BINAS: Bilinear Interpretable Neural Architecture Search
ACML(2021)
摘要
. Making neural networks practical often requires adhering to resource constraints such as latency, energy and memory. To solve this we introduce a Bilinear Interpretable approach for constrained Neural Architecture Search (BINAS) that is based on an accurate and simple bilinear formulation of both an accuracy estimator and the expected resource requirement, jointly with a scalable search method with theoretical guarantees. One major advantage of BINAS is providing interpretability via insights about the contribution of different design choices. For example, we find that in the examined search space, adding depth and width is more effective at deeper stages of the network and at the beginning of each resolution stage. BINAS differs from previous methods that typically use complicated accuracy predictors that make them hard to interpret, sensitive to many hyper-parameters, and thus with compromised final accuracy. Our experiments 3 show that BINAS generates comparable to or better than state of the art architectures, while reducing the marginal search cost, as well as strictly satisfying the resource constraints.
更多查看译文
关键词
architecture,search
AI 理解论文
溯源树
样例
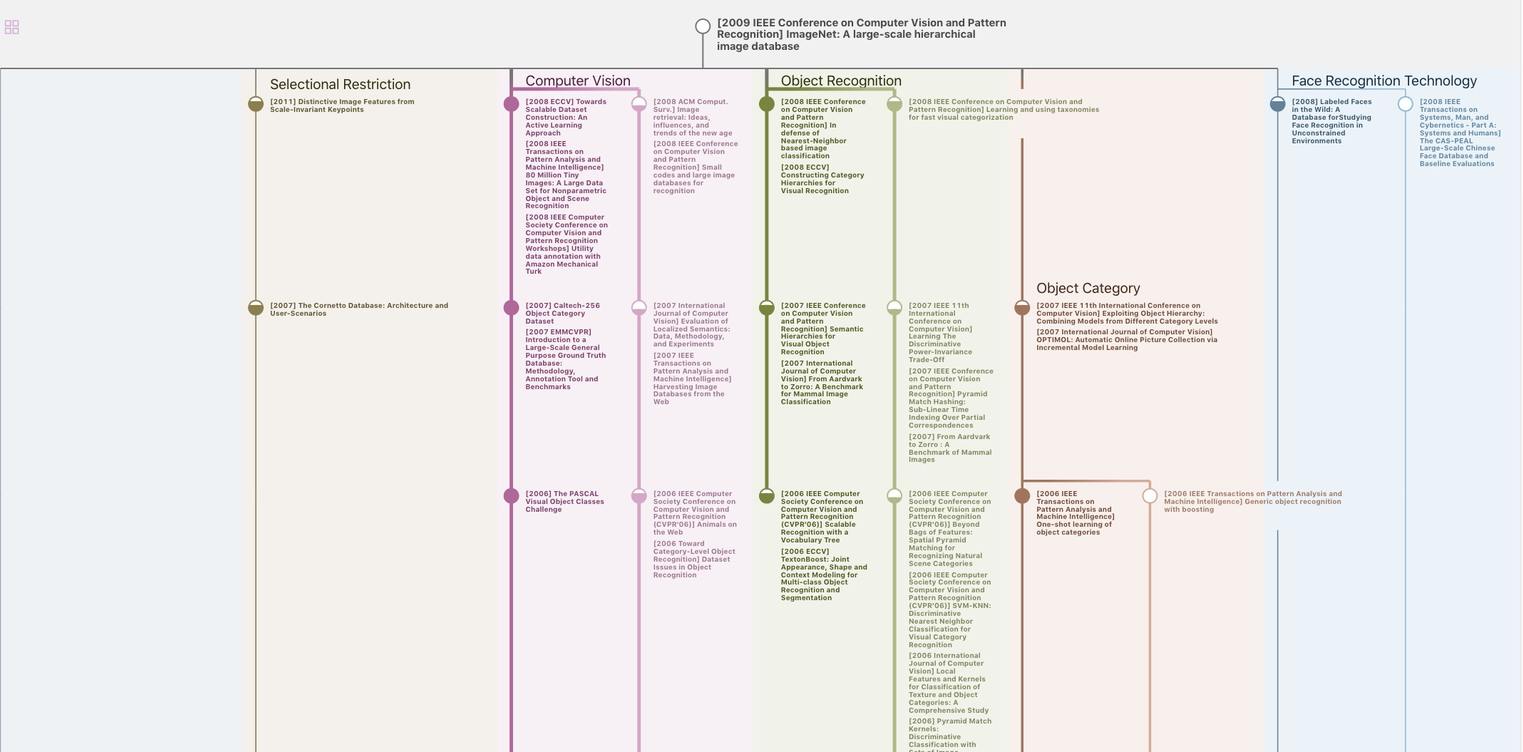
生成溯源树,研究论文发展脉络
Chat Paper
正在生成论文摘要