Optimizing Test-Time Query Representations for Dense Retrieval
conf_acl(2022)
摘要
Recent developments of dense retrieval rely on quality representations of queries and contexts coming from pre-trained query and context encoders. In this paper, we introduce TouR (test-time optimization of query representations), which further optimizes instance-level query representations guided by signals from test-time retrieval results. We leverage a cross-encoder re-ranker to provide fine-grained pseudo labels over retrieval results and iteratively optimize query representations with the gradient descent method. Our theoretical analysis reveals that TouR can be viewed as a generalization of the classical Rocchio's algorithm for pseudo relevance feedback, and we present two variants leveraging psuedo labels as either hard binary or soft continuous labels. We first apply TouR on phrase retrieval with our proposed phrase re-ranker. On passage retrieval, we demonstrate its effectiveness with an off-the-shelf re-ranker. TouR improves the end-to-end open-domain QA accuracy significantly, as well as passage retrieval performance. Compared to re-ranker, TouR requires a smaller number of candidates, and achieves consistently better performance and runs up to 4x faster with our efficient implementation.
更多查看译文
关键词
dense retrieval,test-time
AI 理解论文
溯源树
样例
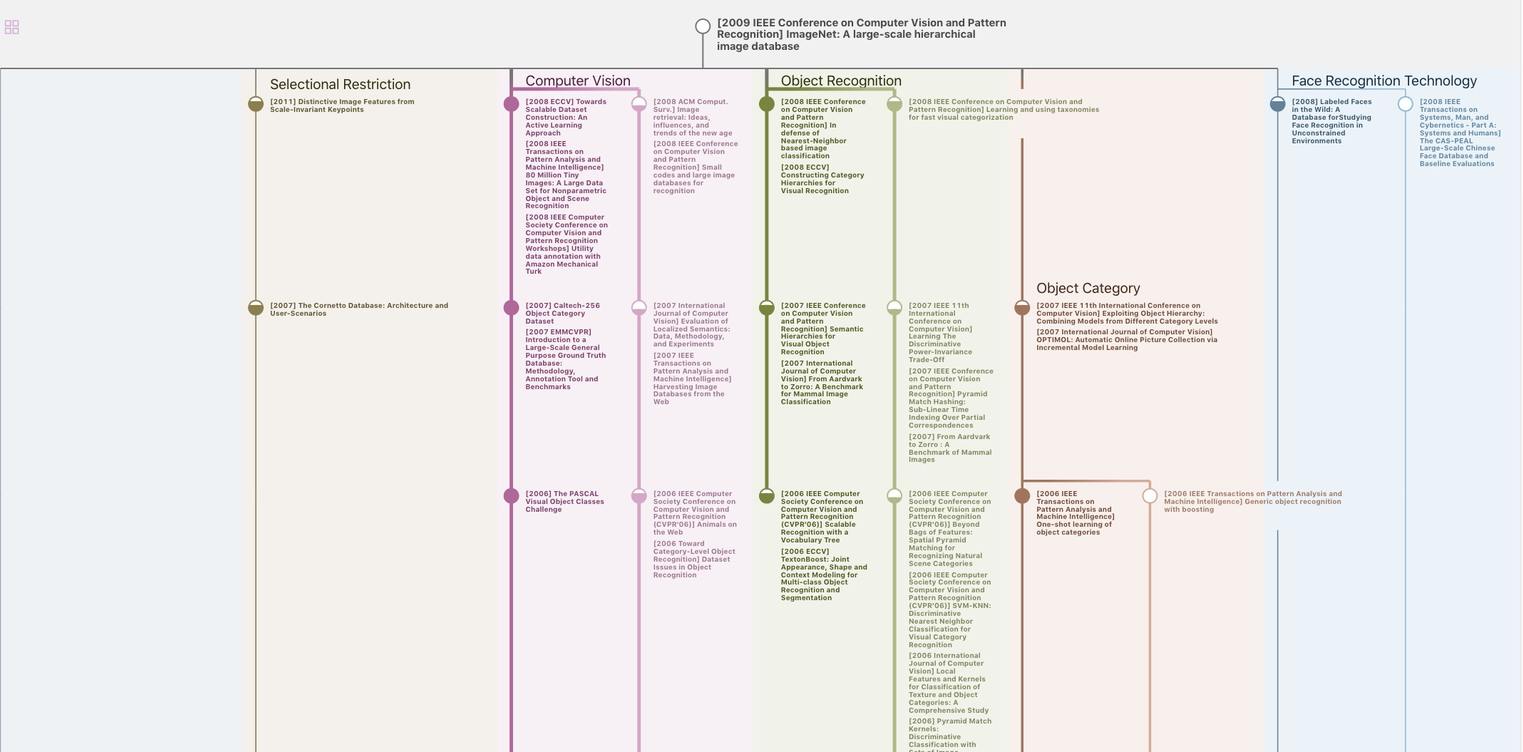
生成溯源树,研究论文发展脉络
Chat Paper
正在生成论文摘要