Optimizing Bipedal Maneuvers of Single Rigid-Body Models for Reinforcement Learning
2022 IEEE-RAS 21st International Conference on Humanoid Robots (Humanoids)(2022)
摘要
In this work, we propose a method to generate reduced-order model reference trajectories for general classes of highly dynamic maneuvers for bipedal robots for use in sim-to-real reinforcement learning. Our approach is to utilize a single rigid-body model (SRBM) to optimize libraries of trajectories offline to be used as expert references that guide learning by regularizing behaviors when incorporated in the reward function of a learned policy. This method translates the model's dynamically rich rotational and translational behavior to a full-order robot model and successfully transfers to real hardware. The SRBM's simplicity allows for fast iteration and refinement of behaviors, while the robustness of learning-based controllers allows for highly dynamic motions to be transferred to hardware. Within this work we introduce a set of transferability constraints that amend the SRBM dynamics to actual bipedal robot hardware, our framework for creating optimal trajectories for a variety of highly dynamic maneuvers as well as our approach to integrating reference trajectories for a high-speed running reinforcement learning policy. We validate our methods on the bipedal robot Cassie on which we were successfully able to demonstrate highly dynamic grounded running gaits up to 3.0 m/s.
更多查看译文
关键词
bipedal maneuvers,learning,models,rigid-body
AI 理解论文
溯源树
样例
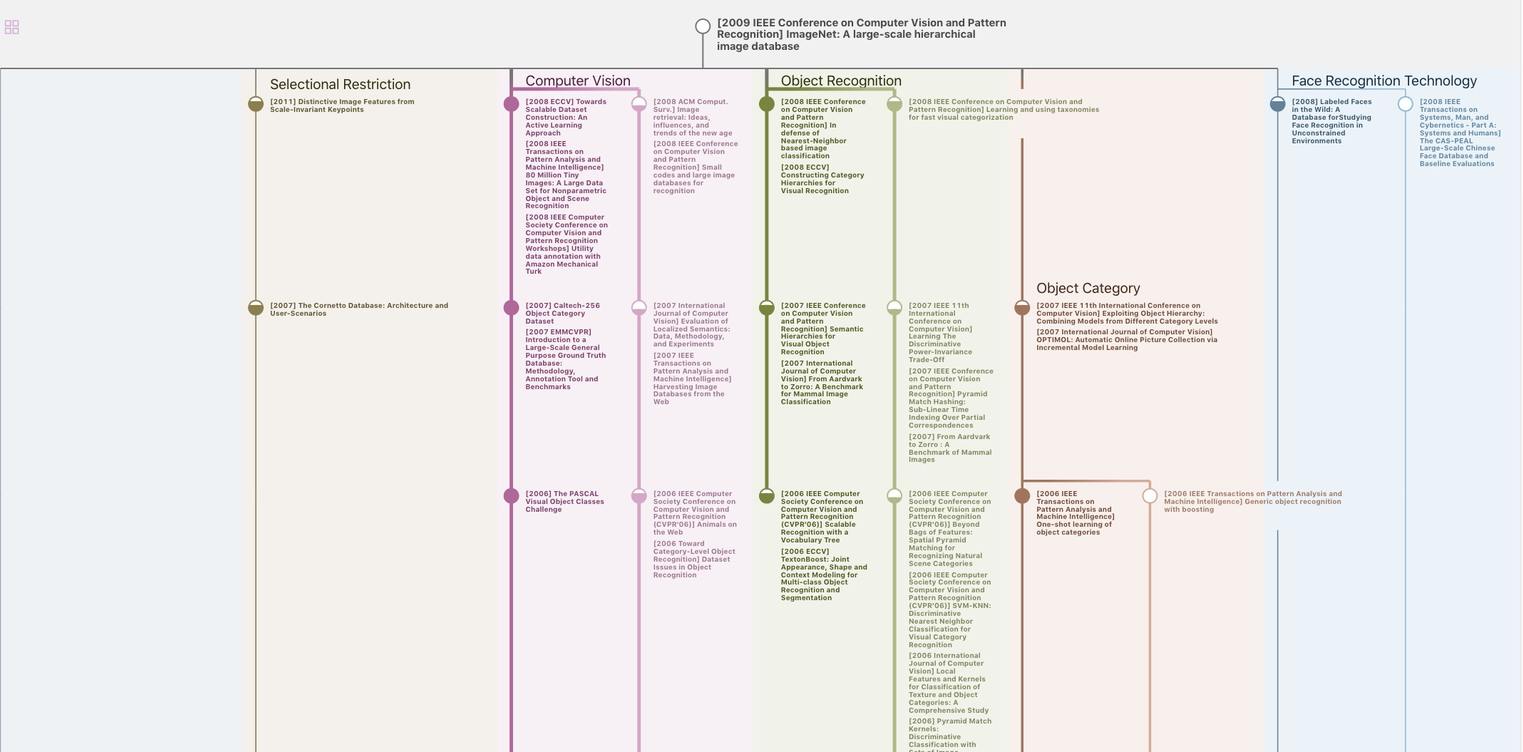
生成溯源树,研究论文发展脉络
Chat Paper
正在生成论文摘要