Multi-tasking atrous convolutional neural network for machinery fault identification
INTERNATIONAL JOURNAL OF ADVANCED MANUFACTURING TECHNOLOGY(2022)
摘要
As fault identification algorithms for rotating machinery based on deep learning are developing rapidly, convolutional neural networks (CNNs) have been attracting extensive attention due to their feature extraction capabilities. However, most of the current CNN–based fault identification models can only evaluate one aspect of the fault, and the recognition accuracy is low in the case of a complex fault. To achieve various and complex fault diagnoses, this paper proposes a multi-tasking atrous convolution neural network (MACNN). First, the network introduces sub-modules such as atrous convolutional layer, batch normalization processing, and PReLU activation function to improve the efficiency of down-sampling and better realize fault feature extraction. Secondly, a parallel multi-independent output layer and a special loss function corresponding to the multitasking structure are proposed, which make the network better in solving the problem of multi-dimensional fault assessment. Finally, based on the MACNN, experiments on rotating machinery fault diagnosis are carried out. In the experiment, three kinds of bearing fault positions and four kinds of depths are identified together, and the accuracy can reach more than 99%, which has obvious advantages over other neural network methods such as artificial neural network (ANN), traditional three-layer convolutional neural network (3L-CNN), and ACNN without multi-task learning.
更多查看译文
关键词
Machinery fault diagnosis,Multi-task learning,Atrous convolutional neural network
AI 理解论文
溯源树
样例
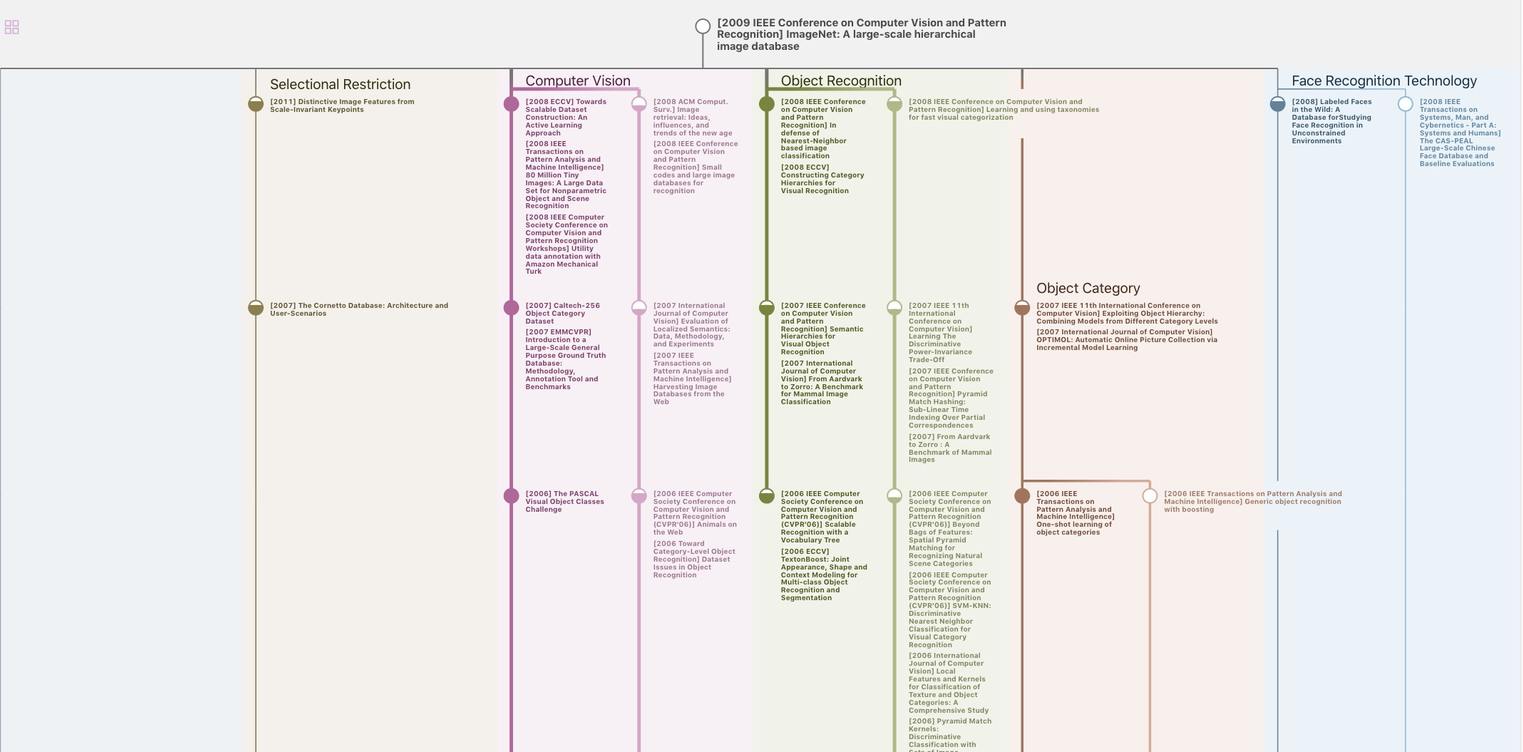
生成溯源树,研究论文发展脉络
Chat Paper
正在生成论文摘要