Classifier Ensemble Based on Multiview Optimization for High-Dimensional Imbalanced Data Classification
IEEE TRANSACTIONS ON NEURAL NETWORKS AND LEARNING SYSTEMS(2024)
摘要
High-dimensional class imbalanced data have plagued the performance of classification algorithms seriously. Because of a large number of redundant/invalid features and the class imbalanced issue, it is difficult to construct an optimal classifier for high-dimensional imbalanced data. Classifier ensemble has attracted intensive attention since it can achieve better performance than an individual classifier. In this work, we propose a multiview optimization (MVO) to learn more effective and robust features from high-dimensional imbalanced data, based on which an accurate and robust ensemble system is designed. Specifically, an optimized subview generation (OSG) in MVO is first proposed to generate multiple optimized subviews from different scenarios, which can strengthen the classification ability of features and increase the diversity of ensemble members simultaneously. Second, a new evaluation criterion that considers the distribution of data in each optimized subview is developed based on which a selective ensemble of optimized subviews (SEOS) is designed to perform the subview selective ensemble. Finally, an oversampling approach is executed on the optimized view to obtain a new class rebalanced subset for the classifier. Experimental results on 25 high-dimensional class imbalanced datasets indicate that the proposed method outperforms other mainstream classifier ensemble methods.
更多查看译文
关键词
Feature extraction,Optimization,Costs,Learning systems,Diversity reception,Data mining,Convolutional neural networks,Class imbalanced data,classification,ensemble learning,high-dimensional data,subview optimization
AI 理解论文
溯源树
样例
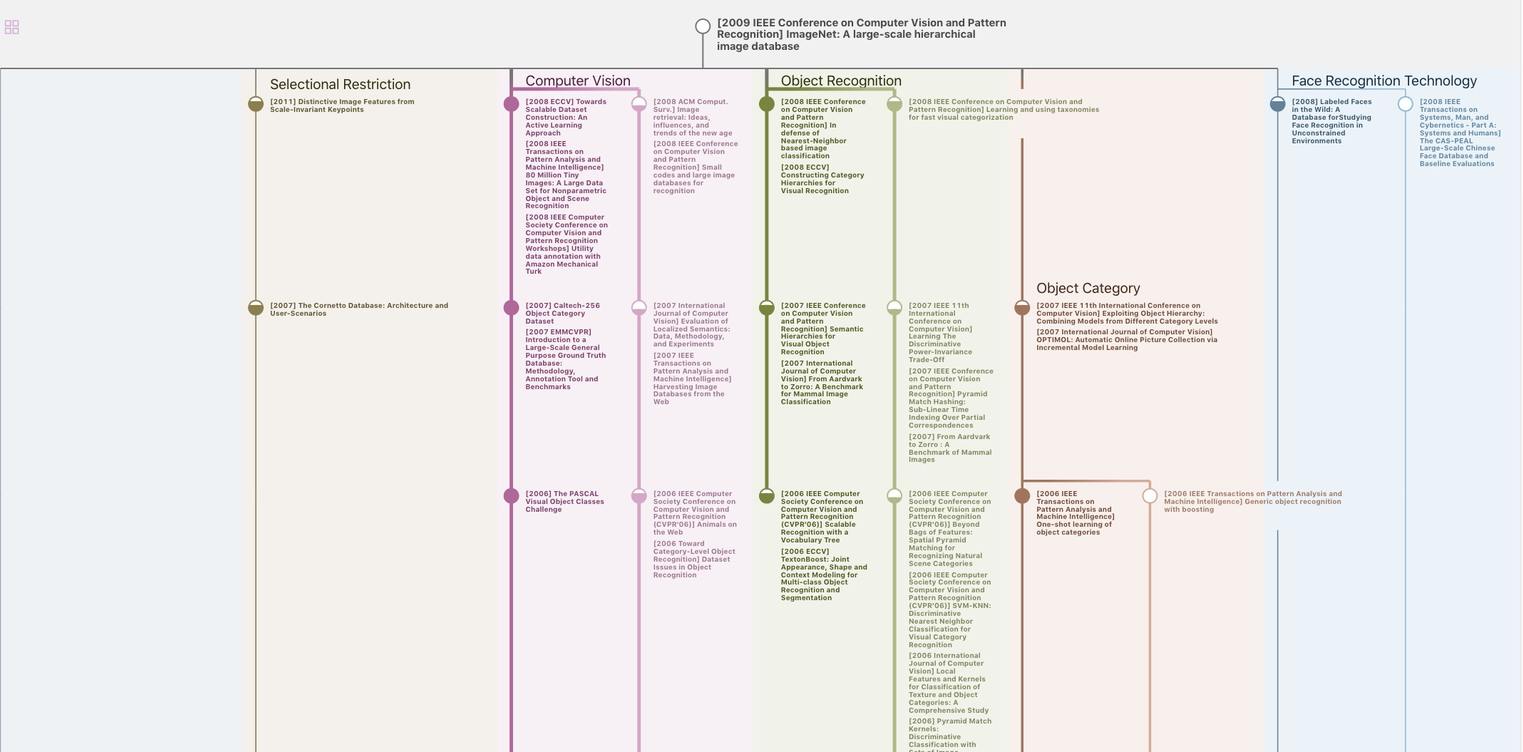
生成溯源树,研究论文发展脉络
Chat Paper
正在生成论文摘要