Ensemble learning for the partial label ranking problem
MATHEMATICAL METHODS IN THE APPLIED SCIENCES(2023)
摘要
The partial label ranking problem is a general interpretation of the preference learning scenario known as the label ranking problem, the goal of which is to learn preference classifiers able to predict a complete ranking with ties over the finite set of labels of the class variable. In this paper, we use unsupervised discretization techniques (equal-frequency and equal-width binning) to heuristically select the threshold for the numerical features in the algorithms based on induction of decision trees (partial label ranking trees algorithm). Moreover, we adapt the most well-known averaging (bootstrap aggregating and random forests) and boosting (adaptive boosting) ensemble methods to the partial label ranking problem, in order to improve the robustness of the built classifiers. We compare the proposed methods with the nearest neighbors-based algorithm (instance based partial label ranking) over the standard benchmark datasets, showing that our versions of the ensemble methods are superior in terms of accuracy. Furthermore, they are affordable in terms of computational efficiency.
更多查看译文
关键词
decision trees, ensembles, label ranking problem, partial label ranking problem, preference learning
AI 理解论文
溯源树
样例
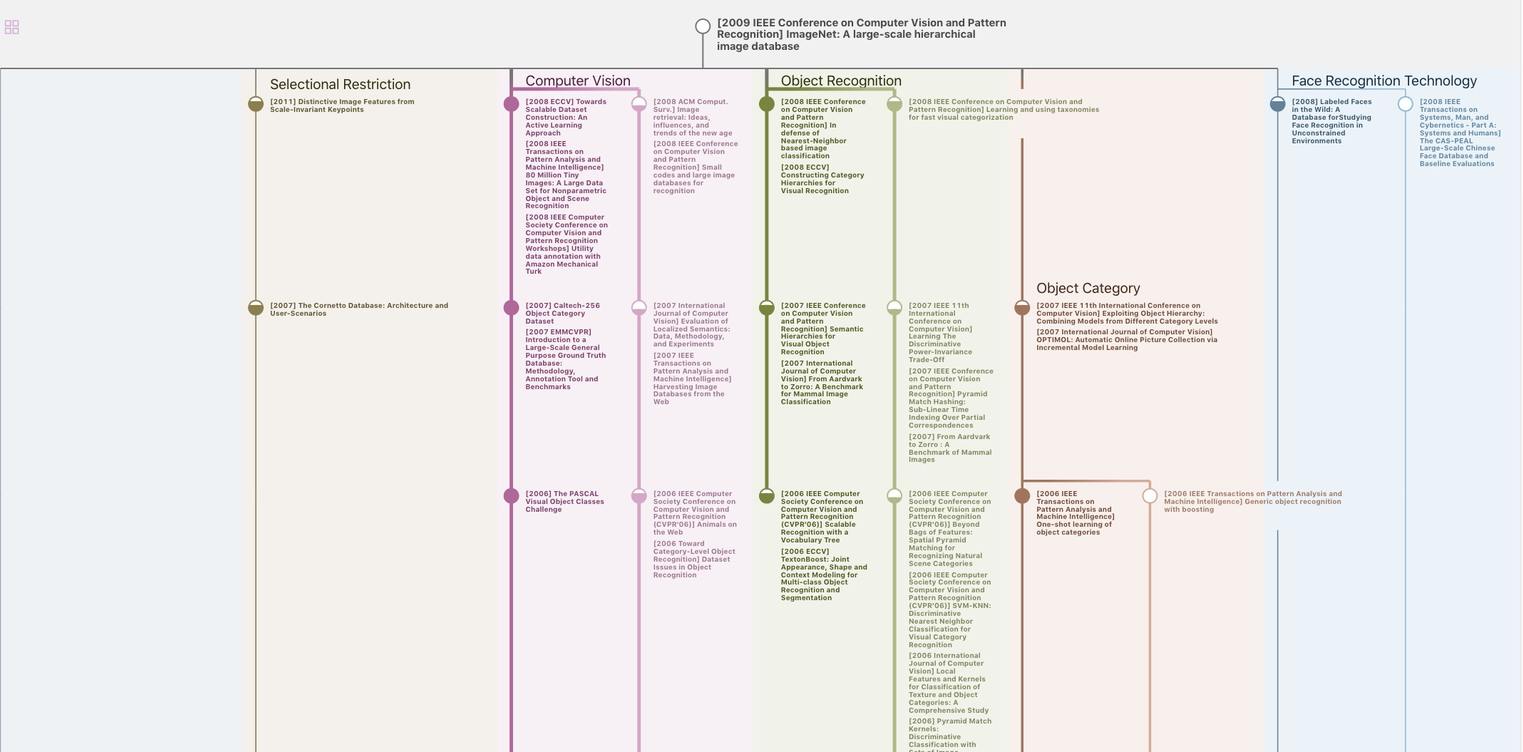
生成溯源树,研究论文发展脉络
Chat Paper
正在生成论文摘要