Multi-hypothesis Inspired Super-resolution for Compression Distorted Screen Content Image
APPLICATIONS OF DIGITAL IMAGE PROCESSING XLIV(2021)
摘要
Multi-hypothesis-based prediction has been repetitively proven to be effective in improving prediction accuracy and enhancing coding performance. In this paper, we introduce the principle of multi-hypothesis to the super-resolution (SR) of compressed screen content images, with the goal of improving the restoration quality of the compression contaminated screen content images. More specifically, the super-resolution is achieved by a deep neural network. The deep neural network learns the mapping relationship between the compressed low-resolution (LR) image and the original high-resolution (HR) image. During learning process, we feed multiple LR patches for training, including the current patch and five neighboring patches, providing more informative clues for the learning of the high-quality restoration. In the inference process, input LR image will be translated with random offsets, yielding five assistant LR items for the SR of the input LR image. The LR and assistant LR items employ separate modules for feature extraction and then the features are fused with concatenation. Subsequently, the deep residual feature extraction is applied, which is composed of multiple consecutive residual blocks. Finally, the deep features are reconstructed with pixel shuffle, producing the SR image. Experimental results verify the effectiveness of the proposed multi-hypothesis-based SR scheme.
更多查看译文
关键词
Screen content, super resolution, multihypothesis, VVC
AI 理解论文
溯源树
样例
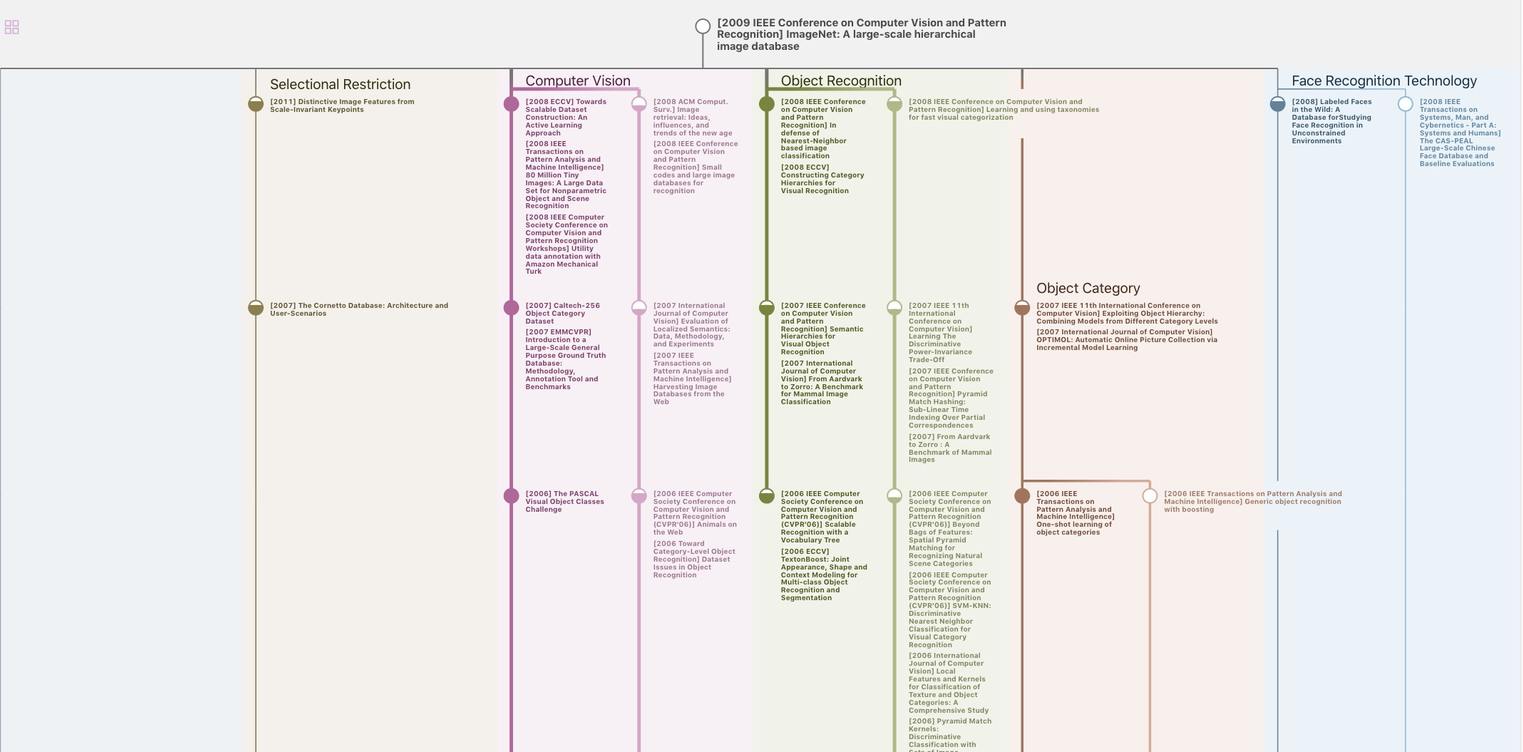
生成溯源树,研究论文发展脉络
Chat Paper
正在生成论文摘要