learning its overall access control policy without dealing with its internal design complexities. Furthermore, compared to the previous literature on policy mining and policy inference, we avoid exhaustive exploration of the authorization space by minimizing our observations. We focus on learning relationship-based access control (ReBAC) policy, and show how we can construct a deterministic finite automaton (DFA) to formally characterize such an enforced policy. We theoretically analyze our proposed learning approach by studying its termination, correctness, and complexity. Furthermore, we conduct extensive experimental analysis based on realistic application scenarios to establish its cost, quality of learning, and scalability in practice.
更多查看译文
关键词
Relationship-based access control, black box, model learning, formal analysis
AI 理解论文
溯源树
样例
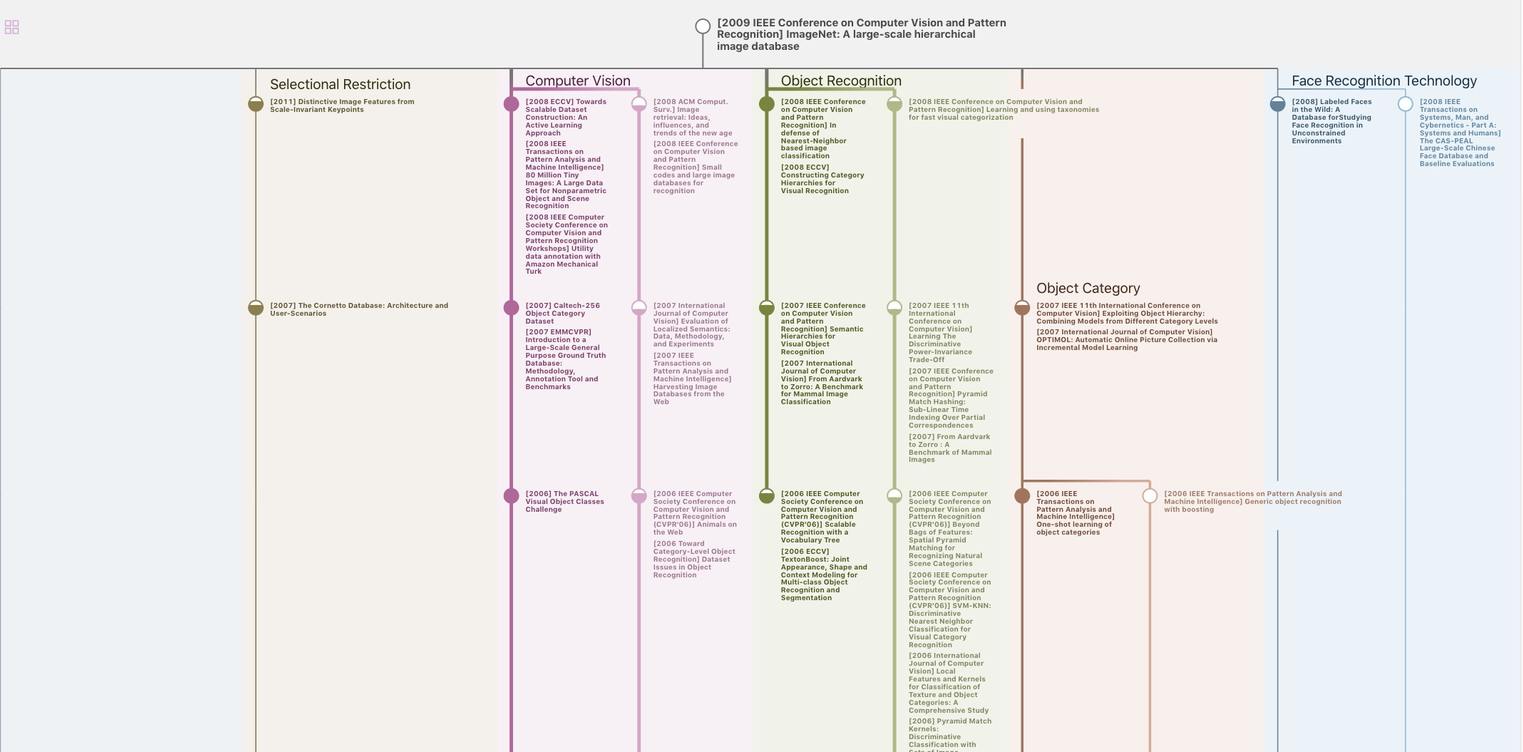
生成溯源树,研究论文发展脉络
Chat Paper
正在生成论文摘要