Quantitative Comparison of Point Cloud Compression Algorithms With PCC Arena
IEEE Transactions on Multimedia(2023)
摘要
With the growth of Extended Reality (XR) and capturing devices, point cloud representation has become attractive to academics and industry. Point Cloud Compression (PCC) algorithms further promote numerous XR applications that may change our daily life. However, in the literature, PCC algorithms are often evaluated with heterogeneous datasets, metrics, and parameters, making the results hard to interpret. In this article, we propose an open-source benchmark platform called PCC Arena. Our platform is modularized in three aspects: PCC algorithms, point cloud datasets, and performance metrics. Users can easily extend PCC Arena in each aspect to fulfill the requirements of their experiments. To show the effectiveness of PCC Arena, we integrate seven PCC algorithms into PCC Arena along with six point cloud datasets. We then compare the algorithms on ten carefully selected metrics to evaluate the quality of the output point clouds. We further conduct a user study to quantify the user-perceived quality of rendered images that are produced by different PCC algorithms. Several novel insights are revealed in our comparison: (i) Signal Processing (SP)-based PCC algorithms are stable for different usage scenarios, but the trade-offs between coding efficiency and quality should be carefully addressed, (ii) Neural Network (NN)-based PCC algorithms have the potential to consume lower bitrates yet provide similar results to SP-based algorithms, (iii) NN-based PCC algorithms may generate artifacts and suffer from long running time, and (iv) NN-based PCC algorithms are worth more in-depth studies as the recently proposed NN-based PCC algorithms improve the quality and running time. We believe that PCC Arena can play an essential role in allowing engineers and researchers to better interpret and compare the performance of future PCC algorithms.
更多查看译文
关键词
Benchmark, compression, quality assessment, subject metrics, objective metrics, Quality-of-Experience, Quality-of-Service
AI 理解论文
溯源树
样例
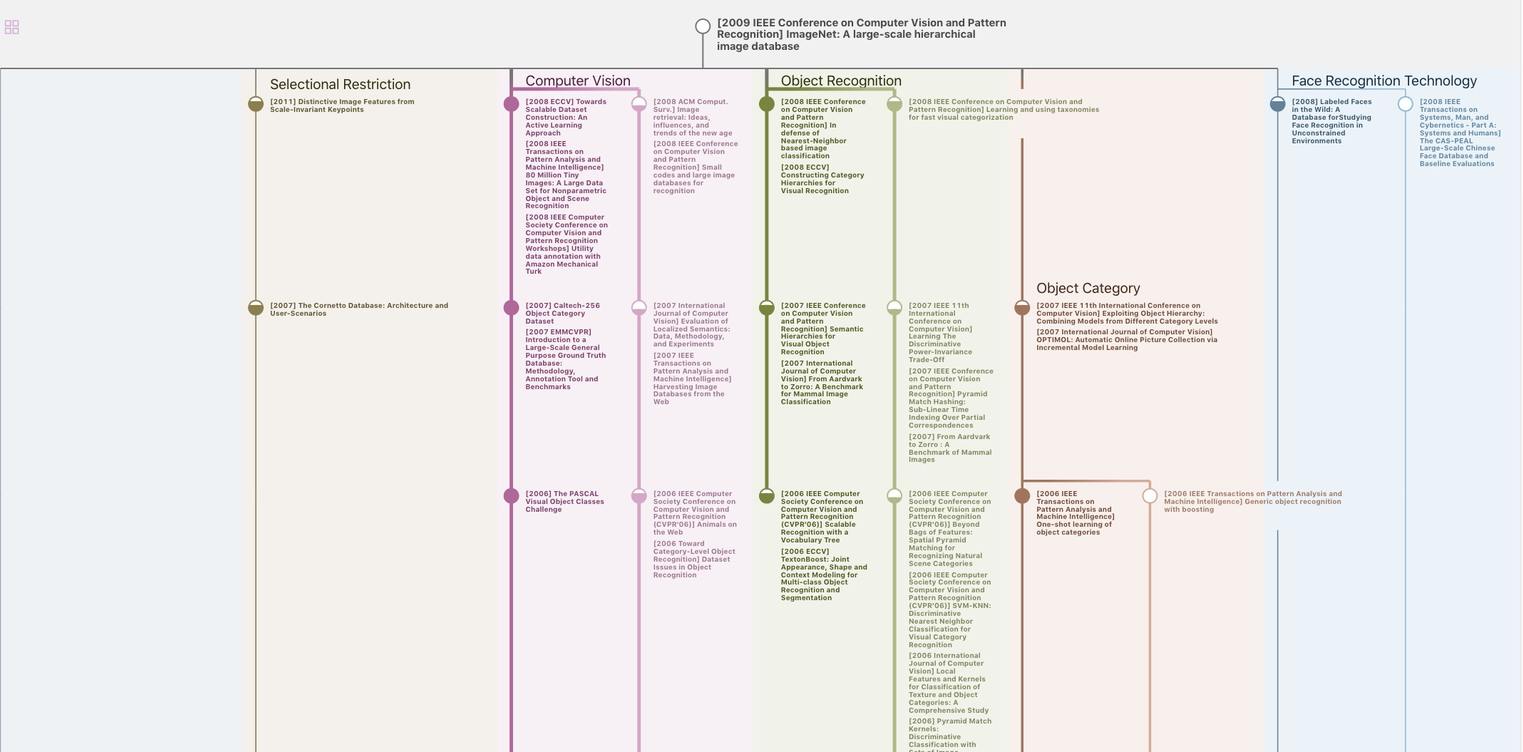
生成溯源树,研究论文发展脉络
Chat Paper
正在生成论文摘要