RASCL: a randomised approach to subspace clusters
International Journal of Data Science and Analytics(2022)
摘要
Subspace clustering aims to discover clusters in projections of highly dimensional numerical data. In this paper, we focus on discovering small collections of highly interesting subspace clusters that do not try to cluster all data points, leaving noisy data points unclustered. To this end, we propose a randomised method that first converts the highly dimensional database to a binarised one using projected samples of the original database. Subsequently, this database is mined for frequent itemsets, which we show can be translated back to subspace clusters. In this way, we are able to explore multiple subspaces of different sizes at the same time. In our extensive experimental analysis, we show on synthetic as well as real-world data that our method is capable of discovering highly interesting subspace clusters efficiently.
更多查看译文
关键词
Subspace clusters,High-dimensional data,Sampling,Maximal itemsets
AI 理解论文
溯源树
样例
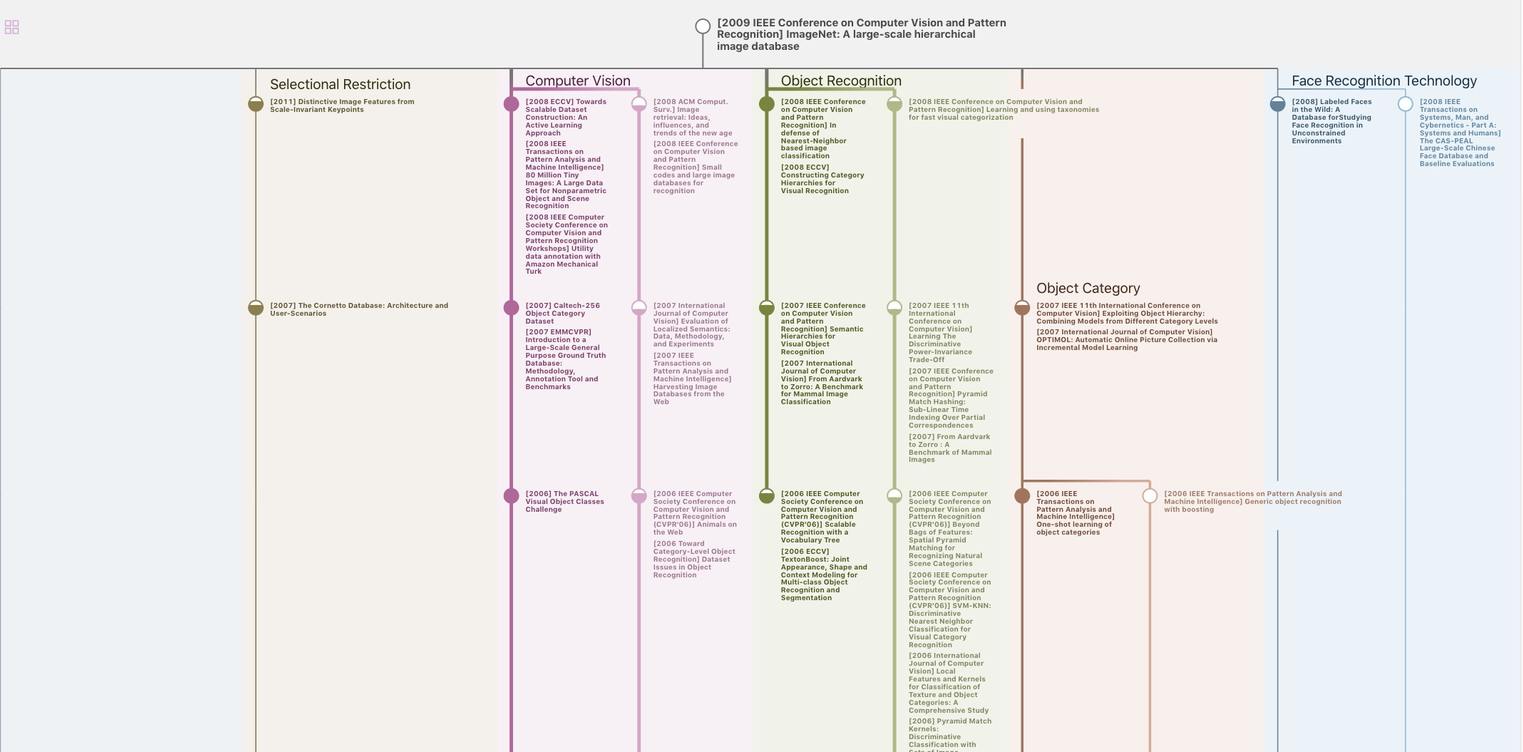
生成溯源树,研究论文发展脉络
Chat Paper
正在生成论文摘要