Walking to Hide: Privacy Amplification via Random Message Exchanges in Network
arxiv(2022)
摘要
The *shuffle model* is a powerful tool to amplify the privacy guarantees of the *local model* of differential privacy. In contrast to the fully decentralized manner of guaranteeing privacy in the local model, the shuffle model requires a central, trusted shuffler. To avoid this central shuffler, recent work of Liew et al. (2022) proposes shuffling locally randomized data in a decentralized manner, via random walks on the communication network constituted by the clients. The privacy amplification bound it thus provides depends on the topology of the underlying communication network, even for infinitely long random walks. It does not match the state-of-the-art privacy amplification bound for the shuffle model (Feldman et al., 2021). In this work, we prove that the output of~$n$ clients' data, each perturbed by an ${\epsilon}_0$-local randomizer, and shuffled by random walks with a logarithmic number of steps, is $( {O} ( (1 - e^{-\epsilon_0} ) \sqrt{ ( e^{\epsilon_0} / n ) \ln (1 / \delta ) } ), O(\delta) )$-differentially private. Importantly, this bound is independent of the topology of the communication network, and asymptotically closes the gap between the privacy amplification bounds for the network shuffle model (Liew et al., 2022) and the shuffle model (Feldman et al., 2021). Our proof is based on a reduction to the shuffle model, and an analysis of the distribution of random walks of finite length. Building on this, we further show that if each client is sampled independently with probability~$p$, the privacy guarantee of the network shuffle model can be further improved to $( {O} ( (1 - e^{-\epsilon_0} ) \sqrt{p ( e^{\epsilon_0} / n ) \ln (1 / \delta ) } ) , O(\delta) )$. Importantly, the subsampling is also performed in a fully decentralized manner that does not require a trusted central entity; compared with related bounds in prior work, our bound is stronger.
更多查看译文
关键词
privacy amplification,random message exchanges,network
AI 理解论文
溯源树
样例
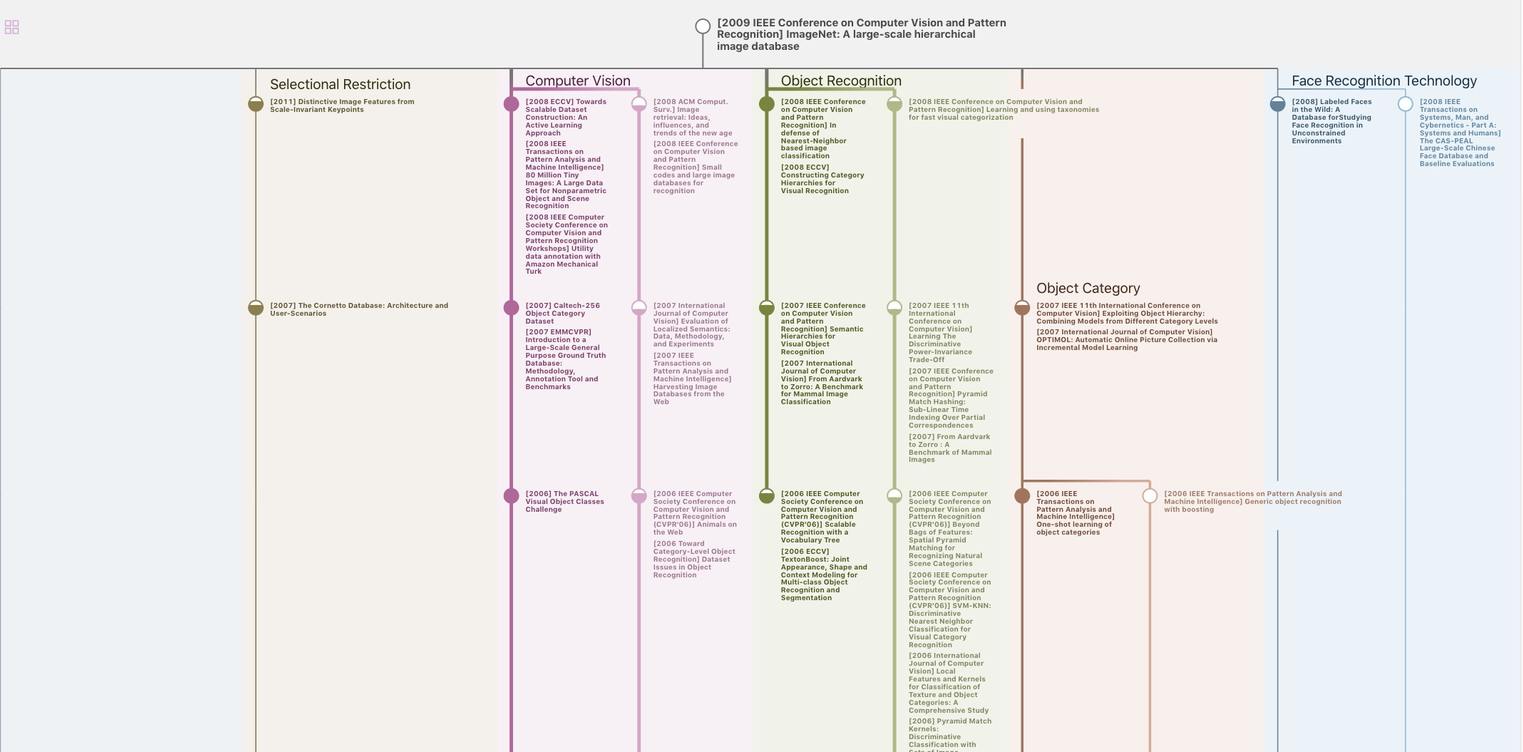
生成溯源树,研究论文发展脉络
Chat Paper
正在生成论文摘要