A convolutional neural network for pipe crack and leak detection in smart water network
STRUCTURAL HEALTH MONITORING-AN INTERNATIONAL JOURNAL(2023)
摘要
The implementation of a smart water network (SWN) is viewed as a strategic approach to address many challenges faced by water utilities, such as pipe leak detection and main break prevention. This paper develops a convolutional neural network (CNN)-based model to classify acoustic wave files collected by the South Australian Water Corporation's (SA Water's) SWN over the city of Adelaide. The VGGish model (VGG refers to the team who developed the model-Visual Geometry Group) is selected as a suitable transfer learning model to extract features from wave files. The CNN model classifies an acoustic wave file as an anomaly or other background or environmental noise. Identification of a wave file as an anomaly triggers a Siamese CNN model to determine whether it is related to a regular/irregular scheduled event (for example, irrigation system near public parks or water consumption by large buildings). A field investigation is initiated if a wave file is classified as an anomaly and it is not related to a scheduled event. The developed models have been validated using data that is recorded by SWN in Adelaide. This validation data set comprises 1098 wave files, which are recorded by 34 accelerometers and are associated with 32 known leaks. The validation results shown that accuracy of alarms generated by the developed models is 92.44%. The validations confirm the developed models as an effective tool for water pipeline leak and crack detection, which, in turn, enables proactive management of the pipeline assets.
更多查看译文
关键词
Smart Water Network, Leak detection, Crack detection, Convolutional Neural Network, Accelerometers, Acoustic Signal
AI 理解论文
溯源树
样例
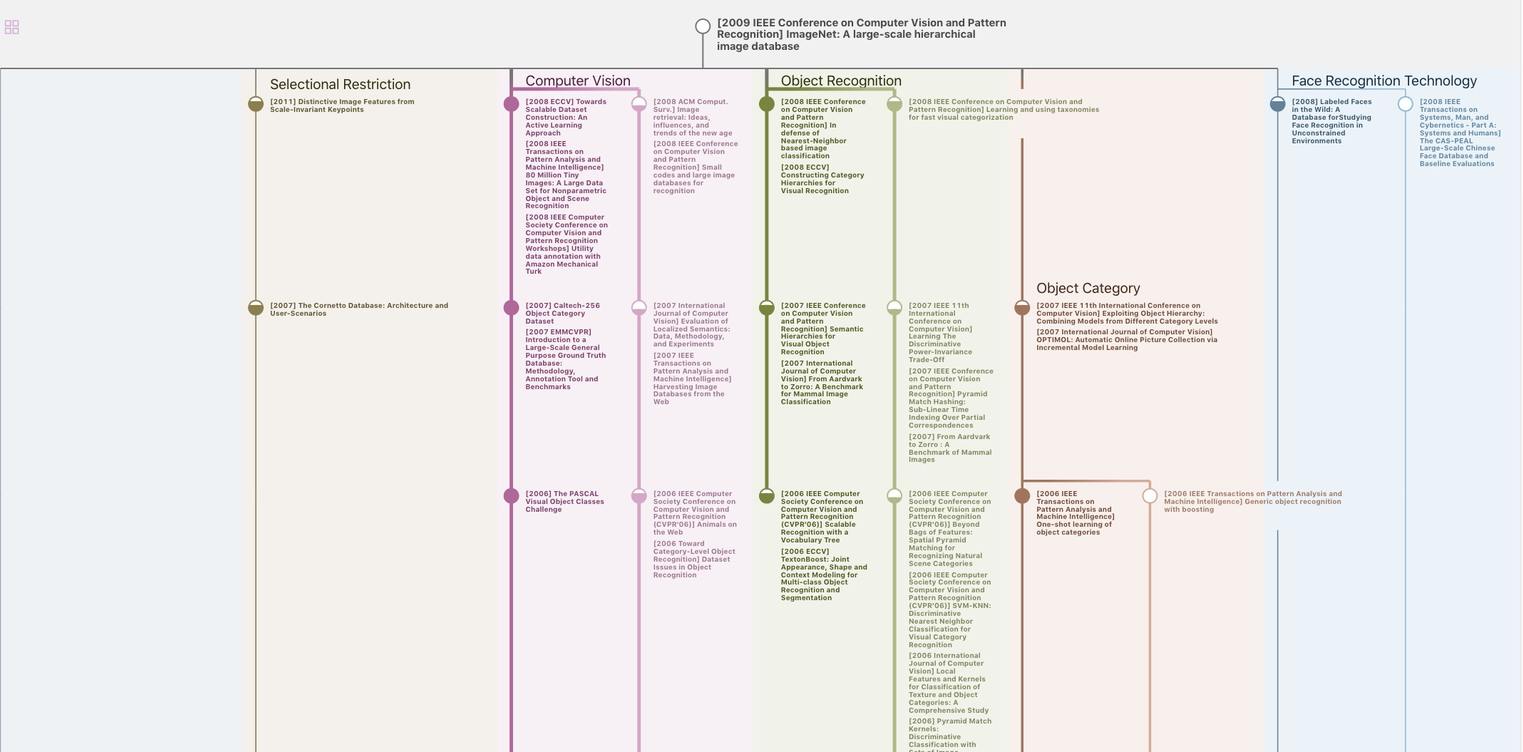
生成溯源树,研究论文发展脉络
Chat Paper
正在生成论文摘要