Arm: Efficient Learning of Neural Retrieval Models with Desired Accuracy by Automatic Knowledge Amalgamation
SIGIR '22: Proceedings of the 45th International ACM SIGIR Conference on Research and Development in Information Retrieval(2022)
摘要
In recent years, there has been increasing interest in adopting published neural retrieval models learned from corpora for text retrieval. Although these models achieve excellent retrieval performance, in terms of popular accuracy metrics, on datasets they have been trained, their performance on new text data might degrade. To obtain the desired retrieval performance on both the data used in training and the latest data collected after training, the simple approach of learning a new model from both datasets is not always feasible since the annotated dataset used in training is often not published along with the learned model. Knowledge amalgamation (KA) is an emerging technique to deal with this problem of inaccessibility of data used in previous training. KA learns a new model (called a student model) from new data by reusing (called amalgamating) a number of trained models (called teacher models) instead of accessing the teachers' original training data. However, in order to efficiently learn an accurate student model, the classical KA approach requires manual selection of an appropriate subset of teacher models for amalgamation. This manual procedure for selecting teacher models prevents the classical KA from being scaled to retrieval tasks for which a large number of candidate teacher models are ready to be reused. This paper presents Arm, an intelligent system for efficiently learning a neural retrieval model with the desired accuracy on incoming data by automatically amalgamating a subset of teacher models (called a teacher model combination or simply combination ) among a large number of teacher models. o filter combinations that fail to produce accurate student models, Arm employs Bayesian optimization to derive an accuracy prediction model based on sampled amalgamation tasks. Then, Arm uses the derived prediction model to exclude unqualified combinations without training the rest combinations. To speed up training, Arm introduces a cost model that picks the teacher model combination with the minimal training cost among all qualified teacher model combinations to produce the final student model. This paper will demonstrate the major workflow of Arm and present the produced student models to users.
更多查看译文
关键词
Neural Retrieval Model, Knowledge Amalgamation, Prediction Model, Cost Model
AI 理解论文
溯源树
样例
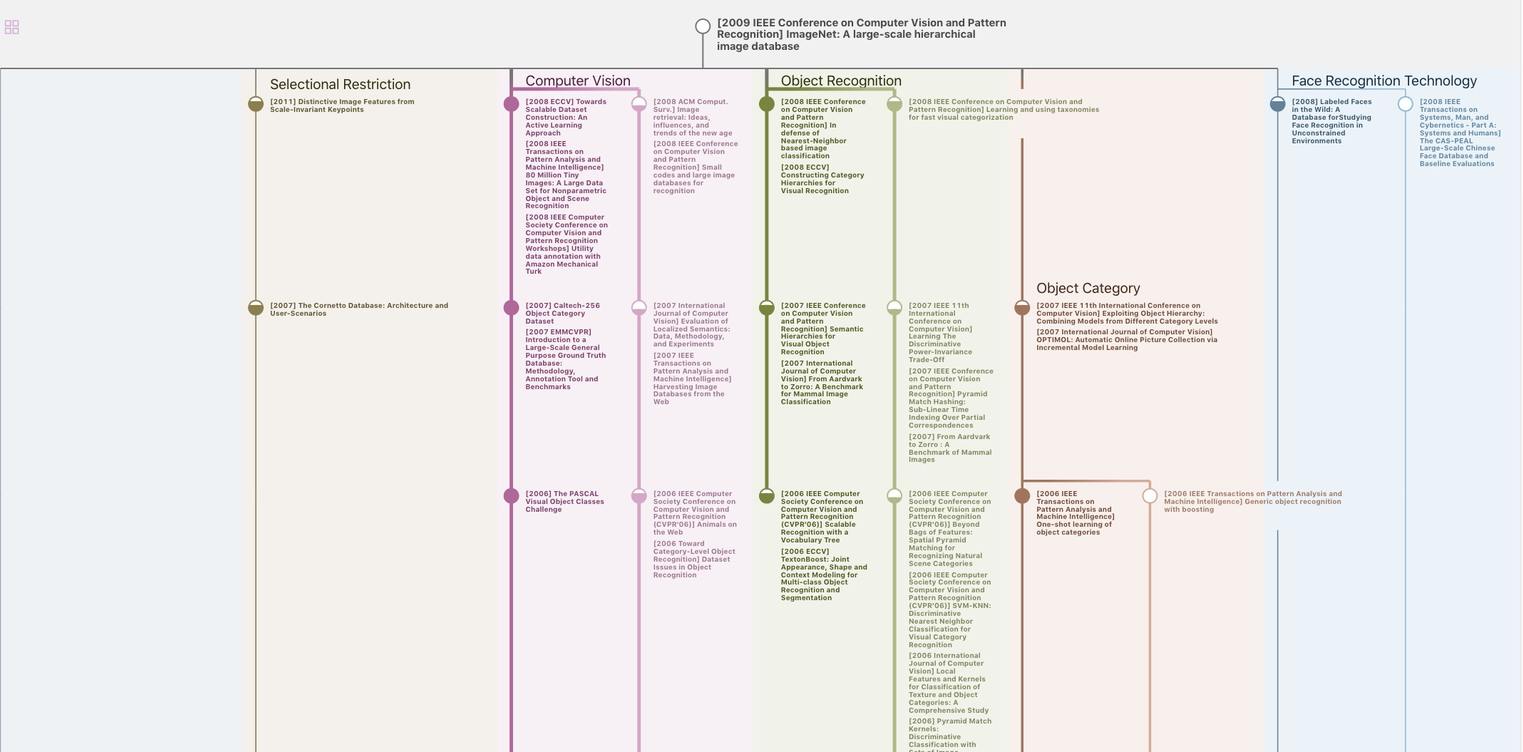
生成溯源树,研究论文发展脉络
Chat Paper
正在生成论文摘要