Optimizing Fact-Learning with a Response-Latency-Based Adaptive System
semanticscholar(2022)
摘要
Over a century of scientific research into learning and forgetting has revealed a number of laws describing human memory processes which are the principal constituents of mathematical models that capture forgetting processes and how they unfold over time. Recently, these models have been applied in computerized learning systems that adapt to individual learners. This is typically done by having the model predict the accuracy of a response to an item, and then updating the model based on the observed behavior to improve the fidelity of predictions on subsequent repetitions. Using accuracy works well in extensive learning sessions that provide enough time for students to make sufficient mistakes to discriminate easy from difficult items (and/or good from poor learners). However, real-life learning sessions tend to be relatively short, and requiring errors to update the model is therefore suboptimal. Here we show that a memory model-based system can be calibrated to a learner’s proficiency in relatively short learning sessions by taking accuracy and response latency into account. We conducted two experiments, testing the system in a real-life secondary education classroom setting as well as in the laboratory with university students, with and without prior knowledge of the material, respectively. We find an advantage of model-based systems over simple control conditions and highlight the benefit of using response latencies along with accuracy.
更多查看译文
AI 理解论文
溯源树
样例
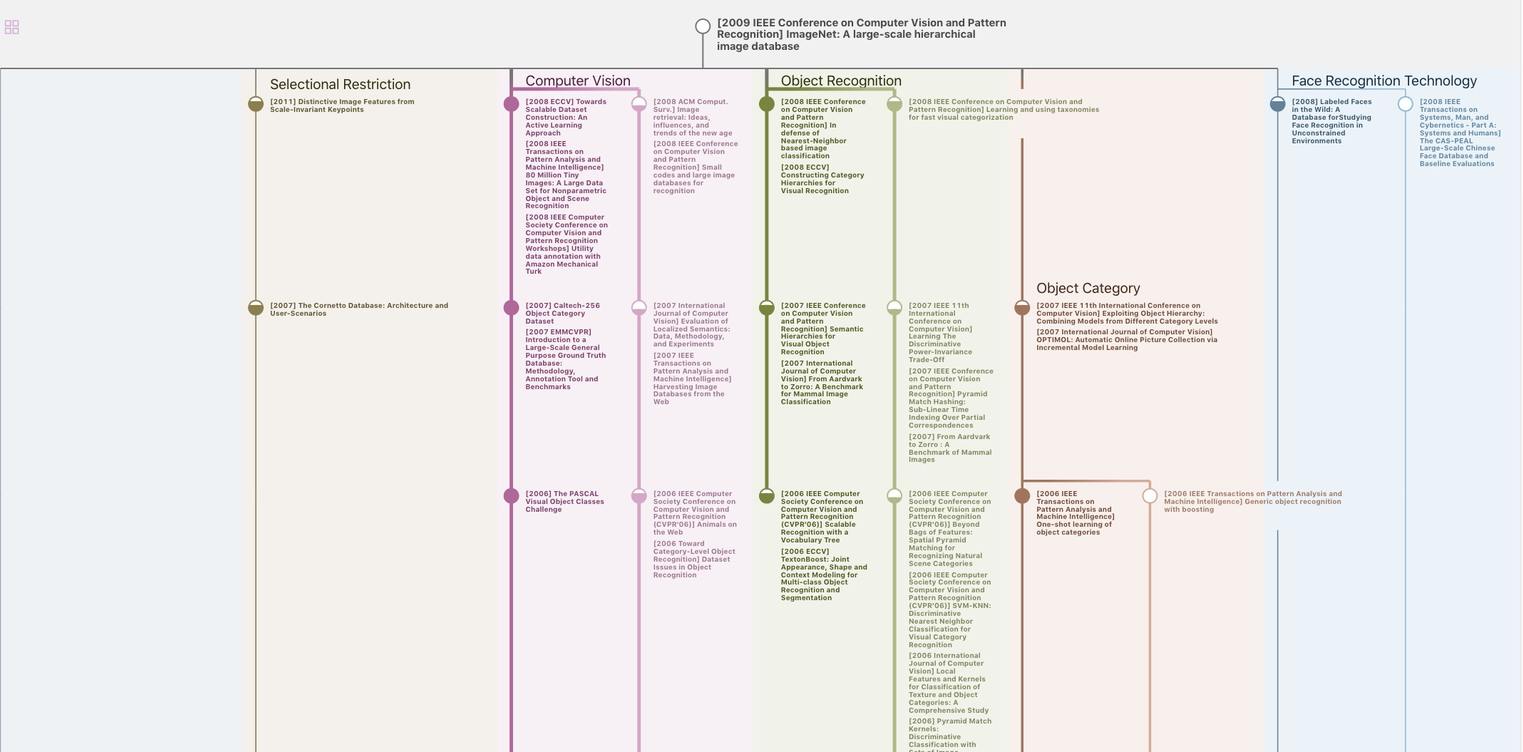
生成溯源树,研究论文发展脉络
Chat Paper
正在生成论文摘要